MFA-Conformer: Multi-scale Feature Aggregation Conformer for Automatic Speaker Verification.
Conference of the International Speech Communication Association (INTERSPEECH)(2022)
摘要
In this paper, we present Multi-scale Feature Aggregation Conformer (MFA-Conformer), an easy-to-implement, simple but effective backbone for automatic speaker verification based on the Convolution-augmented Transformer (Conformer). The architecture of the MFA-Conformer is inspired by recent state-of-the-art models in speech recognition and speaker verification. Firstly, we introduce a convolution sub-sampling layer to decrease the computational cost of the model. Secondly, we adopt Conformer blocks which combine Transformers and convolution neural networks (CNNs) to capture global and local features effectively. Finally, the output feature maps from all Conformer blocks are concatenated to aggregate multi-scale representations before final pooling. We evaluate the MFA-Conformer on the widely used benchmarks. The best system obtains 0.64%, 1.29% and 1.63% EER on VoxCeleb1-O, SITW.Dev, and SITW.Eval set, respectively. MFA-Conformer significantly outperforms the popular ECAPA-TDNN systems in both recognition performance and inference speed. Last but not the least, the ablation studies clearly demonstrate that the combination of global and local feature learning can lead to robust and accurate speaker embedding extraction. We will release the code for future works to do comparison.
更多查看译文
关键词
speaker verification, Transformer, Conformer, speaker recognition
AI 理解论文
溯源树
样例
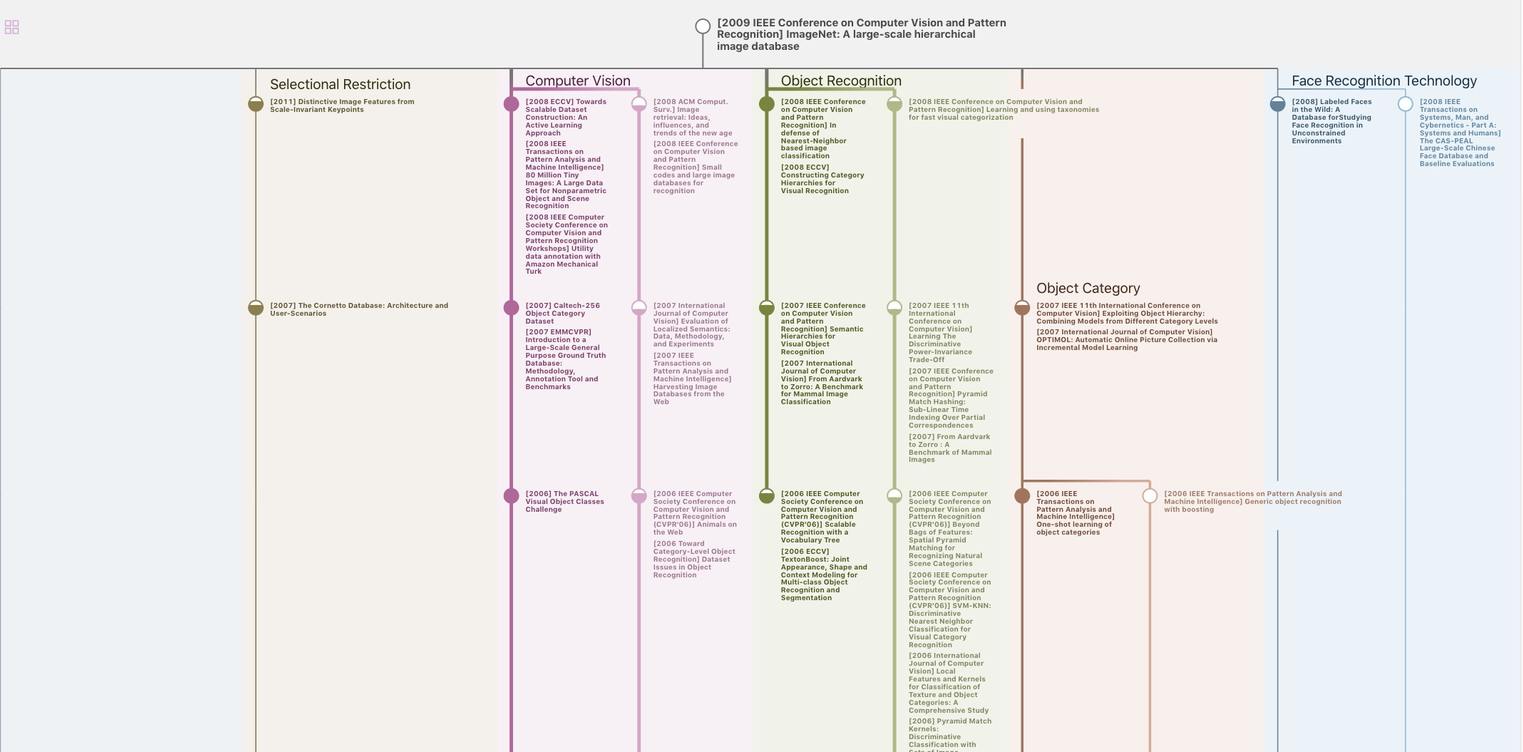
生成溯源树,研究论文发展脉络
Chat Paper
正在生成论文摘要