Improving Voice Trigger Detection with Metric Learning
Conference of the International Speech Communication Association (INTERSPEECH)(2022)
摘要
Voice trigger detection is an important task, which enables activating a voice assistant when a target user speaks a keyword phrase. A detector is typically trained on speech data independent of speaker information and used for the voice trigger detection task. However, such a speaker independent voice trigger detector typically suffers from performance degradation on speech from underrepresented groups, such as accented speakers. In this work, we propose a novel voice trigger detector that can use a small number of utterances from a target speaker to improve detection accuracy. Our proposed model employs an encoder-decoder architecture. While the encoder performs speaker independent voice trigger detection, similar to the conventional detector, the decoder predicts a personalized embedding for each utterance. A personalized voice trigger score is then obtained as a similarity score between the embeddings of enrollment utterances and a test utterance. The personalized embedding allows adapting to target speaker's speech when computing the voice trigger score, hence improving voice trigger detection accuracy. Experimental results show that the proposed approach achieves a 38% relative reduction in a false rejection rate (FRR) compared to a baseline speaker independent voice trigger model.
更多查看译文
关键词
keyword spotting, speaker recognition, personalization, metric learning
AI 理解论文
溯源树
样例
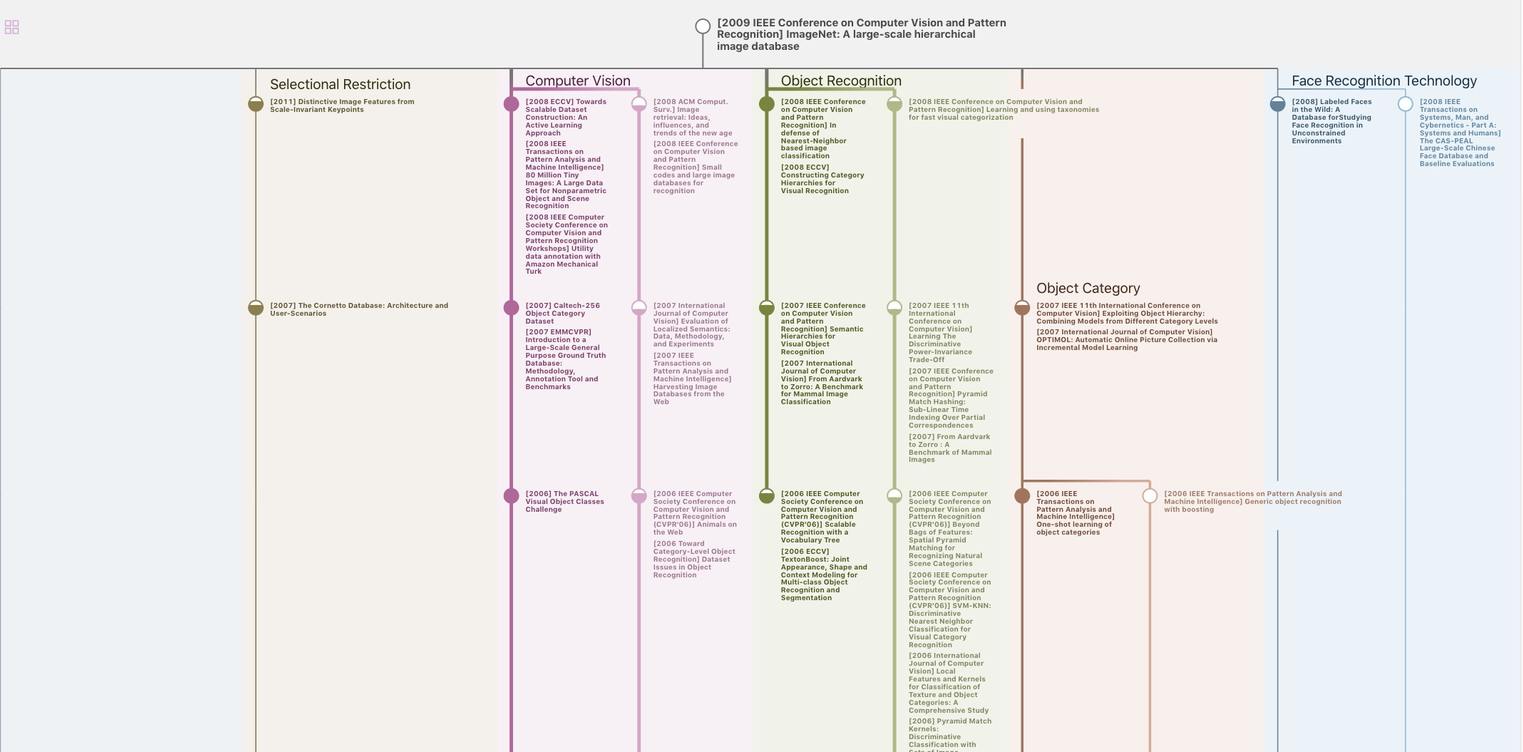
生成溯源树,研究论文发展脉络
Chat Paper
正在生成论文摘要