Calibrating constitutive models with full-field data via physics informed neural networks
arxiv(2022)
摘要
The calibration of solid constitutive models with full-field experimental data is a long-standing challenge, especially in materials which undergo large deformation. In this paper, we propose a physics-informed deep-learning framework for the discovery of constitutive model parameterizations given full-field displacement data and global force-displacement data. Contrary to the majority of recent literature in this field, we work with the weak form of the governing equations rather than the strong form to impose physical constraints upon the neural network predictions. The approach presented in this paper is computationally efficient, suitable for irregular geometric domains, and readily ingests displacement data without the need for interpolation onto a computational grid. A selection of canonical hyperelastic materials models suitable for different material classes is considered including the Neo-Hookean, Gent, and Blatz-Ko constitutive models as exemplars for general hyperelastic behavior, polymer behavior with lock-up, and compressible foam behavior respectively. We demonstrate that physics informed machine learning is an enabling technology and may shift the paradigm of how full-field experimental data is utilized to calibrate constitutive models under finite deformations.
更多查看译文
关键词
constitutive modelling,full-field data,inverse modelling,physics informed neural networks
AI 理解论文
溯源树
样例
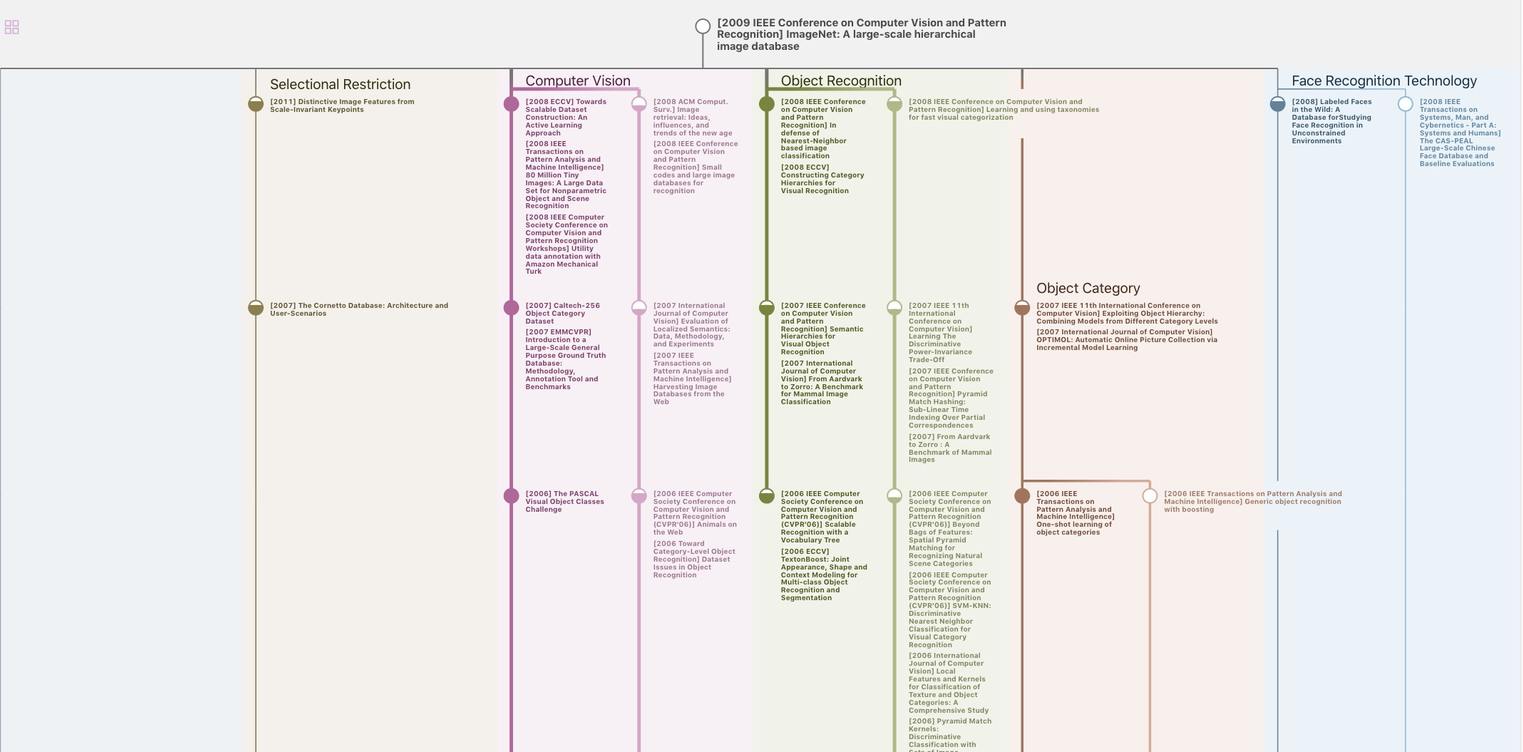
生成溯源树,研究论文发展脉络
Chat Paper
正在生成论文摘要