TAC: Optimizing Error-Bounded Lossy Compression for Three-Dimensional Adaptive Mesh Refinement Simulations
High Performance Distributed Computing(2022)
摘要
Today's scientific simulations require a significant reduction of data volume because of extremely large amounts of data they produce and the limited I/O bandwidth and storage space. Error-bounded lossy compression has been considered one of the most effective solutions to the above problem. However, little work has been done to improve error-bounded lossy compression for Adaptive Mesh Refinement (AMR) simulation data. Unlike the previous work that only leverages 1D compression, in this work, we propose to leverage high-dimensional (e.g., 3D) compression for each refinement level of AMR data. To remove the data redundancy across different levels, we propose three pre-process strategies and adaptively use them based on the data characteristics. Experiments on seven AMR datasets from a real-world large-scale AMR simulation demonstrate that our proposed approach can improve the compression ratio by up to 3.3x under the same data distortion, compared to the state-of-the-art method. In addition, we leverage the flexibility of our approach to tune the error bound for each level, which achieves much lower data distortion on two application-specific metrics.
更多查看译文
关键词
AMR, Lossy compression, scientific data, compression performance
AI 理解论文
溯源树
样例
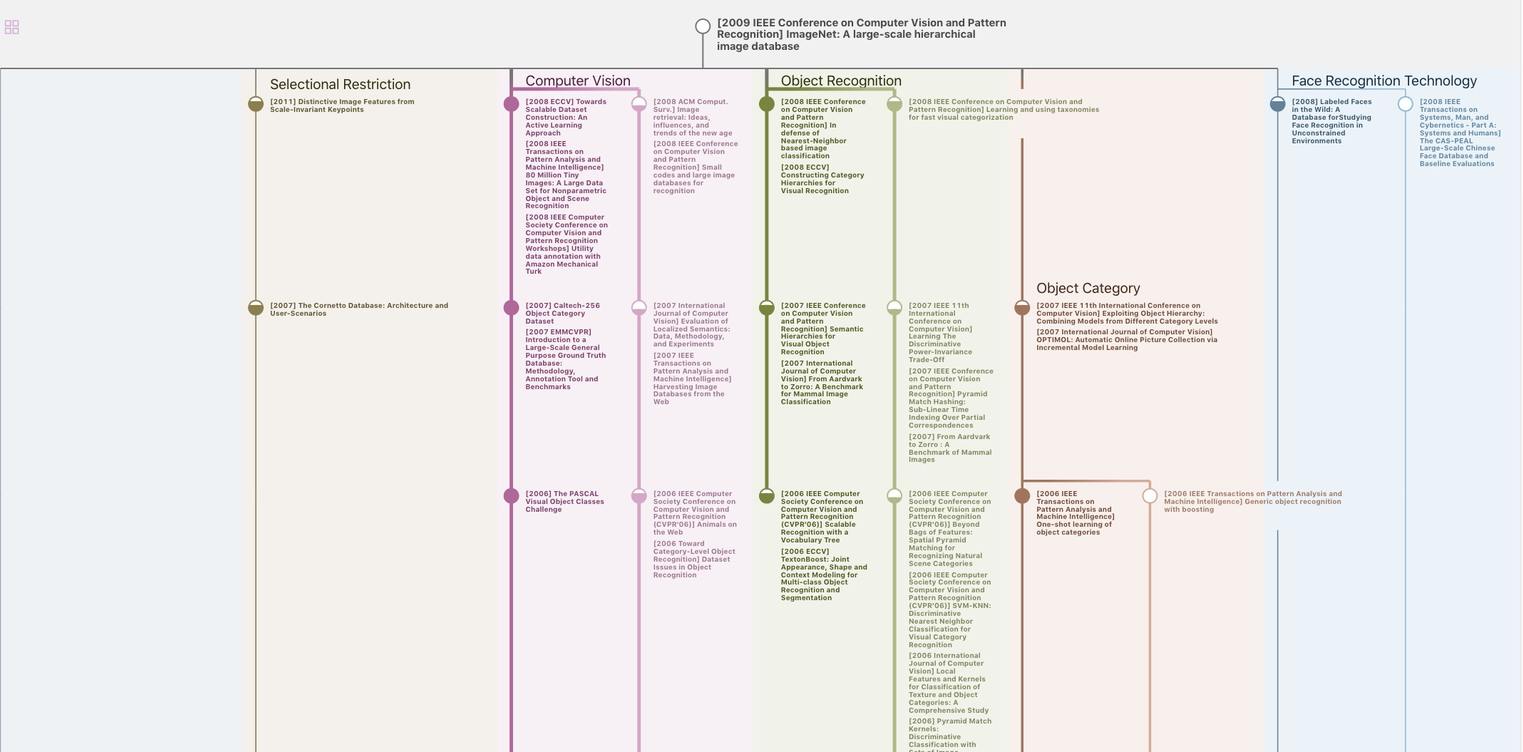
生成溯源树,研究论文发展脉络
Chat Paper
正在生成论文摘要