Adaptive Online Capacity Prediction Based on Transfer Learning for Fast Charging Lithium-Ion Batteries
Energy(2022)
摘要
The aging of the battery is complicated and depends on both internal and external factors. Fast charging will amplify the cell-to-cell differences and make battery capacity prediction more challenging. In this paper, a long short-term memory network-based transfer learning model is proposed for adaptive online capacity prediction under fast charging. First, a novel voltage feature of charging to 80% state of charge in about 10 min is introduced. A sliding window is designed to integrate the voltage feature and the cycle number. The feature is highly practical and can be easily measured in all fast charging conditions. Second, to deal with the cell-to-cell differences and improve the model adaptivity, a cross-validation method with both high-and low-similarity tasks is performed to derive optimal hyperparameters. Then, the offline model can be trained using the existing complete battery lifespan data. Third, with the arrival of new battery data, the model can be finetuned at the full connected layer. The well-adjusted model can be applied for online capacity prediction. The other four features are compared to prove the superiority of the proposed feature. Six experiments with different fast charging conditions are carried out to verify the effectiveness and adaptability of the proposed method.(c) 2022 Elsevier Ltd. All rights reserved.
更多查看译文
关键词
Lithium-ion battery,Capacity prediction,Fast charging,Integrated feature,Adaptivity,Transfer learning
AI 理解论文
溯源树
样例
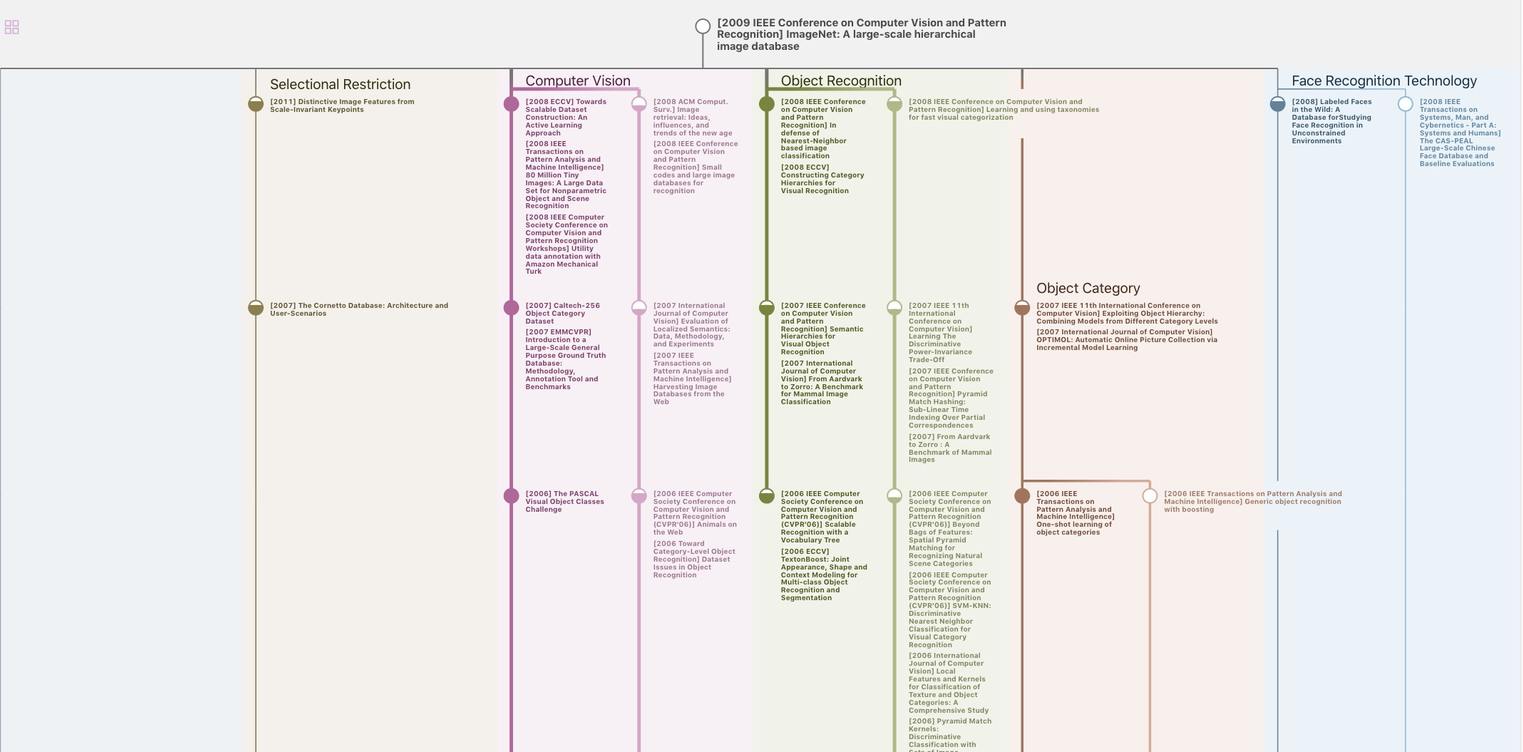
生成溯源树,研究论文发展脉络
Chat Paper
正在生成论文摘要