Hierarchical Self-supervised Representation Learning for Movie Understanding
IEEE Conference on Computer Vision and Pattern Recognition(2022)
摘要
Most self-supervised video representation learning approaches focus on action recognition. In contrast, in this paper we focus on self-supervised video learning for movie understanding and propose a novel hierarchical self-supervised pretraining strategy that separately pretrains each level of our hierarchical movie understanding model (based on [37]). Specifically, we propose to pretrain the low-level video backbone using a contrastive learning objective, while pretrain the higher-level video contextualizer using an event mask prediction task, which enables the usage of different data sources for pretraining different levels of the hierarchy. We first show that our self-supervised pre-training strategies are effective and lead to improved performance on all tasks and metrics on VidSitu benchmark [37] (e.g., improving on semantic role prediction from 47% to 61% CIDEr scores). We further demonstrate the effectiveness of our contextualized event features on LVU tasks [54], both when used alone and when combined with instance features, showing their complementarity.
更多查看译文
关键词
Self-& semi-& meta- Representation learning, Scene analysis and understanding, Video analysis and understanding
AI 理解论文
溯源树
样例
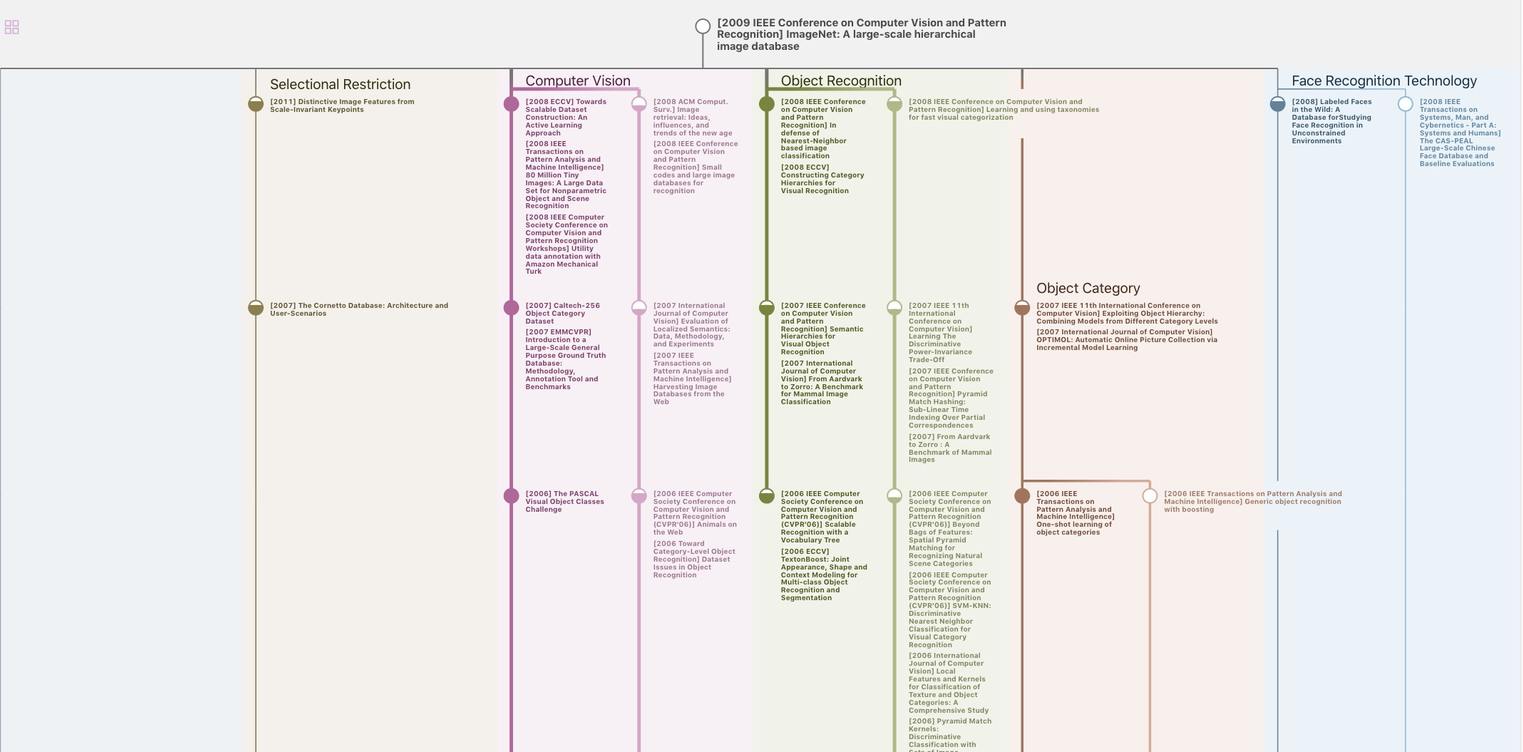
生成溯源树,研究论文发展脉络
Chat Paper
正在生成论文摘要