Hyperbolic Geometry-Based Deep Learning Methods to Produce Population Trees from Genotype Data
biorxiv(2022)
摘要
The production of population-level trees using the genomic data of individuals is a fundamental task in the field of population genetics. Typically, these trees are produced using standard methods like hierarchical clustering, neighbor joining, or maximum likelihood. However, such methods are non-parametric: they require all data to be present at the time of tree formation, and the addition of new data points necessitates the regeneration of the entire tree, a potentially expensive process. They also do not easily integrate with larger workflows. In this study, we aim to address these problems by introducing parametric deep learning methods for tree formation from genotype data. Our models specifically create continuous representations of population trees in hyperbolic space, which has previously proven highly effective in embedding hierarchically structured data. We present two different architectures - a multi-layer perceptron (MLP) and a variational autoencoder (VAE) - and we analyze their performance using a variety of metrics along with comparisons to established tree-building methods. Both models tested produce embedding spaces that reflect human evolutionary history. In addition, we demonstrate the generalizability of these models by verifying that addition of new samples to an existing tree occurs in a semantically meaningful manner. Finally, we use Dasgupta's Cost to compare the quality of trees generated by our models to those produced by established methods. Despite the fact that the benchmark methods are directly fit on the evaluation data, our models are able to outperform some of these and achieve highly comparable performance overall.
### Competing Interest Statement
The authors have declared no competing interest.
更多查看译文
关键词
population trees,deep learning methods,genotype,deep learning,geometry-based
AI 理解论文
溯源树
样例
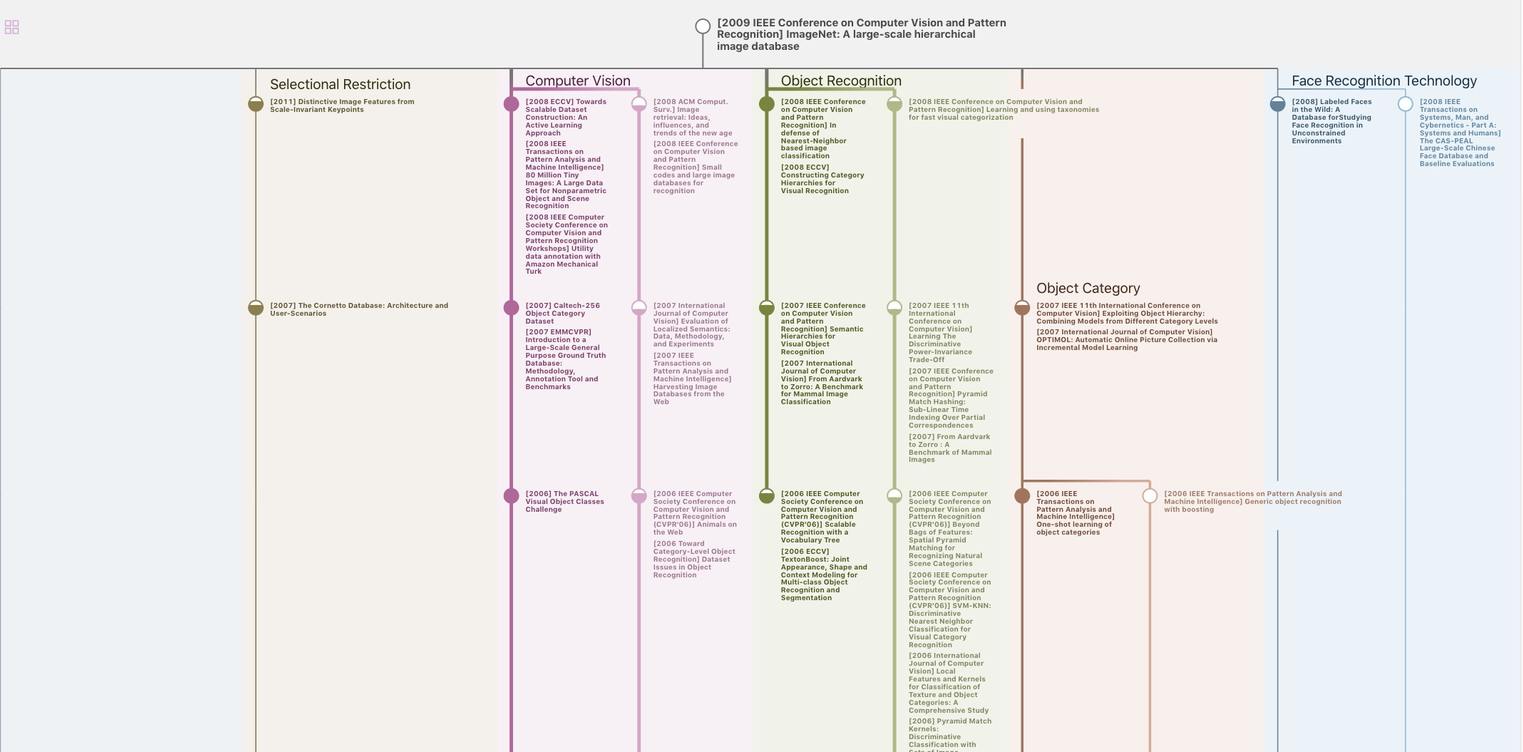
生成溯源树,研究论文发展脉络
Chat Paper
正在生成论文摘要