A New Complexity Metric for Nonconvex Rank-one Generalized Matrix Completion
Mathematical Programming(2023)
摘要
In this work, we develop a new complexity metric for an important class of low-rank matrix optimization problems in both symmetric and asymmetric cases, where the metric aims to quantify the complexity of the nonconvex optimization landscape of each problem and the success of local search methods in solving the problem. The existing literature has focused on two recovery guarantees. The RIP constant is commonly used to characterize the complexity of matrix sensing problems. On the other hand, the incoherence and the sampling rate are used when analyzing matrix completion problems. The proposed complexity metric has the potential to generalize these two notions and also applies to a much larger class of problems. To mathematically study the properties of this metric, we focus on the rank-1 generalized matrix completion problem and illustrate the usefulness of the new complexity metric on three types of instances, namely, instances with the RIP condition, instances obeying the Bernoulli sampling model, and a synthetic example. We show that the complexity metric exhibits a consistent behavior in the three cases, even when other existing conditions fail to provide theoretical guarantees. These observations provide a strong implication that the new complexity metric has the potential to generalize various conditions of optimization complexity proposed for different applications. Furthermore, we establish theoretical results to provide sufficient conditions and necessary conditions for the existence of spurious solutions in terms of the proposed complexity metric. This contrasts with the RIP and incoherence conditions that fail to provide any necessary condition.
更多查看译文
关键词
Matrix completion,Complexity metric,Nonconvex optimization,Global convergence,05C90,65F55,90C26
AI 理解论文
溯源树
样例
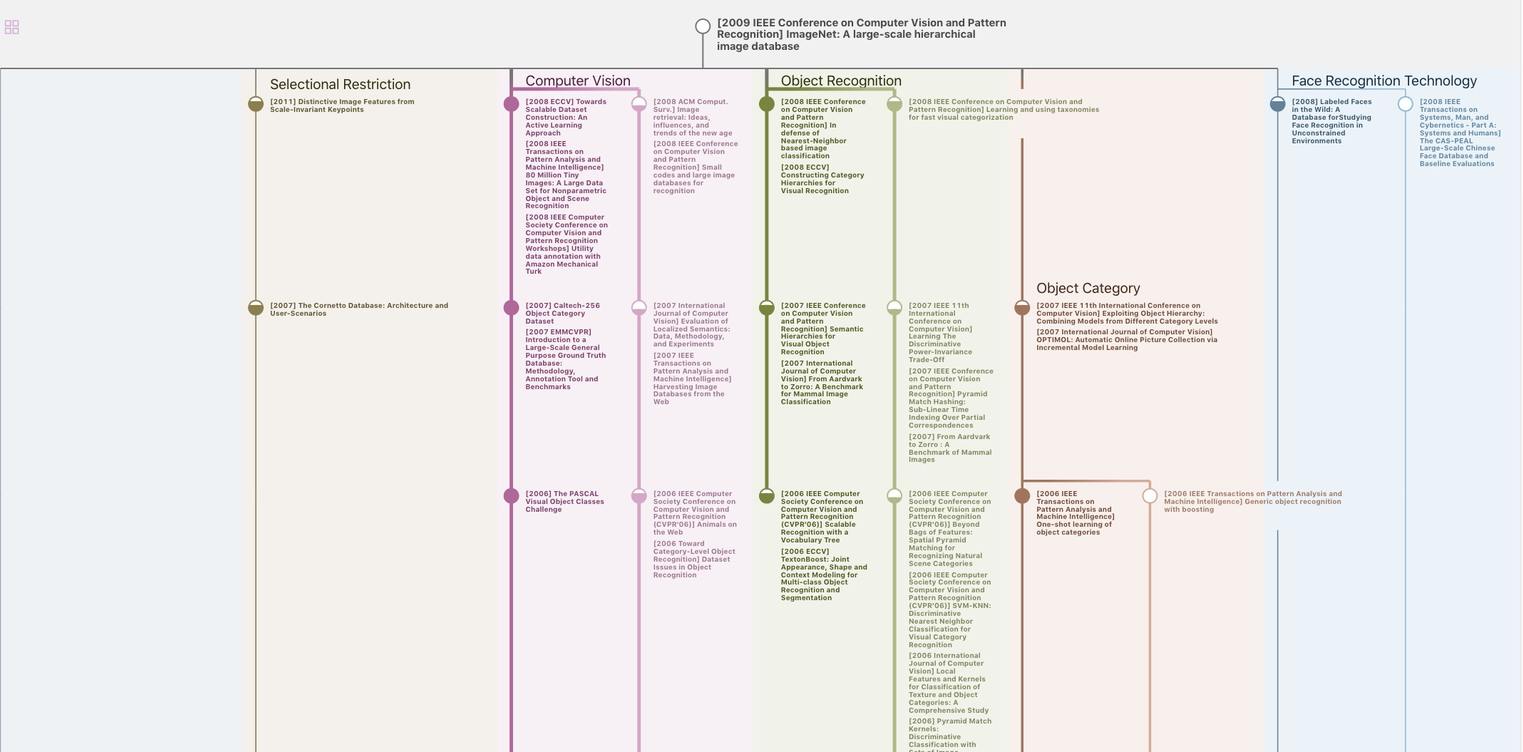
生成溯源树,研究论文发展脉络
Chat Paper
正在生成论文摘要