Sea level simulation with signal decomposition and machine learning
Ocean Engineering(2021)
摘要
In recent years, under the background of global warming, influenced by changing climate conditions and human activities, the sea level time series have shown great non-stationarity and randomness, making it difficult to predict sea levels scientifically and accurately. Sea level simulations and predictions under climate change are hot topics in global environment and climate studies. In this paper, three prediction models based on signal decomposition (time-varying filtering based empirical mode decomposition (TVF-EMD), wavelet transform (WT), and complementary ensemble empirical mode decomposition (CEEMD) coupled with the Elman neural network (ENN) are established, and the prediction performances of the monthly mean sea level (MMSL, time series length between 16 and 61 years) at 12 stations and daily mean sea level (DMSL, time series length is 790 days) at six stations are evaluated. The results show that the MMSL has multiple time scales. Among the TVF-EMD-ENN, WT-ENN and CEEMD-ENN models, the TVF-EMD-ENN model is the optimal model for predicting the MMSL and DMSL, in MMSL and DMSL prediction, the Nash-Sutcliffe efficiency coefficient (NSE) range is 0.98–1.0 and 0.974–0.995, respectively, NSE is greater than 0.97 and performing better than the ENN and long short-term memory (LSTM) model. The difference in the prediction results between the TVF-EMD-ENN, WT-ENN, and CEEMD-ENN are mainly caused by the first subcomponent (Sc-1). The performance of the TVF-EMD-ENN model is optimal because the contribution of Sc-1 to the original sequence is small, and the complexity of the Sc-1 model is low. The predicted future MMSL exhibits a period of alternating sea level rise and decline, and the rate of increase is relatively low. The proposed approach has great significance for sea-level prediction and environmental management.
更多查看译文
关键词
Decomposition methods,Elman neural network,Sea level prediction,Complexity,Contribution
AI 理解论文
溯源树
样例
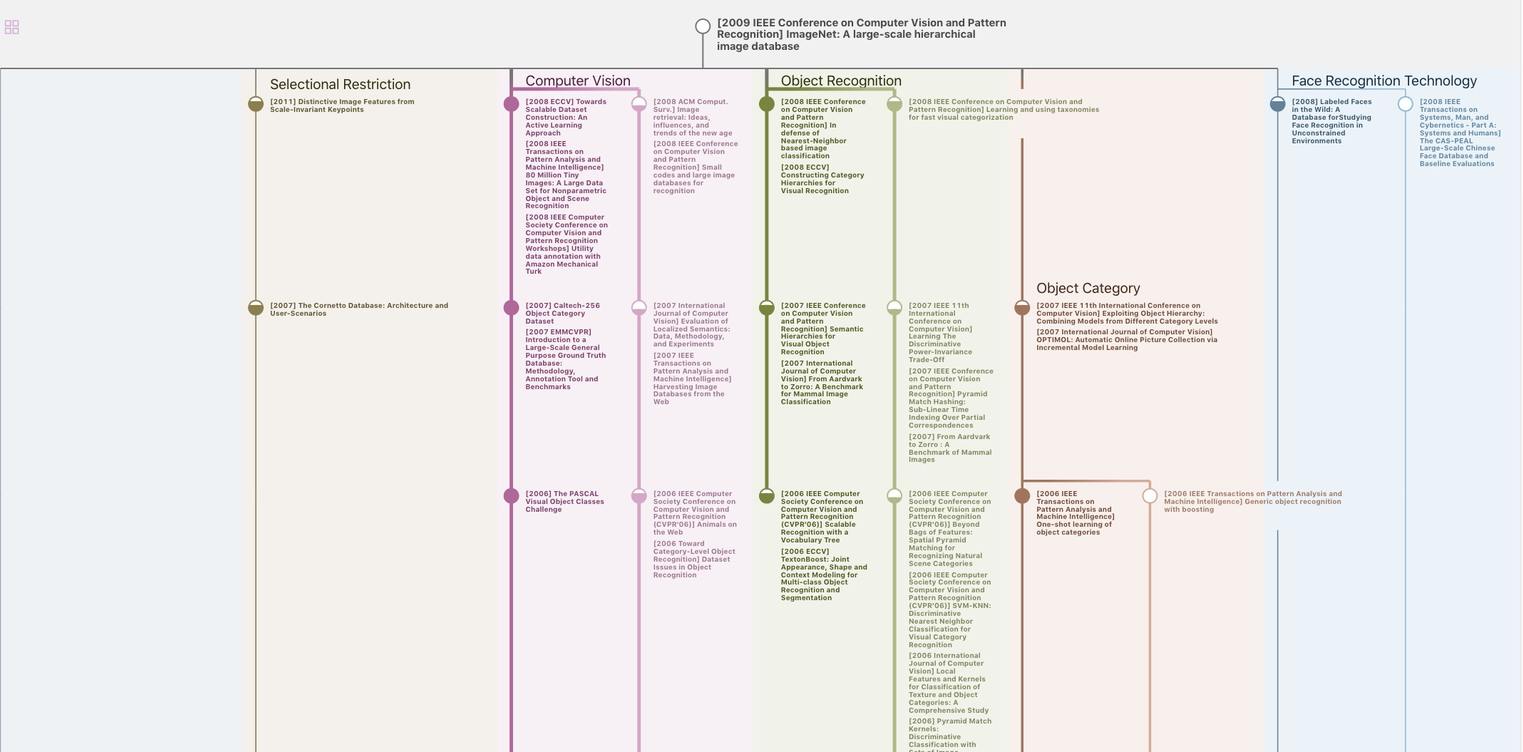
生成溯源树,研究论文发展脉络
Chat Paper
正在生成论文摘要