The Bethe Hessian and Information Theoretic Approaches for Online Change-Point Detection in Network Data
Sankhya A(2021)
摘要
Sequences of networks are currently a common form of network data sets. Identification of structural change-points in a network data sequence is a natural problem. The problem of change-point detection can be classified into two main types - offline change-point detection and online or sequential change-point detection. In this paper, we propose three different algorithms for online change-point detection based on certain cusum statistics for network data with community structures. For two of the proposed algorithms, we use information theoretic measures to construct the statistic for the estimation of a change-point. In the third algorithm, we use eigenvalues of the Bethe Hessian matrix to construct the statistic for the estimation of a change-point. We show the consistency property of the estimated change-point theoretically under networks generated from the multi-layer stochastic block model and the multi-layer degree-corrected block model. We also conduct an extensive simulation study to demonstrate the key properties of the algorithms as well as their efficacy.
更多查看译文
关键词
Change-point detection,Bethe hessian operator,Spectral clustering,Community detection,Sparse networks,Variation of information.
AI 理解论文
溯源树
样例
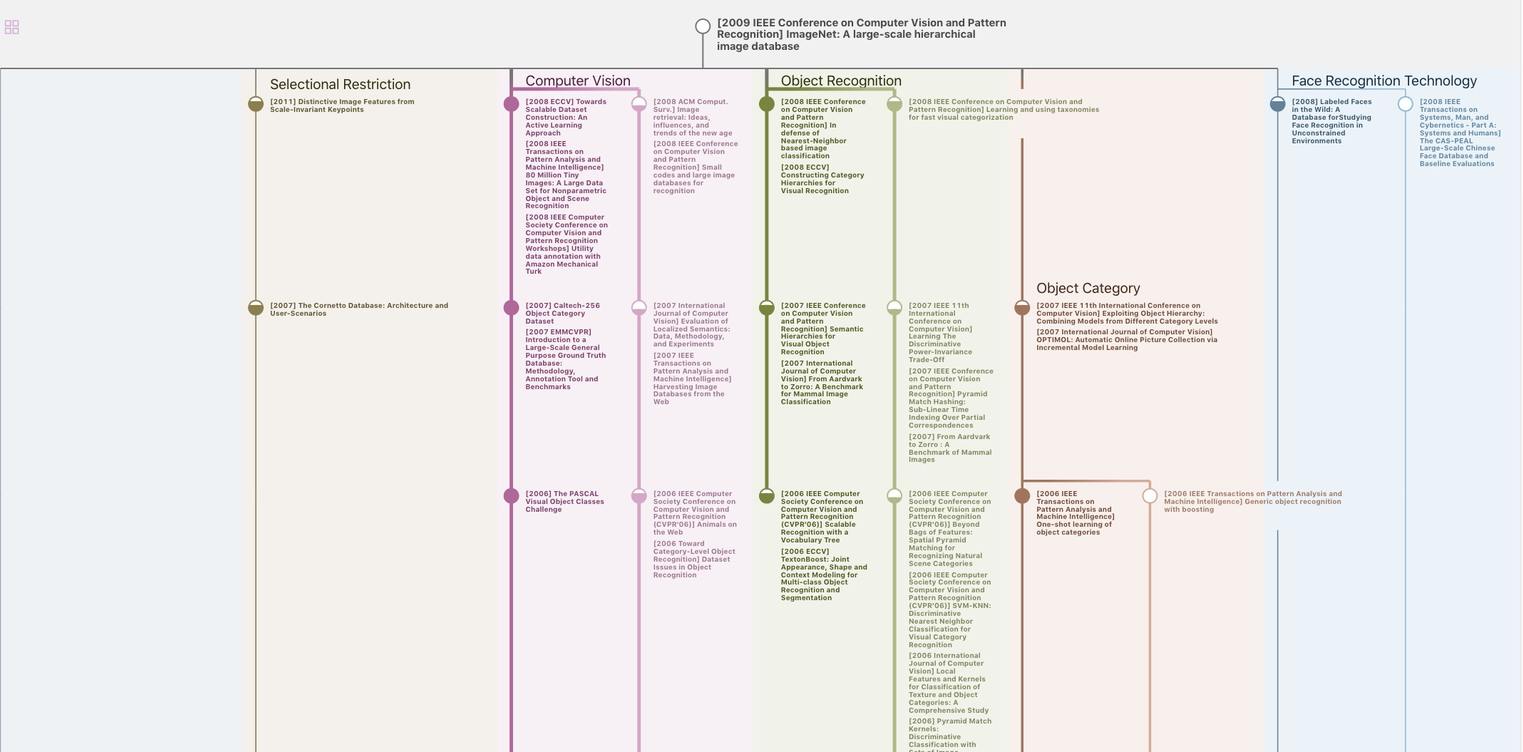
生成溯源树,研究论文发展脉络
Chat Paper
正在生成论文摘要