Cancer Subtyping via Embedded Unsupervised Learning on Transcriptomics Data.
Annual International Conference of the IEEE Engineering in Medicine and Biology Society (EMBC)(2022)
摘要
Cancer is one of the deadliest diseases worldwide. Accurate diagnosis and classification of cancer subtypes are indispensable for effective clinical treatment. Promising results on automatic cancer subtyping systems have been published recently with the emergence of various deep learning methods. However, such automatic systems often overfit the data due to the high dimensionality and scarcity. In this paper, we propose to investigate automatic subtyping from an unsupervised learning perspective by directly constructing the underlying data distribution itself, hence sufficient data can be generated to alleviate the issue of overfitting. Specifically, we bypass the strong Gaussianity assumption that typically exists but fails in the unsupervised learning subtyping literature due to small-sized samples by vector quantization. Our proposed method better captures the latent space features and models the cancer subtype manifestation on a molecular basis, as demonstrated by the extensive experimental results.
更多查看译文
关键词
embedded unsupervised learning,data,cancer
AI 理解论文
溯源树
样例
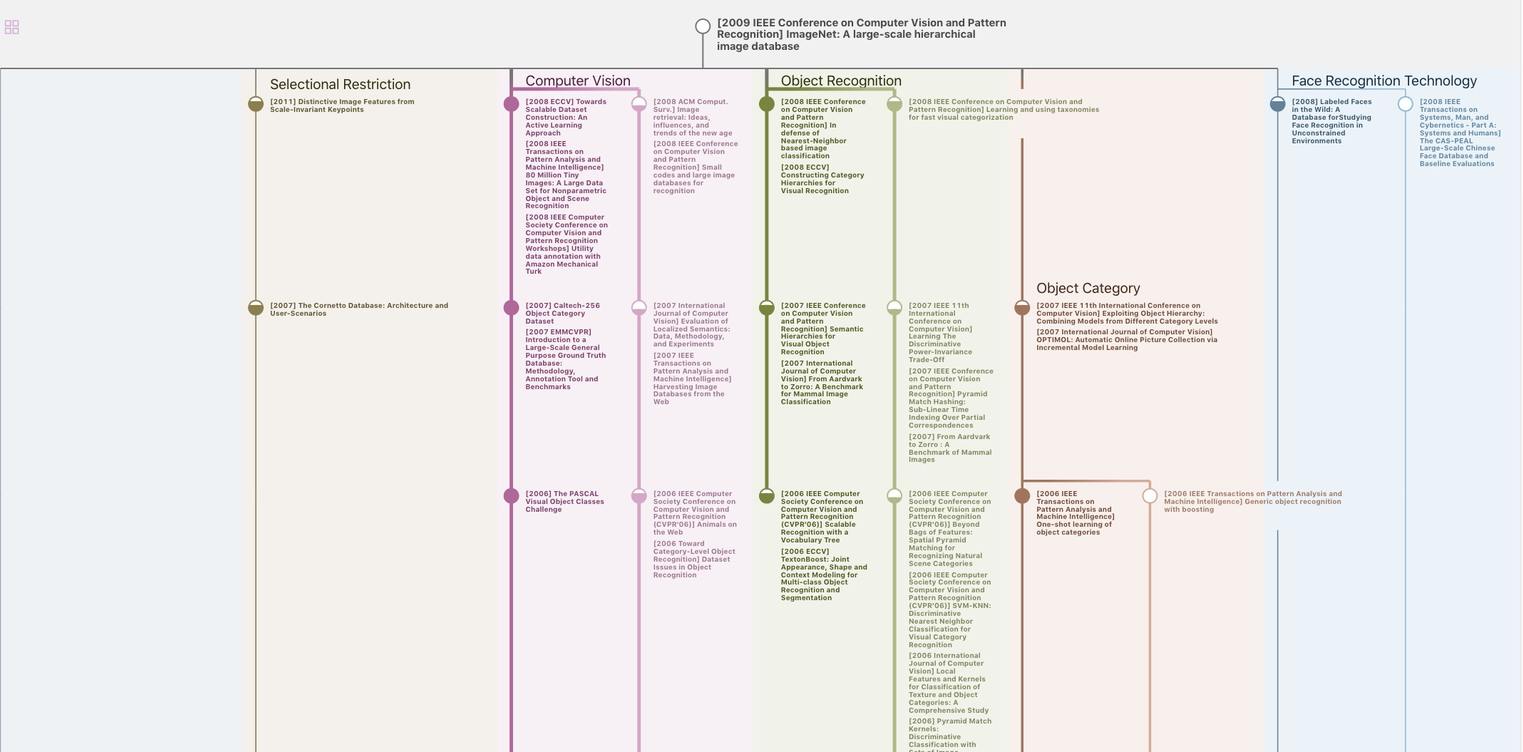
生成溯源树,研究论文发展脉络
Chat Paper
正在生成论文摘要