Multi-Agent Distributed Reinforcement Learning for Making Decentralized Offloading Decisions
IEEE INFOCOM 2022 - IEEE Conference on Computer Communications(2022)
摘要
We formulate computation offloading as a decentralized decision-making problem with autonomous agents. We design an interaction mechanism that incentivizes agents to align private and system goals by balancing between competition and cooperation. The mechanism provably has Nash equilibria with optimal resource allocation in the static case. For a dynamic environment, we propose a novel multi-agent online learning algorithm that learns with partial, delayed and noisy state information, and a reward signal that reduces information need to a great extent. Empirical results confirm that through learning, agents significantly improve both system and individual performance, e.g., 40% offloading failure rate reduction, 32% communication overhead reduction, up to 38% computation resource savings in low contention, 18% utilization increase with reduced load variation in high contention, and improvement in fairness. Results also confirm the algorithm’s good convergence and generalization property in significantly different environments.
更多查看译文
关键词
Offloading,Distributed Systems,Reinforcement Learning,Decentralized Decision-Making
AI 理解论文
溯源树
样例
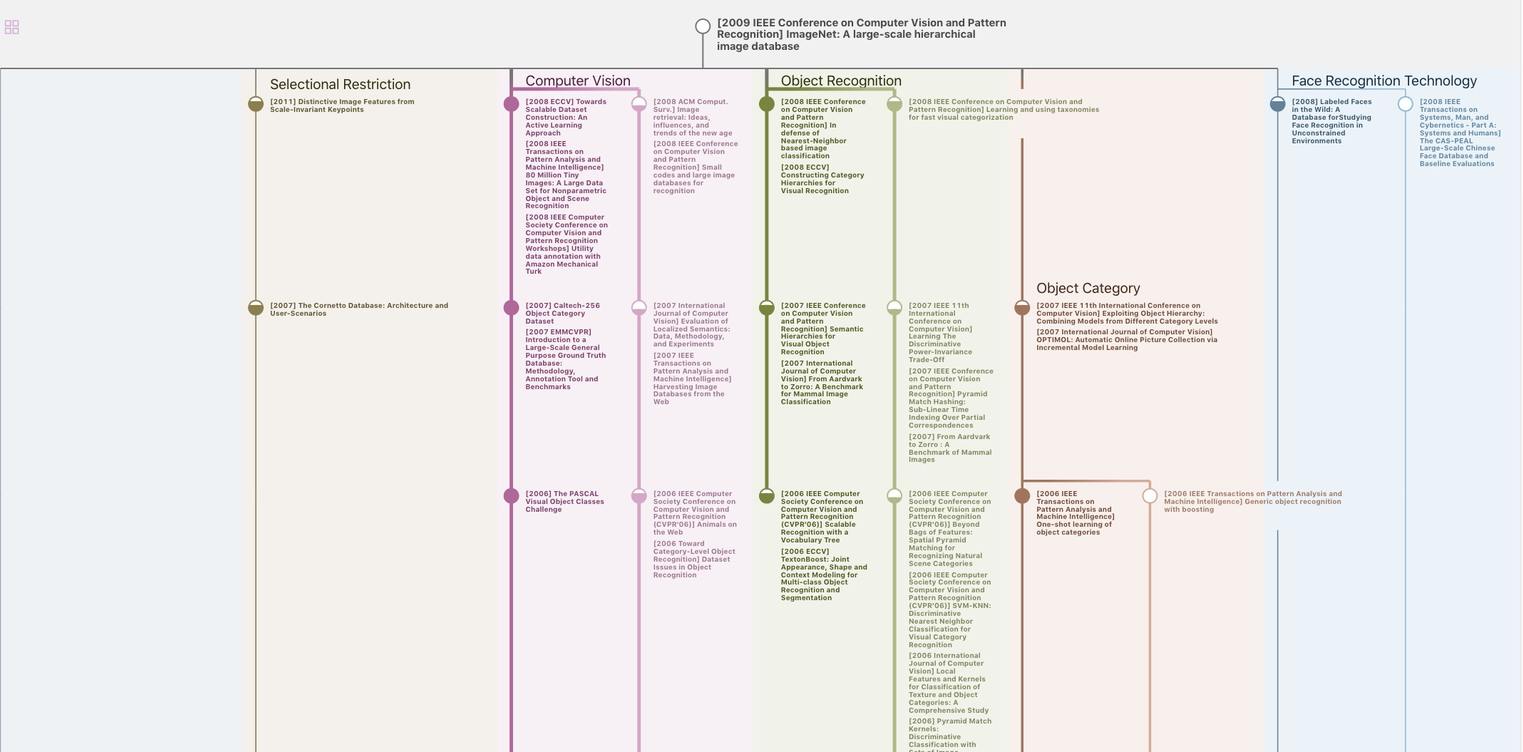
生成溯源树,研究论文发展脉络
Chat Paper
正在生成论文摘要