Accurate Deep Learning-Aided Density-Free Strategy for Many-Body Dispersion-Corrected Density Functional Theory
JOURNAL OF PHYSICAL CHEMISTRY LETTERS(2022)
Abstract
Using a deep neuronal network (DNN) model trained on the large ANI-1 data set of small organic molecules, we propose a transferable density-free many-body dispersion (DNN-MBD) model. The DNN strategy bypasses the explicit Hirshfeld partitioning of the Kohn-Sham electron density required by MBD models to obtain the atom-in-molecules volumes used by the Tkatchenko-Scheffler polarizability rescaling. The resulting DNN-MBD model is trained with minimal basis iterative Stockholder atomic volumes and, coupled to density functional theory ( DFT), exhibits comparable (if not greater) accuracy to other approaches based on different partitioning schemes. Implemented in the Tinker-HP package, the DNN-MBD model decreases the overall computational cost compared to MBD models where the explicit density partitioning is performed. Its coupling with the recently introduced Stochastic formulation of the MBD equations (J. Chem. Theory Comput. 2022, 18 (3), 1633-1645) enables large routine dispersion-corrected DFT calculations at preserved accuracy. Furthermore, the DNN electron density-free features extend the MBD model's applicability beyond electronic structure theory within methodologies such as force fields and neural networks.
MoreTranslated text
Key words
Dispersion Correction,Density-Functional Theory,Hybrid Density Functionals,Molecular Simulations
AI Read Science
Must-Reading Tree
Example
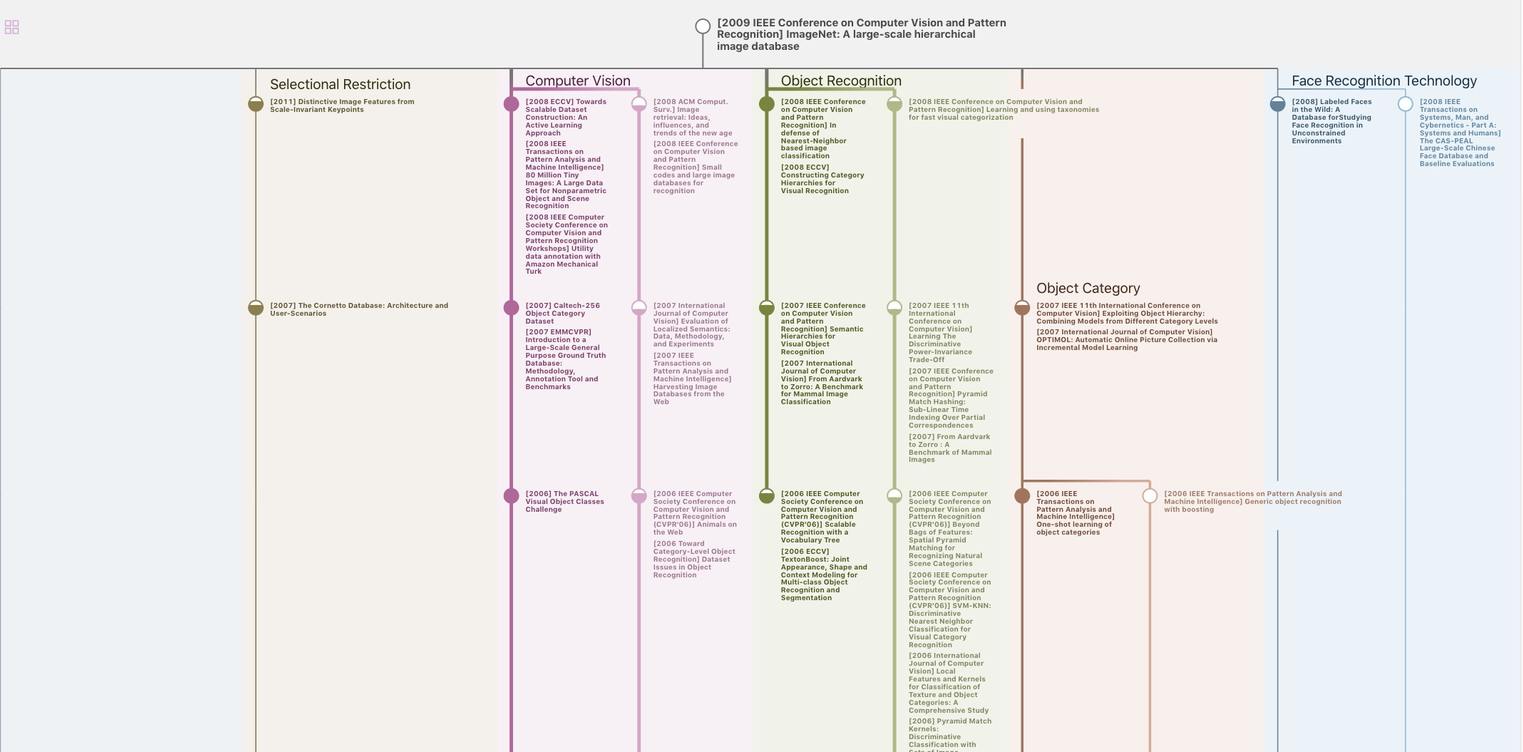
Generate MRT to find the research sequence of this paper
Chat Paper
Summary is being generated by the instructions you defined