Deep transfer learning for system identification using long short-term memory neural networks
ArXiv(2022)
摘要
Recurrent neural networks (RNNs) have many advantages over more traditional system identification techniques. They may be applied to linear and nonlinear systems, and they require fewer modeling assumptions. However, these neural network models may also need larger amounts of data to learn and generalize. Furthermore, neural networks training is a time-consuming process. Hence, building upon long-short term memory neural networks (LSTM), this paper proposes using two types of deep transfer learning, namely parameter fine-tuning and freezing, to reduce the data and computation requirements for system identification. We apply these techniques to identify two dynamical systems, namely a second-order linear system and a Wiener-Hammerstein nonlinear system. Results show that compared with direct learning, our method accelerates learning by 10% to 50%, which also saves data and computing resources.
更多查看译文
关键词
deep transfer,system identification,neural networks,long,short-term
AI 理解论文
溯源树
样例
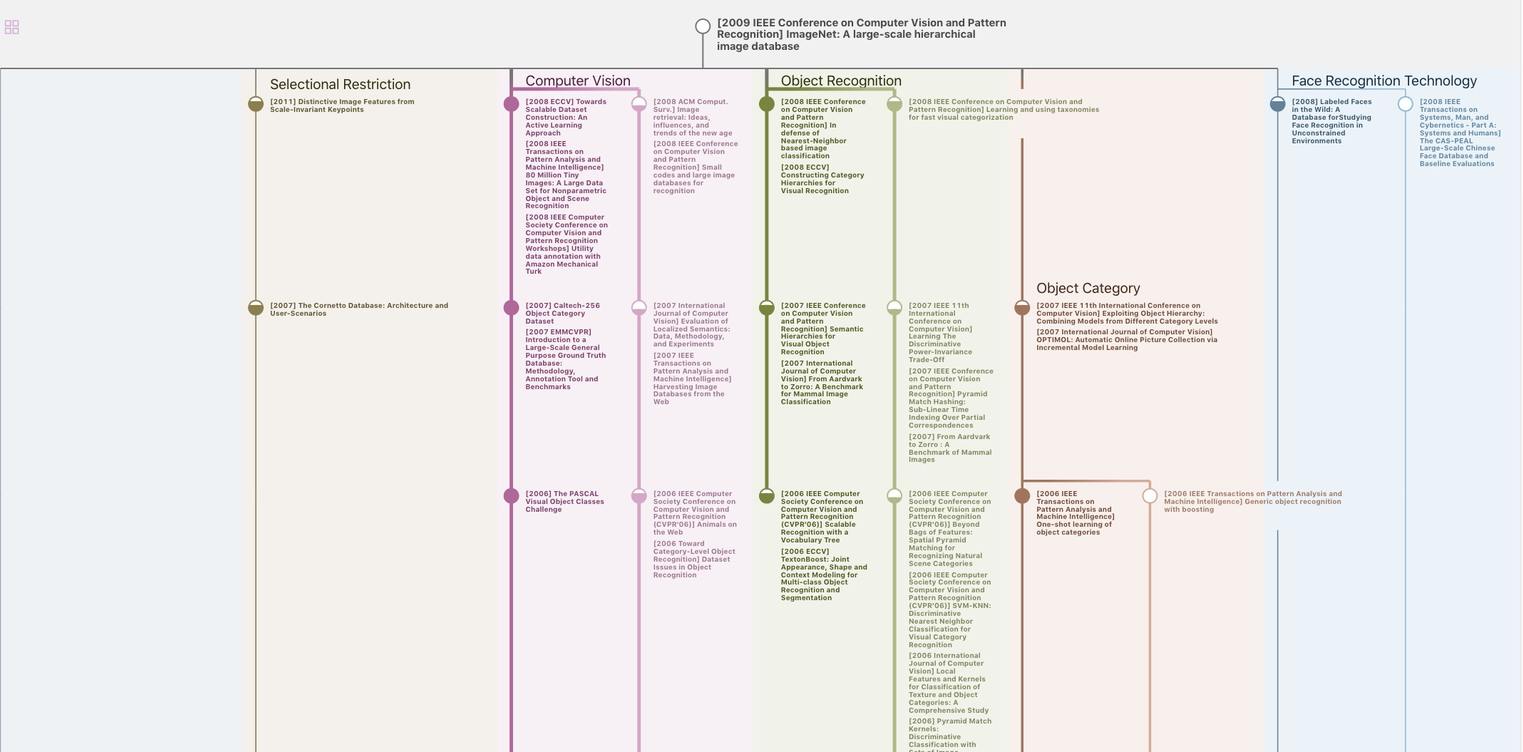
生成溯源树,研究论文发展脉络
Chat Paper
正在生成论文摘要