Assessing Methods and Tools to Improve Reporting, Increase Transparency, and Reduce Failures in Machine Learning Applications in Health Care
RADIOLOGY-ARTIFICIAL INTELLIGENCE(2022)
摘要
Artificial intelligence applications for health care have come a long way. Despite the remarkable progress, there are several examples of unfulfilled promises and outright failures. There is still a struggle to translate successful research into successful real-world applications. Machine learning (ML) products diverge from traditional software products in fundamental ways. Particularly, the main component of an ML solution is not a specific piece of code that is written for a specific purpose; rather, it is a generic piece of code, a model, customized by a training process driven by hyperparameters and a dataset. Datasets are usually large, and models are opaque. Therefore, datasets and models cannot be inspected in the same, direct way as traditional software products. Other methods are needed to detect failures in ML products. This report investigates recent advancements that promote auditing, supported by transparency, as a mechanism to detect potential failures in ML products for health care applications. It reviews practices that apply to the early stages of the ML lifecycle, when datasets and models are created; these stages are unique to ML products. Concretely, this report demonstrates how two recently proposed checklists, datasheets for datasets and model cards, can be adopted to increase the transparency of crucial stages of the ML lifecycle, using ChestX-ray8 and CheXNet as examples. The adoption of checklists to document the strengths, limitations, and applications of datasets and models in a structured format leads to increased transparency, allowing early detection of potential problems and opportunities for improvement. (C) RSNA, 2022
更多查看译文
关键词
Artificial Intelligence, Machine Learning, Lifecycle, Auditing, Transparency, Failures, Datasheets, Datasets, Model Cards
AI 理解论文
溯源树
样例
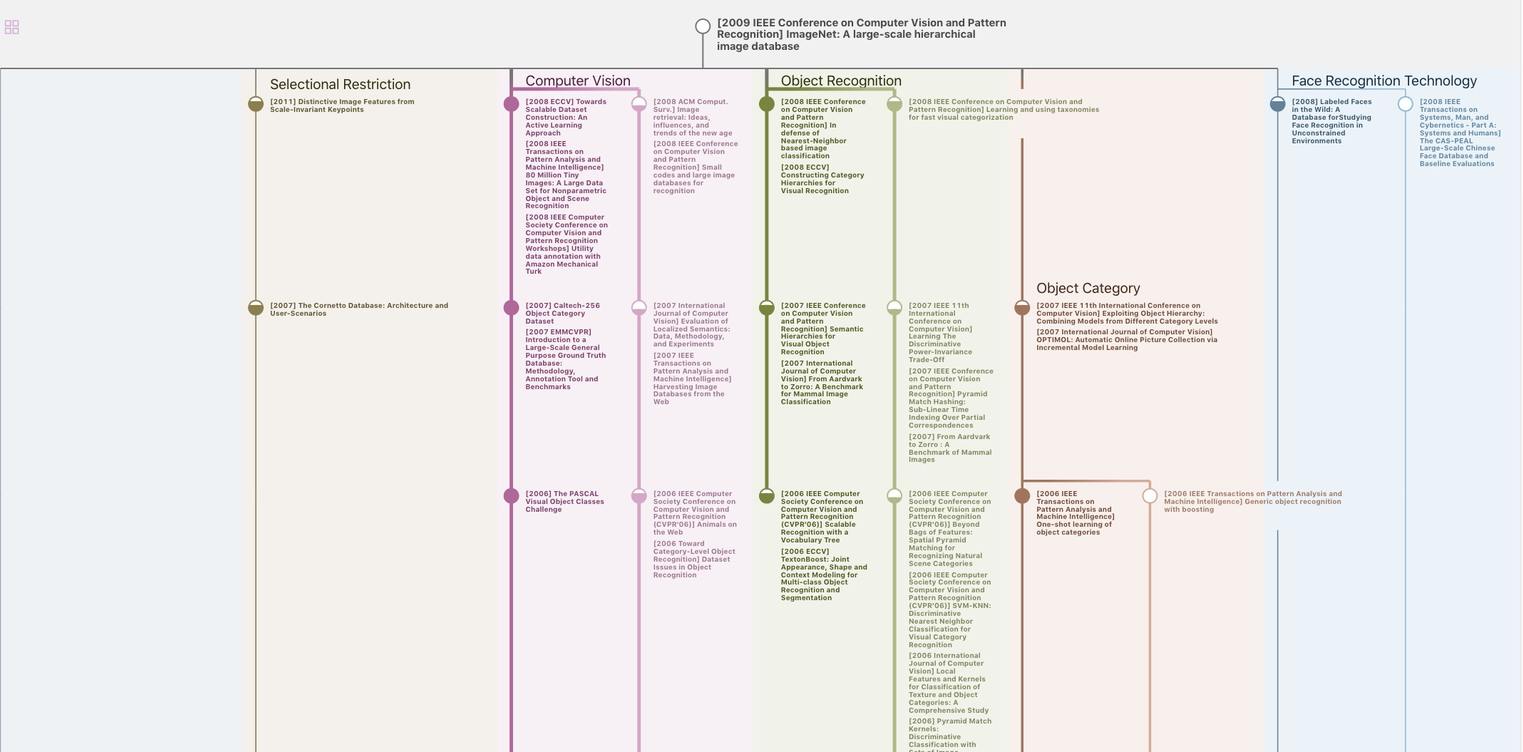
生成溯源树,研究论文发展脉络
Chat Paper
正在生成论文摘要