Heterogeneous Target Speech Separation
Conference of the International Speech Communication Association (INTERSPEECH)(2022)
摘要
We introduce a new paradigm for single-channel target source separation where the sources of interest can be distinguished using non-mutually exclusive concepts (e.g., loudness, gender, language, spatial location, etc). Our proposed heterogeneous separation framework can seamlessly leverage datasets with large distribution shifts and learn cross-domain representations under a variety of concepts used as conditioning. Our experiments show that training separation models with heterogeneous conditions facilitates the generalization to new concepts with unseen out-of-domain data while also performing substantially higher than single-domain specialist models. Notably, such training leads to more robust learning of new harder source separation discriminative concepts and can yield improvements over permutation invariant training with oracle source selection. We analyze the intrinsic behavior of source separation training with heterogeneous metadata and propose ways to alleviate emerging problems with challenging separation conditions. We release the collection of preparation recipes for all datasets used to further promote research towards this challenging task.
更多查看译文
关键词
target speech separation, semi-supervised learning, heterogeneous conditions, conditional inference
AI 理解论文
溯源树
样例
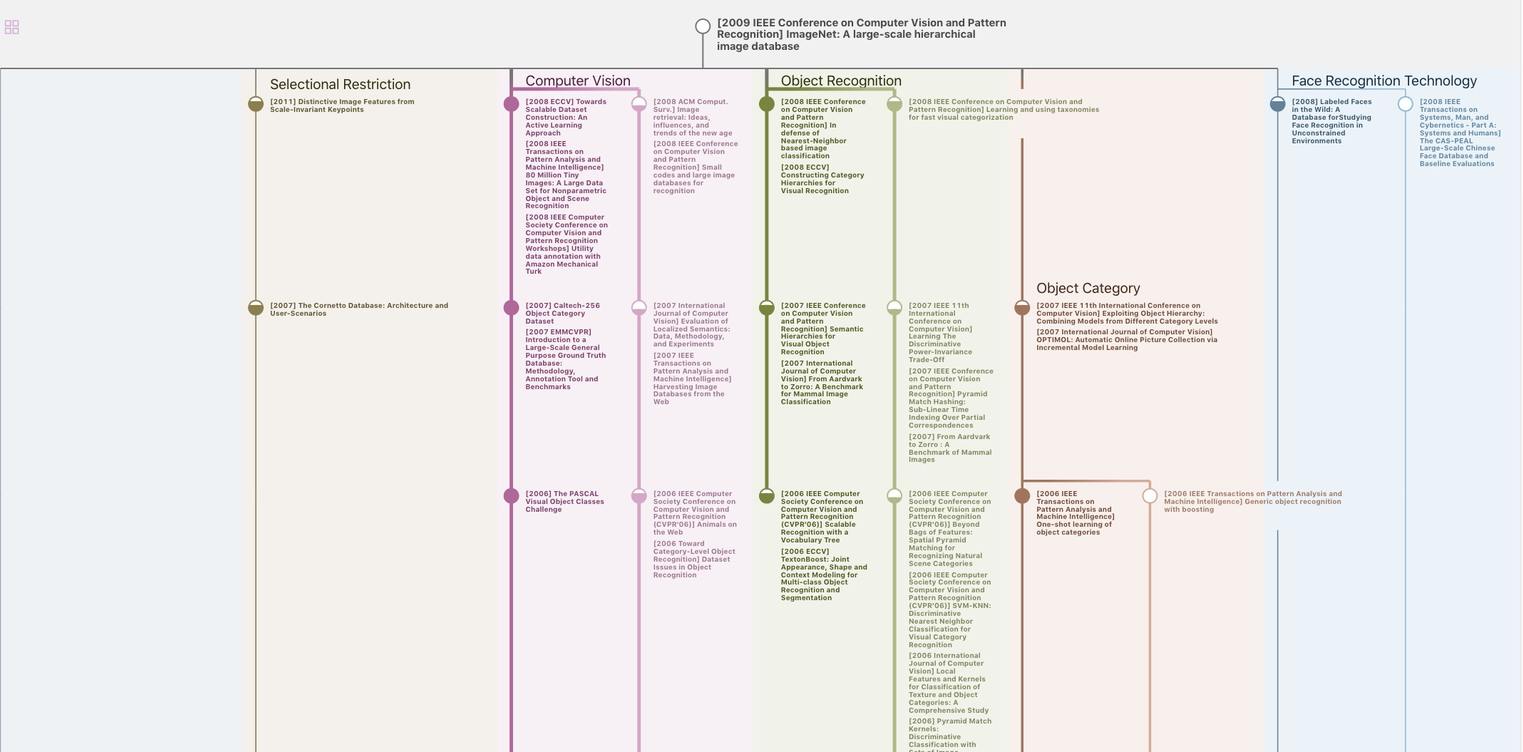
生成溯源树,研究论文发展脉络
Chat Paper
正在生成论文摘要