Flexible Sampling for Long-Tailed Skin Lesion Classification
MEDICAL IMAGE COMPUTING AND COMPUTER ASSISTED INTERVENTION, MICCAI 2022, PT III(2022)
摘要
Most of the medical tasks naturally exhibit a long-tailed distribution due to the complex patient-level conditions and the existence of rare diseases. Existing long-tailed learning methods usually treat each class equally to re-balance the long-tailed distribution. However, considering that some challenging classes may present diverse intra-class distributions, re-balancing all classes equally may lead to a significant performance drop. To address this, in this paper, we propose a curriculum learning-based framework called Flexible Sampling for the long-tailed skin lesion classification task. Specifically, we initially sample a subset of training data as anchor points based on the individual class prototypes. Then, these anchor points are used to pre-train an inference model to evaluate the per-class learning difficulty. Finally, we use a curriculum sampling module to dynamically query new samples from the rest training samples with the learning difficulty-aware sampling probability. We evaluated our model against several state-of-the-art methods on the ISIC dataset. The results with two long-tailed settings have demonstrated the superiority of our proposed training strategy, which achieves a new benchmark for long-tailed skin lesion classification.
更多查看译文
关键词
Long-tailed classification, Skin lesion, Flexible sampling
AI 理解论文
溯源树
样例
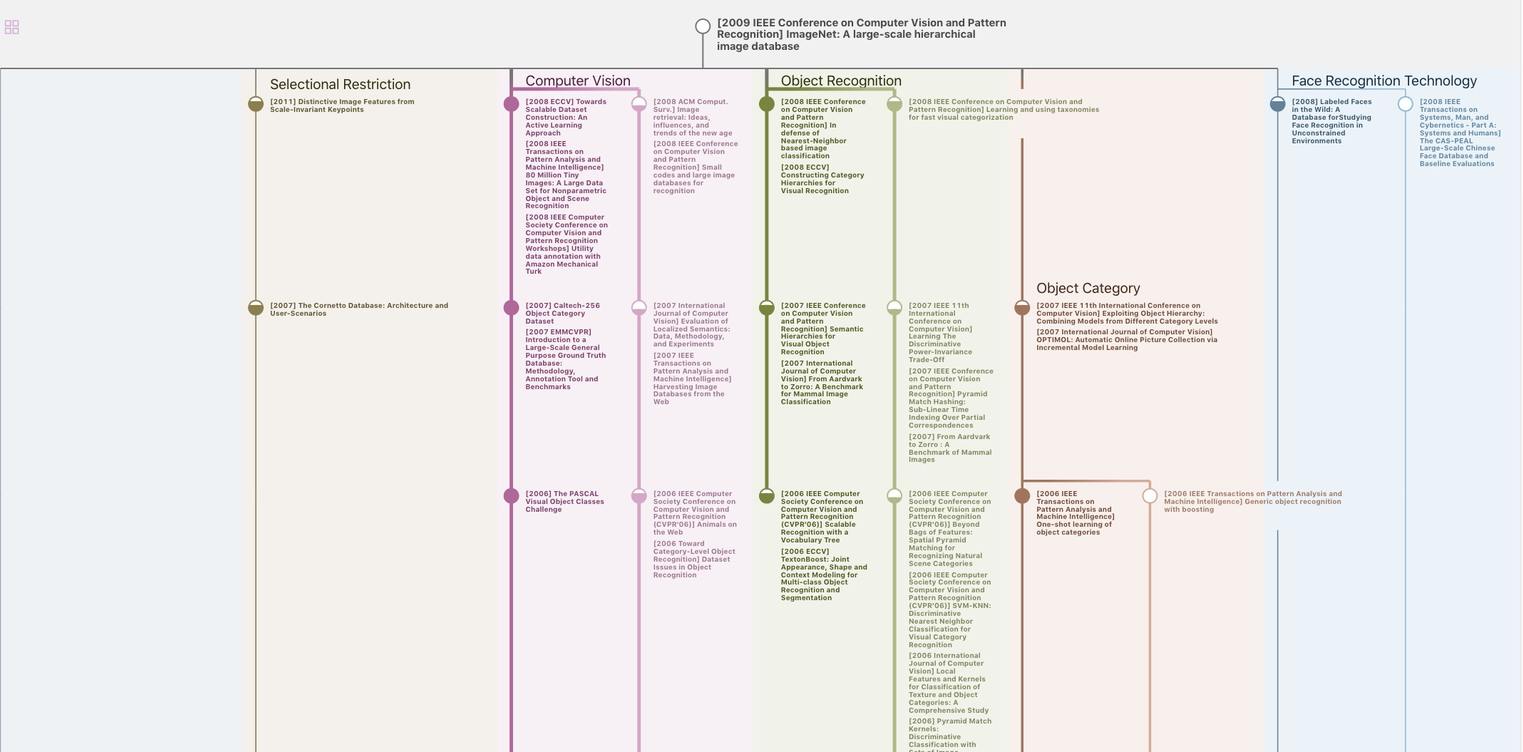
生成溯源树,研究论文发展脉络
Chat Paper
正在生成论文摘要