Data assimilation for deterministic prediction of vessel motion in real-time
Ocean Engineering(2022)
摘要
This paper describes the development of a data assimilation framework for deterministic vessel motion prediction in real-time, through the coupling of artificial neural network (ANN) and Ensemble Kalman Filter (EnKF). The ANN uses measurements of vessel motion and upstream wave elevation as inputs to predict future vessel motions. However, due to the uncertainties in the measurements and ANN, large errors may be built up over time. To improve the accuracy of the predictions, an EnKF has been used to combine measured data with the ANN predictions, to generate an updated prediction, which is subsequently used as input for the next prediction. Using a turret-moored Floating Production Storage Offloading (FPSO) vessel, the developed framework was first tested using synthesized measurements generated from numerical simulations and subsequently applied on experimental measurements of vessel motion. Sensitivity study was carried out to determine the data assimilation parameters, including the ensemble size and data assimilation interval. The data assimilation framework using ANN-EnKF is promising, in that it is able to improve the motion prediction accuracy for heave and pitch as compared to ANN, which do not incorporate measurements. The improvement is more obvious for the experimental measurements that have higher uncertainties.
更多查看译文
关键词
Data assimilation,Ensemble Kalman filter,Artificial neural network,Deterministic motion prediction,Floating structures,FPSO
AI 理解论文
溯源树
样例
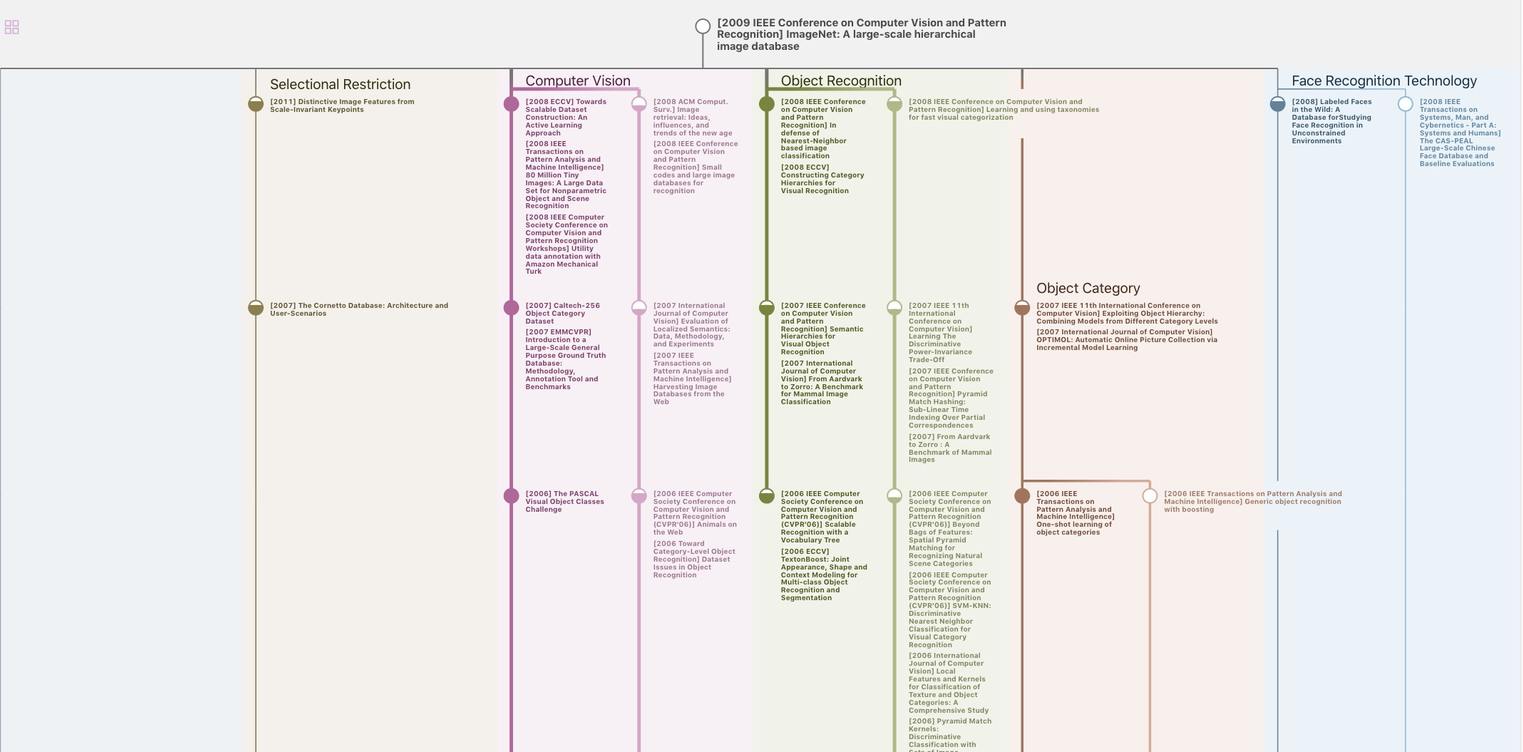
生成溯源树,研究论文发展脉络
Chat Paper
正在生成论文摘要