Artifacts of different dimension reduction methods on hybrid CNN feature hierarchy for Hyperspectral Image Classification
Optik(2021)
摘要
Hyperspectral Image Classification (HSIC) is a challenging task due to the spectral mixing effect which induces high intra-class variability and inter-class similarity. To overcome these limitations, Convolutional Neural Networks (CNNs) are utilized for feature extraction and classification. However, 3D CNNs are computationally expensive and 2D CNN alone cannot efficiently extract discriminating spectral–spatial features. Therefore, to overcome these challenges, this work presents a compact hybrid CNN model which overcomes the aforementioned challenges by distributing spatial–spectral feature extraction across 3D and 2D layers. An intensive preprocessing (several dimensional reduction methods) has been carried out to improve the classification results and to reduce the computational time. The experimental results show that the proposed pipeline outperformed in terms of generalization performance and statistical significance as compared to the state-of-the-art CNN models except commonly used computationally expensive design choices.
更多查看译文
关键词
Dimension reduction,Hybrid CNN,Hyperspectral Image Classification,Spectral–spatial information
AI 理解论文
溯源树
样例
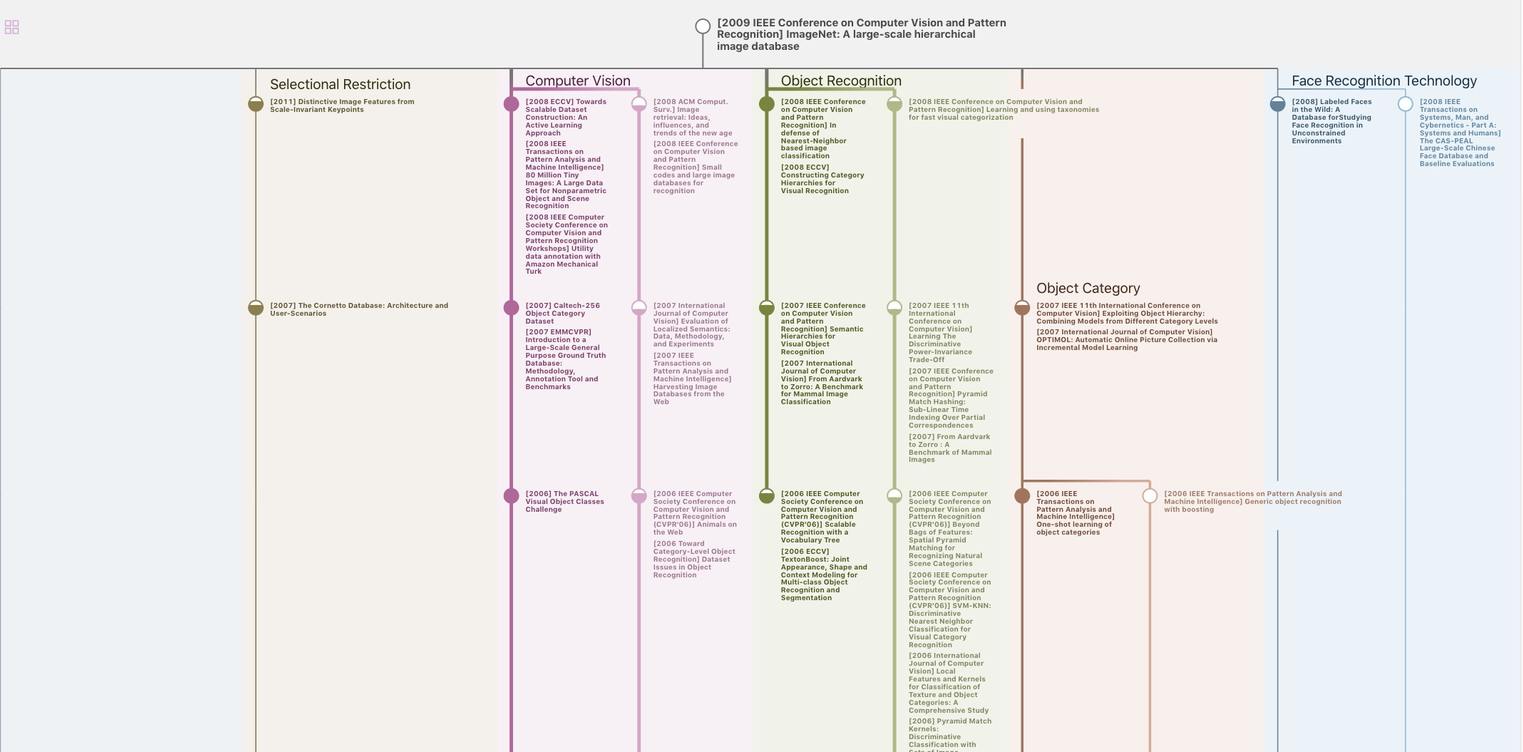
生成溯源树,研究论文发展脉络
Chat Paper
正在生成论文摘要