Mining of Single-Cell Signaling Time-Series for Dynamic Phenotypes with Clustering.
Methods in molecular biology (Clifton, N.J.)(2022)
摘要
Fluorescent live cell time-lapse microscopy is steadily contributing to our better understanding of the relationship between cell signaling and fate. However, large volumes of time-series data generated in these experiments and the heterogenous nature of signaling responses due to cell-cell variability hinder the exploration of such datasets. The population averages insufficiently describe the dynamics, yet finding prototypic dynamic patterns that relate to different cell fates is difficult when mining thousands of time-series. Here we demonstrate a protocol where we identify such dynamic phenotypes in a population of PC-12 cells that respond to a range of sustained growth factor perturbations. We use Time-Course Inspector, a free R/Shiny web application to explore and cluster single-cell time-series.
更多查看译文
关键词
Cell-cell heterogeneity,Clustering,Computational biology,Data analysis,Signal processing,Signaling dynamics,Single-cell data,Time-series
AI 理解论文
溯源树
样例
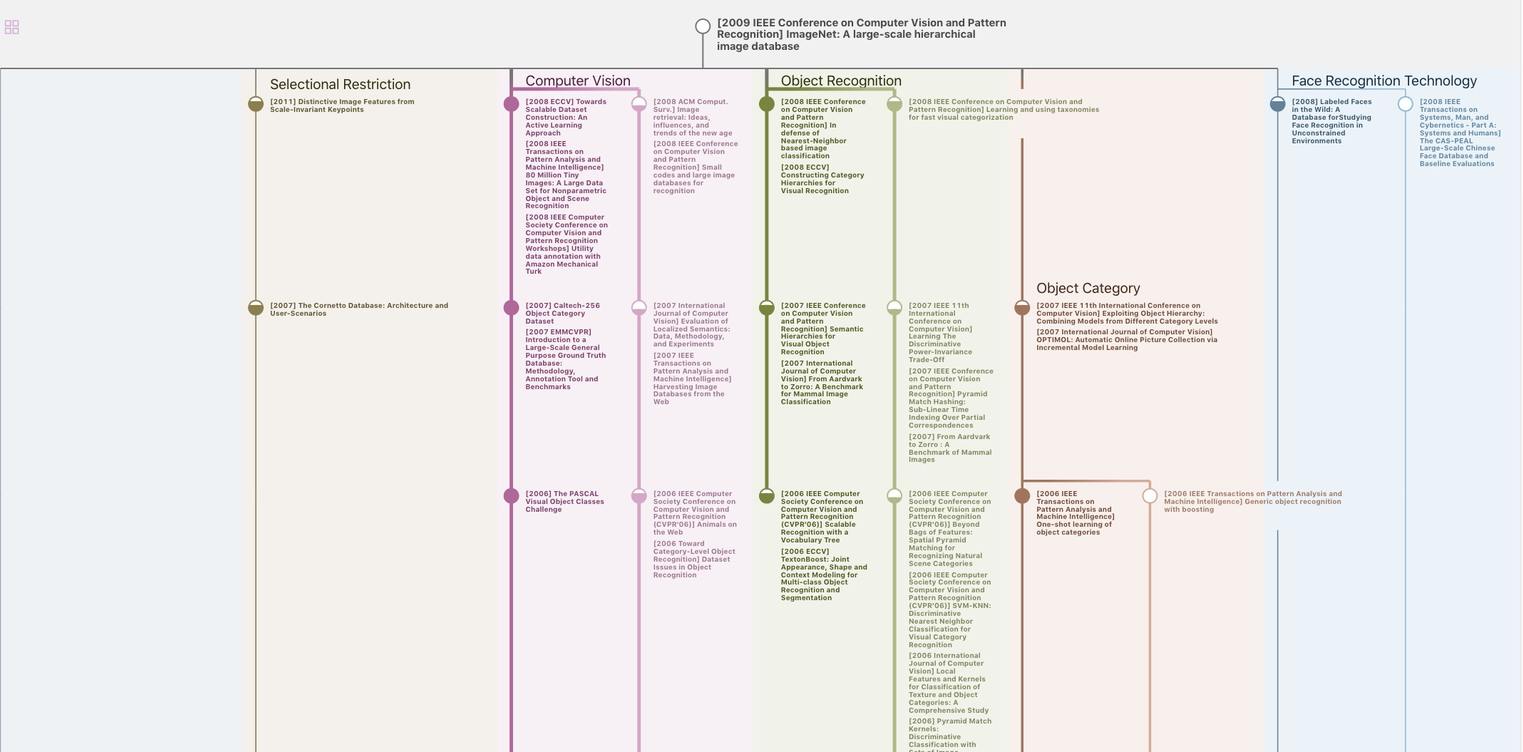
生成溯源树,研究论文发展脉络
Chat Paper
正在生成论文摘要