Safe Controller for Output Feedback Linear Systems using Model-Based Reinforcement Learning
arxiv(2022)
摘要
The objective of this research is to enable safety-critical systems to simultaneously learn and execute optimal control policies in a safe manner to achieve complex autonomy. Learning optimal policies via trial and error, i.e., traditional reinforcement learning, is difficult to implement in safety-critical systems, particularly when task restarts are unavailable. Safe model-based reinforcement learning techniques based on a barrier transformation have recently been developed to address this problem. However, these methods rely on full state feedback, limiting their usability in a real-world environment. In this work, an output-feedback safe model-based reinforcement learning technique based on a novel barrier-aware dynamic state estimator has been designed to address this issue. The developed approach facilitates simultaneous learning and execution of safe control policies for safety-critical linear systems. Simulation results indicate that barrier transformation is an effective approach to achieve online reinforcement learning in safety-critical systems using output feedback.
更多查看译文
关键词
safe controller,output feedback linear systems,reinforcement learning,model-based
AI 理解论文
溯源树
样例
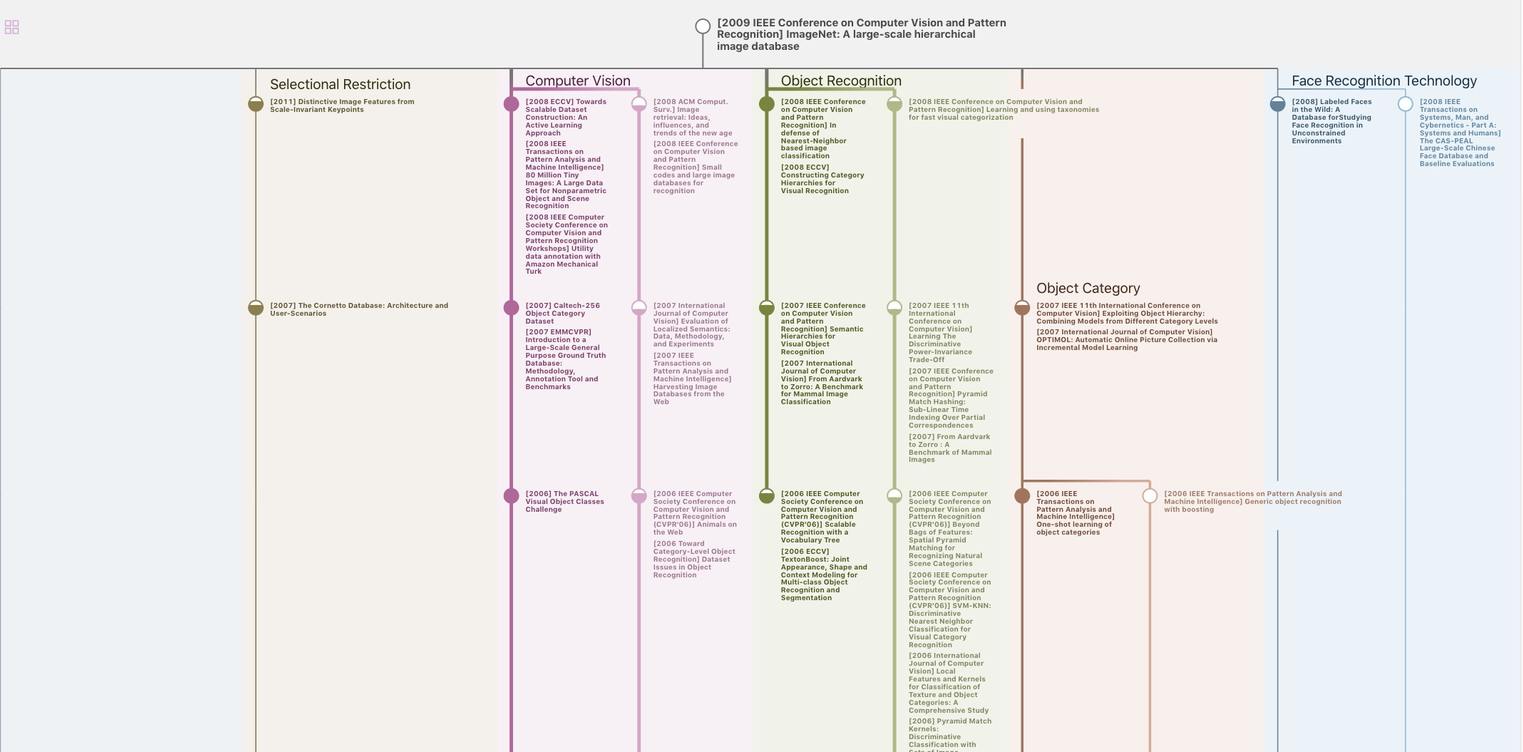
生成溯源树,研究论文发展脉络
Chat Paper
正在生成论文摘要