A Generative Adversarial Approach for Sybil Attacks Recognition for Vehicular Crowdsensing
International Conference on Connected Vehicles and Expo(2022)
摘要
Vehicular crowdsensing (VCS) is a subset of crowd-sensing where data collection is outsourced to group vehicles. Here, an entity interested in collecting data from a set of Places of Sensing Interest (PsI), advertises a set of sensing tasks, and the associated rewards. Vehicles attracted by the offered rewards deviate from their ongoing trajectories to visit and collect from one or more PsI. In this win-to-win scenario, vehicles reach their final destination with the extra reward, and the entity obtains the desired samples. Unfortunately, the efficiency of VCS can be undermined by the Sybil attack, in which an attacker can benefit from the injection of false vehicle identities. In this paper, we present a case study and analyze the effects of such an attack. We also propose a defense mechanism based on generative adversarial neural networks (GANs). We discuss GANs' advantages, and drawbacks in the context of VCS, and new trends in GANs' training that make them suitable for VCS.
更多查看译文
AI 理解论文
溯源树
样例
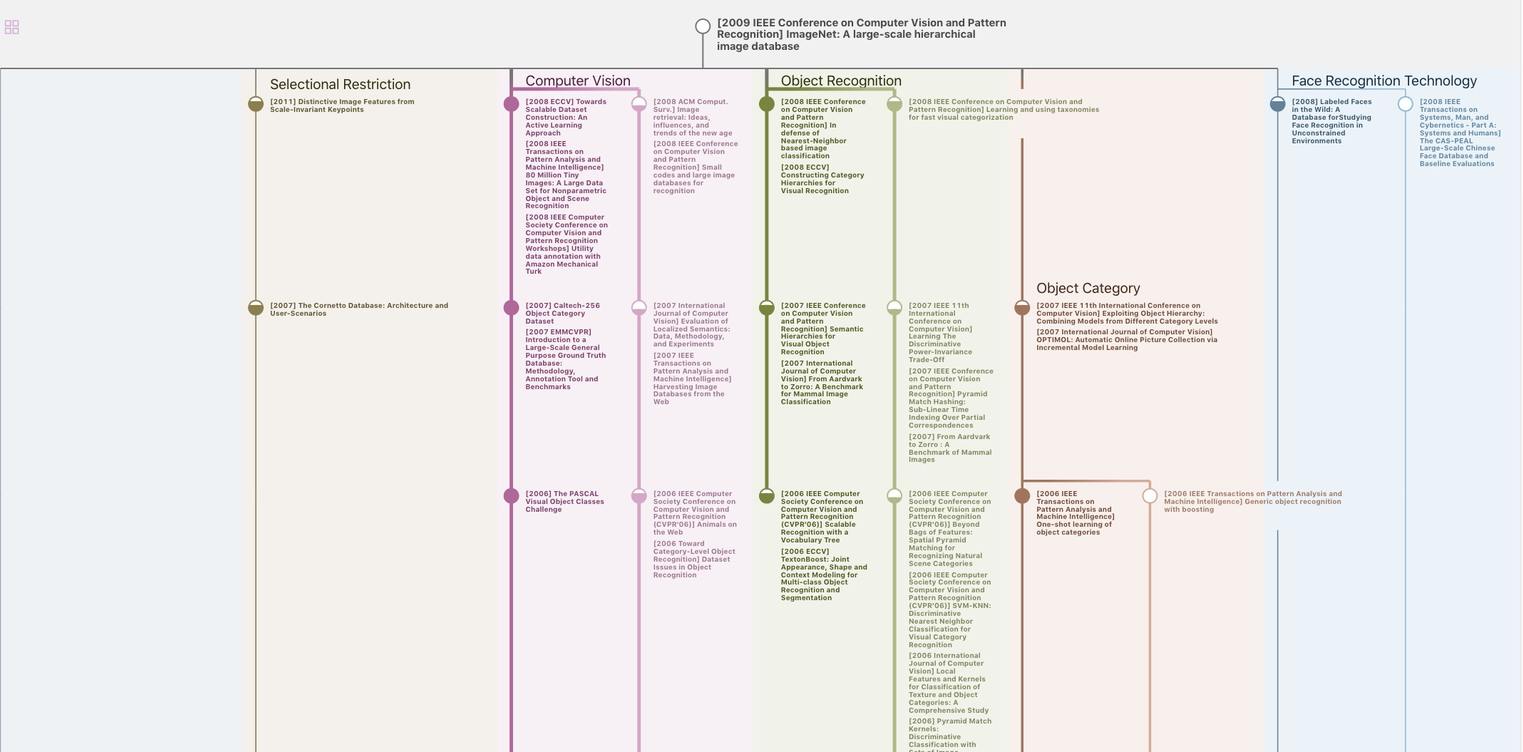
生成溯源树,研究论文发展脉络
Chat Paper
正在生成论文摘要