Learning-Based Point Cloud Registration for 6D Object Pose Estimation in the Real World.
European Conference on Computer Vision(2022)
摘要
In this work, we tackle the task of estimating the 6D pose of an object from point cloud data. While recent learning-based approaches to addressing this task have shown great success on synthetic datasets, we have observed them to fail in the presence of real-world data. We thus analyze the causes of these failures, which we trace back to the difference between the feature distributions of the source and target point clouds, and the sensitivity of the widely-used SVD-based loss function to the range of rotation between the two point clouds. We address the first challenge by introducing a new normalization strategy, Match Normalization, and the second via the use of a loss function based on the negative log likelihood of point correspondences. Our two contributions are general and can be applied to many existing learning-based 3D object registration frameworks, which we illustrate by implementing them in two of them, DCP and IDAM. Our experiments on the real-scene TUD-L, LINEMOD and Occluded-LINEMOD datasets evidence the benefits of our strategies. They allow for the first time learning-based 3D object registration methods to achieve meaningful results on real-world data. We therefore expect them to be key to the future development of point cloud registration methods.
更多查看译文
关键词
6D object pose estimation,Point cloud registration
AI 理解论文
溯源树
样例
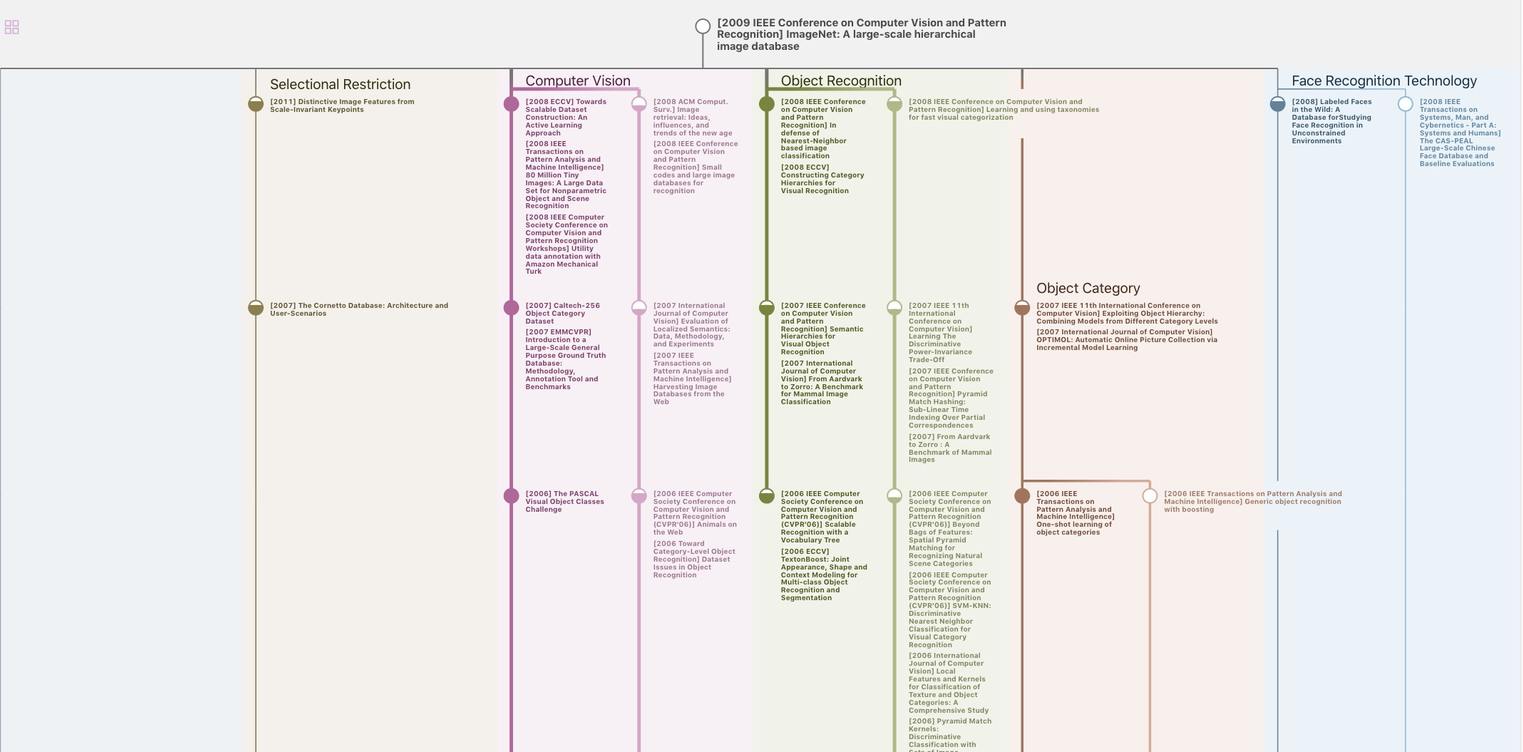
生成溯源树,研究论文发展脉络
Chat Paper
正在生成论文摘要