Simplicial Embeddings in Self-Supervised Learning and Downstream Classification
ICLR 2023(2022)
摘要
Simplicial Embeddings (SEM) are representations learned through self-supervised learning (SSL), wherein a representation is projected into $L$ simplices of $V$ dimensions each using a softmax operation. This procedure conditions the representation onto a constrained space during pretraining and imparts an inductive bias for group sparsity. For downstream classification, we formally prove that the SEM representation leads to better generalization than an unnormalized representation. Furthermore, we empirically demonstrate that SSL methods trained with SEMs have improved generalization on natural image datasets such as CIFAR-100 and ImageNet. Finally, when used in a downstream classification task, we show that SEM features exhibit emergent semantic coherence where small groups of learned features are distinctly predictive of semantically-relevant classes.
更多查看译文
关键词
Self-Supervised learning,Representation learning,Pre-training
AI 理解论文
溯源树
样例
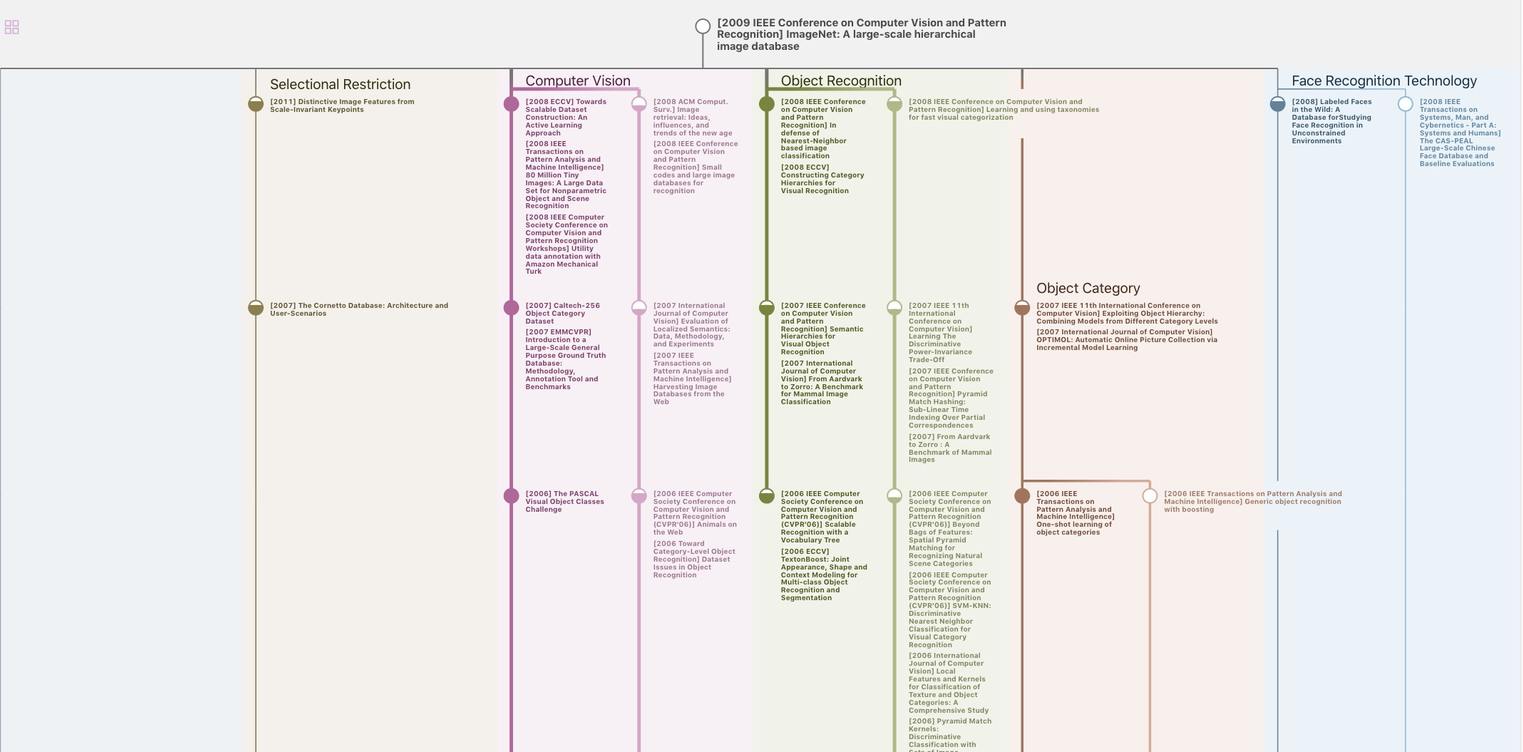
生成溯源树,研究论文发展脉络
Chat Paper
正在生成论文摘要