Self-supervised Calorie-aware Heterogeneous Graph Networks for Food Recommendation
ACM transactions on multimedia computing, communications and applications/ACM transactions on multimedia computing communications and applications(2023)
摘要
With the rapid development of online recipe sharing platforms, food recommendation is emerging as an important application. Although recent studies have made great progress on food recommendation, they have two shortcomings that are likely to affect the recommendation performance. (1) The relations between ingredients are not considered, which may lead to sub-optimal representations of recipes and further result in the neglect of the user’s personalized ingredient combination preference. (2) Existing methods do not consider the impact of users’ preferences on calories in users’ food decision-making process. In this article, we propose a Self-supervised Calorie-aware Heterogeneous Graph Network (SCHGN) to model the relations between ingredients and incorporate calories of food simultaneously. Specifically, we first incorporate users, recipes, ingredients, and calories into a heterogeneous graph and explicitly present the complex relations among them with directed edges. Then, we explore the co-occurrence relation of ingredients in different recipes via self-supervised ingredient prediction. To capture users’ dynamic preferences on calories of food, we learn calorie-aware user representations by hierarchical message passing and compute a comprehensive user-guided recipe representation by attention mechanism. The final food recommendation is accomplished based on the similarity between a user’s calorie-aware representation and the user-guided representation of a recipe. Extensive experiment results on benchmark datasets demonstrate the effectiveness of the proposed method.
更多查看译文
关键词
Food recommendation,recipe calories,heterogeneous graph,self-supervised learning
AI 理解论文
溯源树
样例
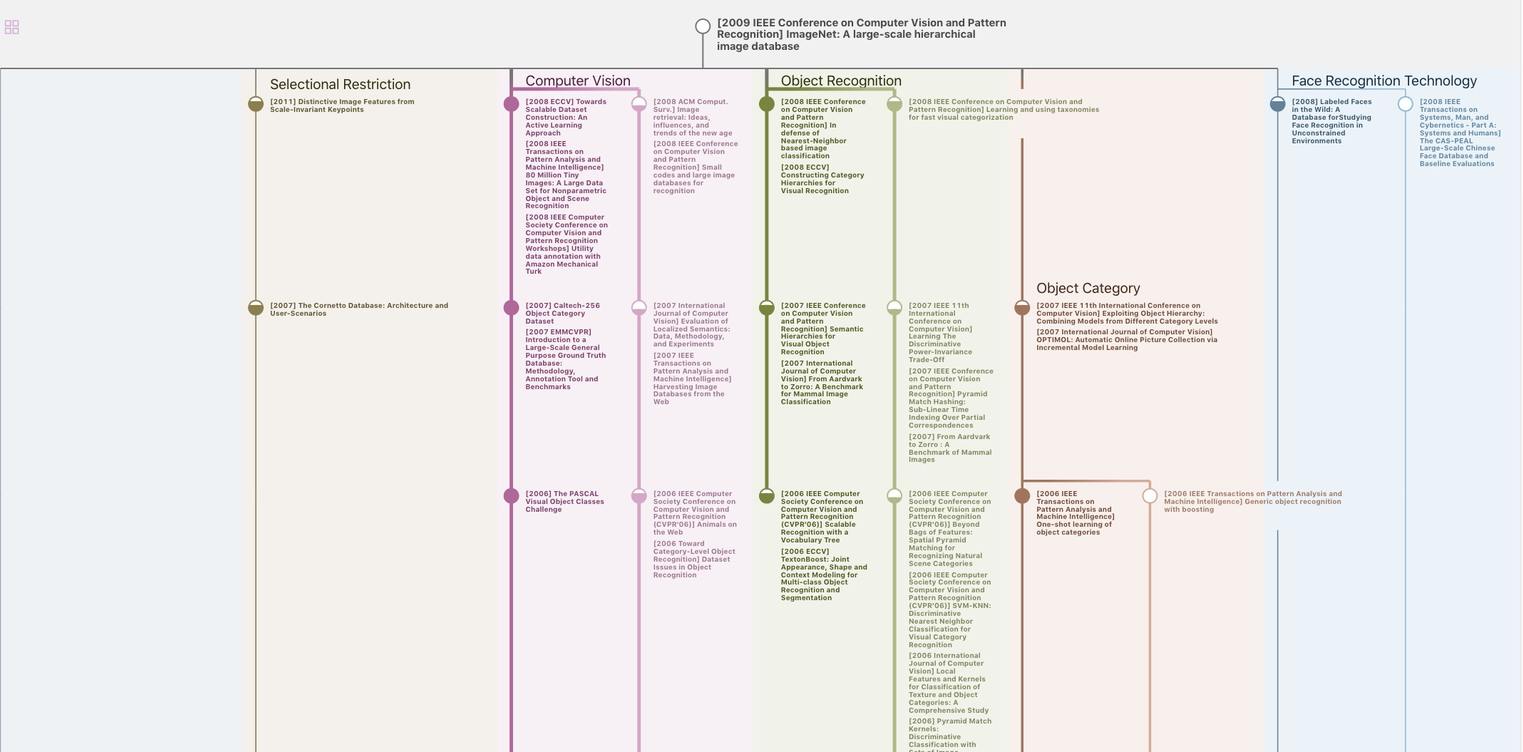
生成溯源树,研究论文发展脉络
Chat Paper
正在生成论文摘要