What to expect from dynamical modelling of cluster haloes - II. Investigating dynamical state indicators with Random Forest
MONTHLY NOTICES OF THE ROYAL ASTRONOMICAL SOCIETY(2022)
摘要
We investigate the importance of various dynamical features in predicting the dynamical state (ds) of galaxy clusters, based on the Random Forest (RF) machine-learning approach. We use a large sample of galaxy clusters from the Three Hundred Project of hydrodynamical zoomed-in simulations, and construct dynamical features from the raw data as well as from the corresponding mock maps in the optical, X-ray, and Sunyaev-Zel'dovich (SZ) channels. Instead of relying on the impurity based feature importance of the RF algorithm, we directly use the out-of-bag (oob) scores to evaluate the importance of individual features and different feature combinations. Among all the features studied, we find the virial ratio, eta, to be the most important single feature. The features calculated directly from the simulations and in three dimensions carry more information on the ds than those constructed from the mock maps. Compared with the features based on X-ray or SZ maps, features related to the centroid positions are more important. Despite the large number of investigated features, a combination of up to three features of different types can already saturate the score of the prediction. Lastly, we show that the most sensitive feature eta is strongly correlated with the well-known half-mass bias in dynamical modelling. Without a selection in ds, cluster haloes have an asymmetric distribution in eta, corresponding to an overall positive half-mass bias. Our work provides a quantitative reference for selecting the best features to discriminate the ds of galaxy clusters in both simulations and observations.
更多查看译文
关键词
methods: statistical, galaxies: clusters: general, galaxies: haloes, galaxies: kinematics and dynamics
AI 理解论文
溯源树
样例
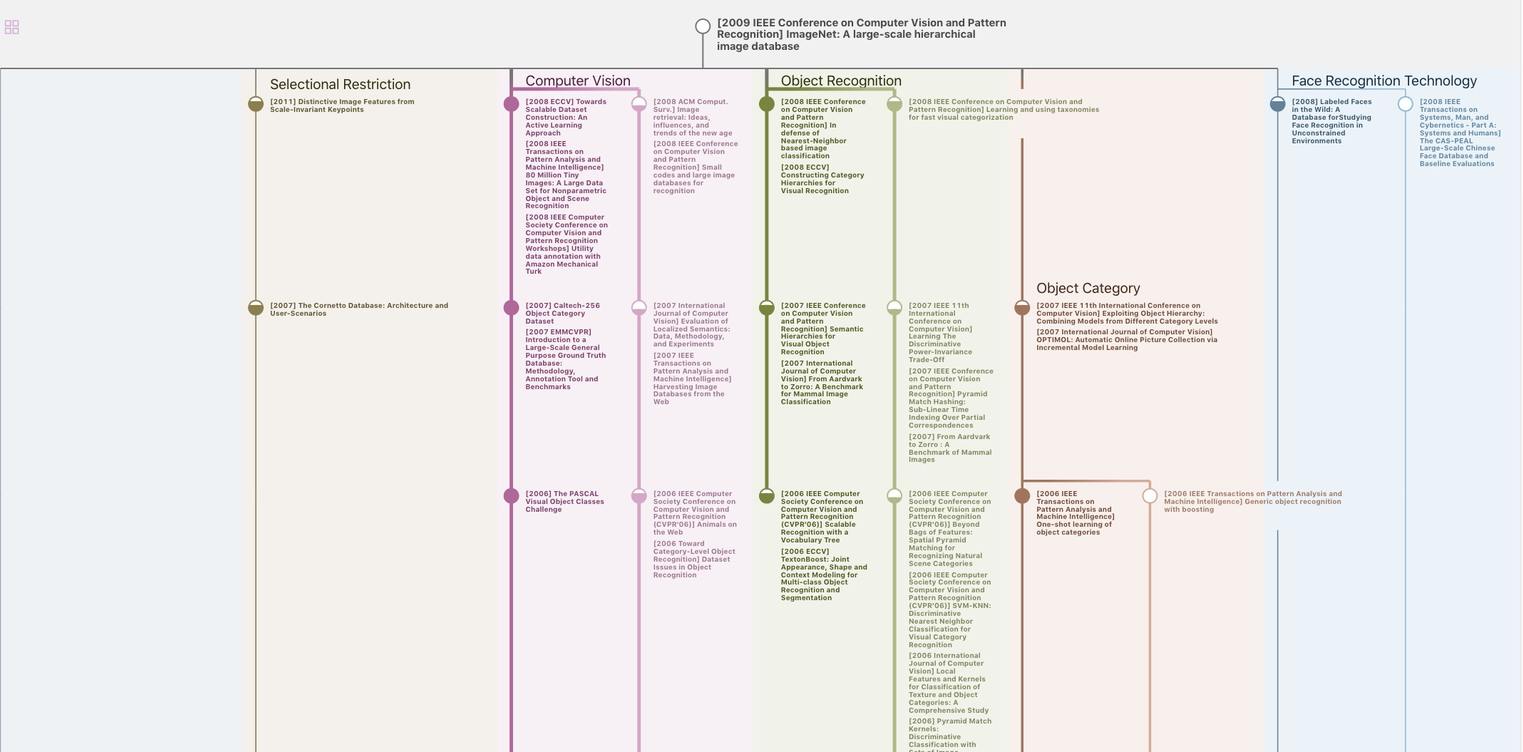
生成溯源树,研究论文发展脉络
Chat Paper
正在生成论文摘要