Disentangling the Impacts of Language and Channel Variability on Speech Separation Networks
Conference of the International Speech Communication Association (INTERSPEECH)(2022)
摘要
Because the performance of speech separation is excellent for speech in which two speakers completely overlap, research attention has been shifted to dealing with more realistic scenarios. However, domain mismatch between training/test situations due to factors, such as speaker, content, channel, and environment, remains a severe problem for speech separation. Speaker and environment mismatches have been studied in the existing literature. Nevertheless, there are few studies on speech content and channel mismatches. Moreover, the impacts of language and channel in these studies are mostly tangled. In this study, we create several datasets for various experiments. The results show that the impacts of different languages are small enough to be ignored compared to the impacts of different channels. In our experiments, training on data recorded by Android phones leads to the best generalizability. Moreover, we provide a new solution for channel mismatch by evaluating projection, where the channel similarity can be measured and used to effectively select additional training data to improve the performance of in-the-wild test data.
更多查看译文
关键词
separation networks,channel variability
AI 理解论文
溯源树
样例
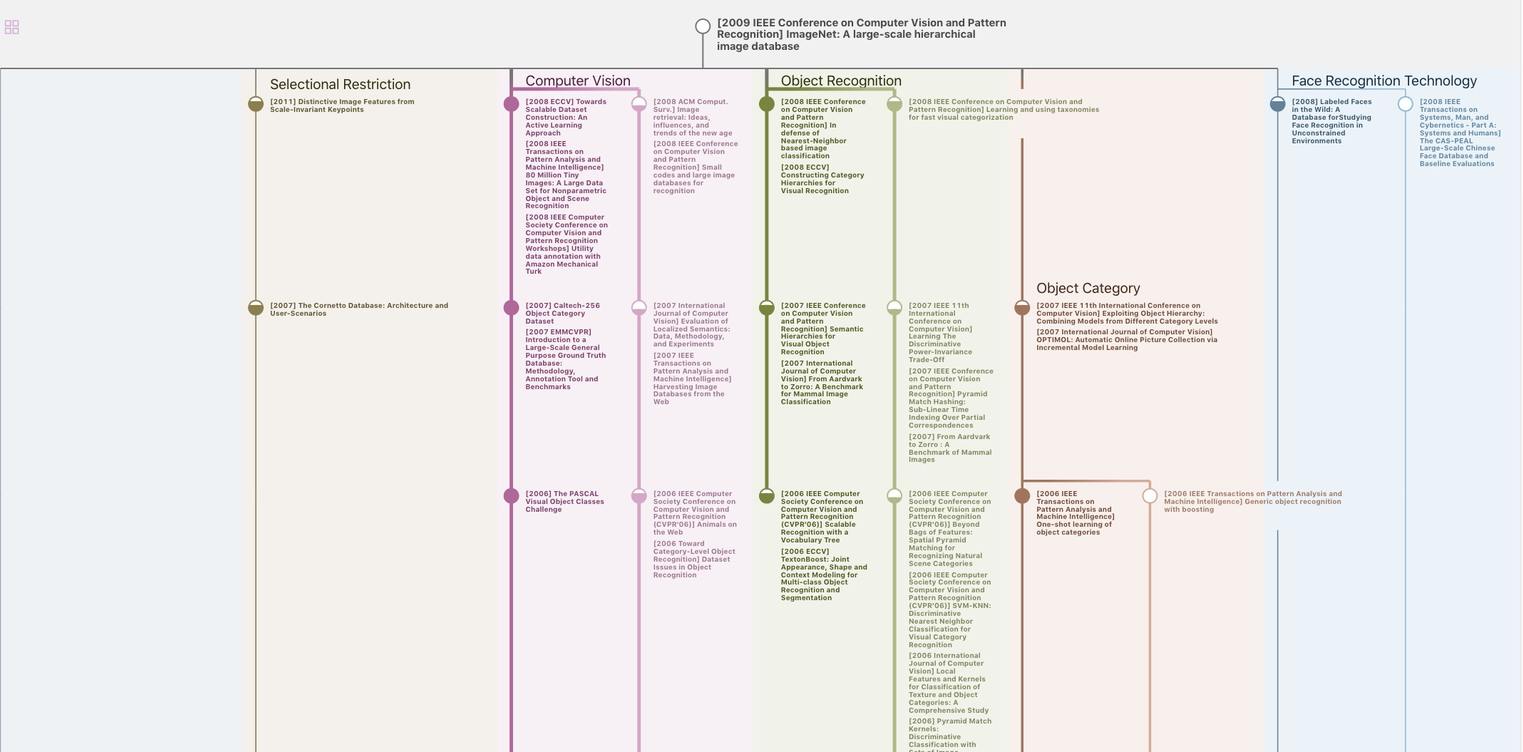
生成溯源树,研究论文发展脉络
Chat Paper
正在生成论文摘要