Advanced Skills through Multiple Adversarial Motion Priors in Reinforcement Learning
2023 IEEE INTERNATIONAL CONFERENCE ON ROBOTICS AND AUTOMATION, ICRA(2023)
摘要
Reinforcement learning (RL) has emerged as a powerful approach for locomotion control of highly articulated robotic systems. However, one major challenge is the tedious process of tuning the reward function to achieve the desired motion style. To address this issue, imitation learning approaches such as adversarial motion priors have been proposed, which encourage a pre-defined motion style. In this work, we present an approach to enhance the concept of adversarial motion prior-based RL, allowing for multiple, discretely switchable motion styles. Our approach demonstrates that multiple styles and skills can be learned simultaneously without significant performance differences, even in combination with motion data-free skills. We conducted several real-world experiments using a wheeled-legged robot to validate our approach. The experiments involved learning skills from existing RL controllers and trajectory optimization, such as ducking and walking, as well as novel skills, such as switching between a quadrupedal and humanoid configuration. For the latter skill, the robot was required to stand up, navigate on two wheels, and sit down. Instead of manually tuning the sit-down motion, we found that a reverse playback of the stand-up movement helped the robot discover feasible sit-down behaviors and avoided the need for tedious reward function tuning.
更多查看译文
AI 理解论文
溯源树
样例
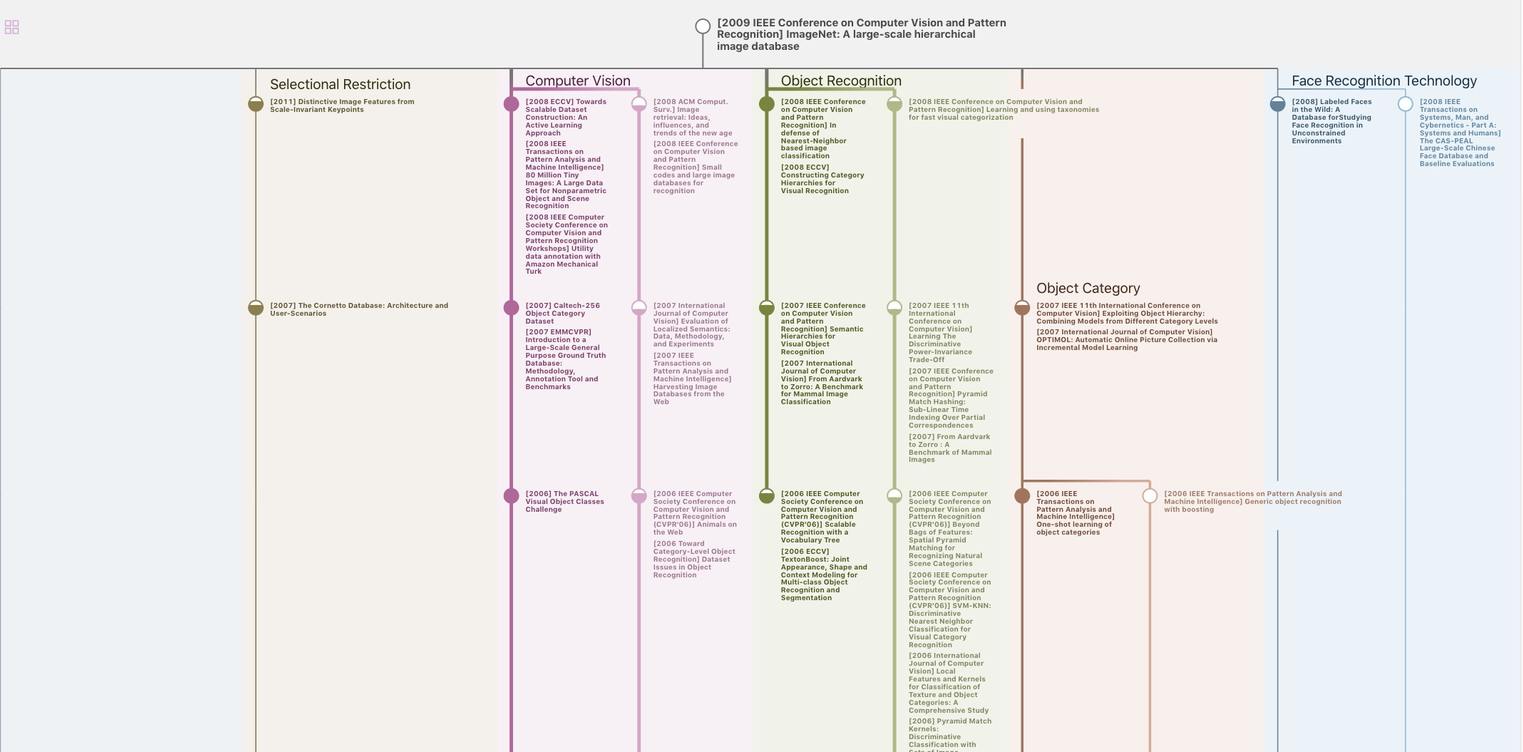
生成溯源树,研究论文发展脉络
Chat Paper
正在生成论文摘要