wenda_gpu: fast domain adaptation for genomic data
bioRxiv(2022)
摘要
Motivation Domain adaptation allows for development of predictive models even in cases with limited sample data. Weighted elastic net domain adaptation specifically leverages features of genomic data to maximize transferability but the method is too computationally demanding to apply to many genome-sized datasets.
Results We developed wenda\_gpu, which uses GPyTorch to train models on genomic data within hours on a single GPU-enabled machine. We show that wenda\_gpu returns comparable results to the original wenda implementation, and that it can be used for improved prediction of cancer mutation status on small sample sizes than regular elastic net.
Availability wenda_gpu is available on GitHub at .
Contact casey.s.greene{at}cuanschutz.edu
Supplementary information Supplementary data are available at Bioinformatics online.
### Competing Interest Statement
The authors have declared no competing interest.
更多查看译文
AI 理解论文
溯源树
样例
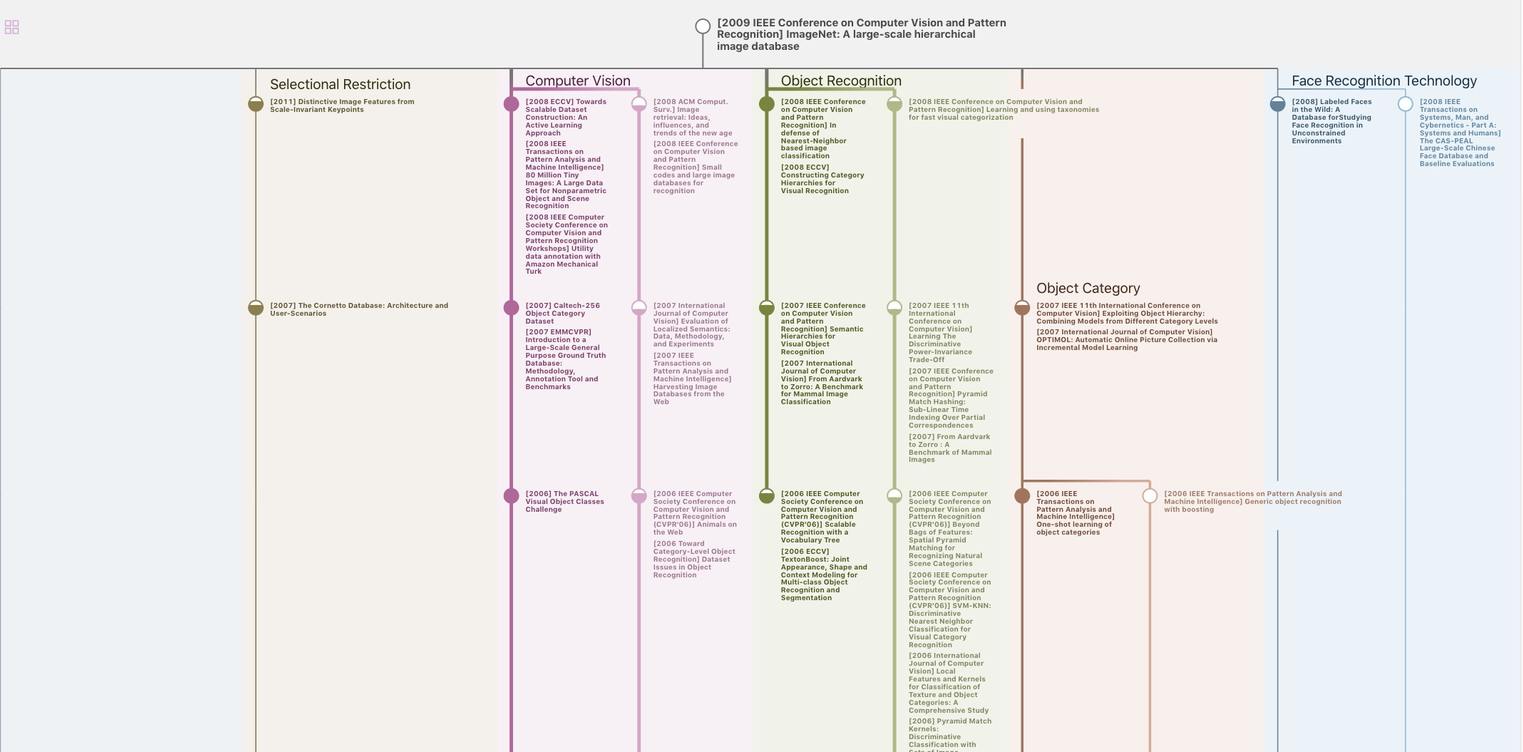
生成溯源树,研究论文发展脉络
Chat Paper
正在生成论文摘要