MBORE: Multi-objective Bayesian Optimisation by Density-Ratio Estimation
PROCEEDINGS OF THE 2022 GENETIC AND EVOLUTIONARY COMPUTATION CONFERENCE (GECCO'22)(2022)
摘要
Optimisation problems often have multiple conflicting objectives that can be computationally and/or financially expensive. Monosurrogate Bayesian optimisation (BO) is a popular model-based approach for optimising such black-box functions. It combines objective values via scalarisation and builds a Gaussian process (GP) surrogate of the scalarised values. The location which maximises a cheap-to-query acquisition function is chosen as the next location to expensively evaluate. While BO is an effective strategy, the use of GPs is limiting. Their performance decreases as the problem input dimensionality increases, and their computational complexity scales cubically with the amount of data. To address these limitations, we extend previous work on BO by density-ratio estimation (BORE) to the multi-objective setting. BORE links the computation of the probability of improvement acquisition function to that of probabilistic classification. This enables the use of state-of-the-art classifiers in a BO-like framework. In this work we present MBORE: multi-objective Bayesian optimisation by densityratio estimation, and compare it to BO across a range of synthetic and real-world benchmarks. We find that MBORE performs as well as or better than BO on a wide variety of problems, and that it outperforms BO on high-dimensional and real-world problems.
更多查看译文
关键词
Bayesian optimisation,Surrogate modelling,Scalarisation methods,Efficient multi-objective optimisation,Acquisition function
AI 理解论文
溯源树
样例
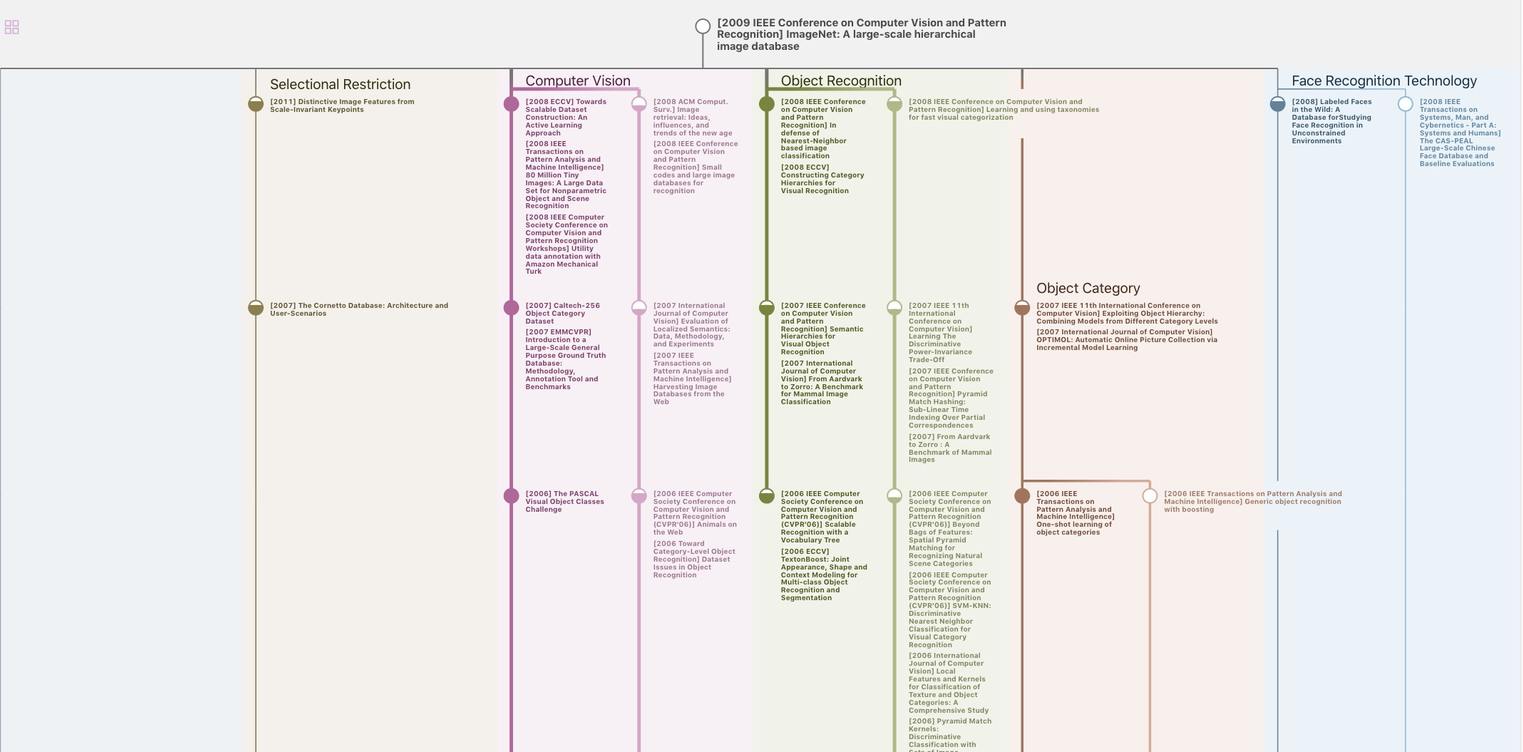
生成溯源树,研究论文发展脉络
Chat Paper
正在生成论文摘要