Antilogic, a new supervised machine learning software for the automatic interpretation of antibiotic susceptibility testing in clinical microbiology: proof-of-concept on three frequently isolated bacterial species.
Clinical microbiology and infection : the official publication of the European Society of Clinical Microbiology and Infectious Diseases(2022)
摘要
OBJECTIVE:Antibiotic susceptibility testing (AST) is necessary in order to adjust empirical antibiotic treatment, but the interpretation of results requires experience and knowledge. We have developed a machine learning software that is capable of reading AST images without any human intervention and that automatically interprets the AST, based on a database of antibiograms that have been clinically validated with European Committee on Antimicrobial Susceptibility Testing rules.
METHODS:We built a database of antibiograms that were labelled by senior microbiologists for three species: Escherichia coli, Klebsiella pneumoniae, and Staphylococcus aureus. We then developed Antilogic, a Python software based on an original image segmentation module and supervised learning models that we trained against the database. Finally, we blind tested Antilogic against a validation set of 5100 photos of antibiograms.
RESULTS:We trained Antilogic against a database of 18072 pictures of antibiograms. Overall agreement against the validation set reached 97% (16 855/17 281) regarding phenotypes. The severity rate of errors was also evaluated: 1.66% (287/17 281) were major errors and 0.80% (136/17 281) were very major errors. After implementation of uncertainty quantifications, the rate of errors decreased to 0.80% (114/13 451) and 0.42% (51/13 451) for major and very major errors respectively.
DISCUSSION:Antilogic is the first machine learning software that has been developed for AST interpretation. It is based on a novel approach that differs from the typical diameter measurement and expert system approach. Antilogic is a proof of concept that artificial intelligence can contribute to faster and easier diagnostic methods in the field of clinical microbiology.
更多查看译文
AI 理解论文
溯源树
样例
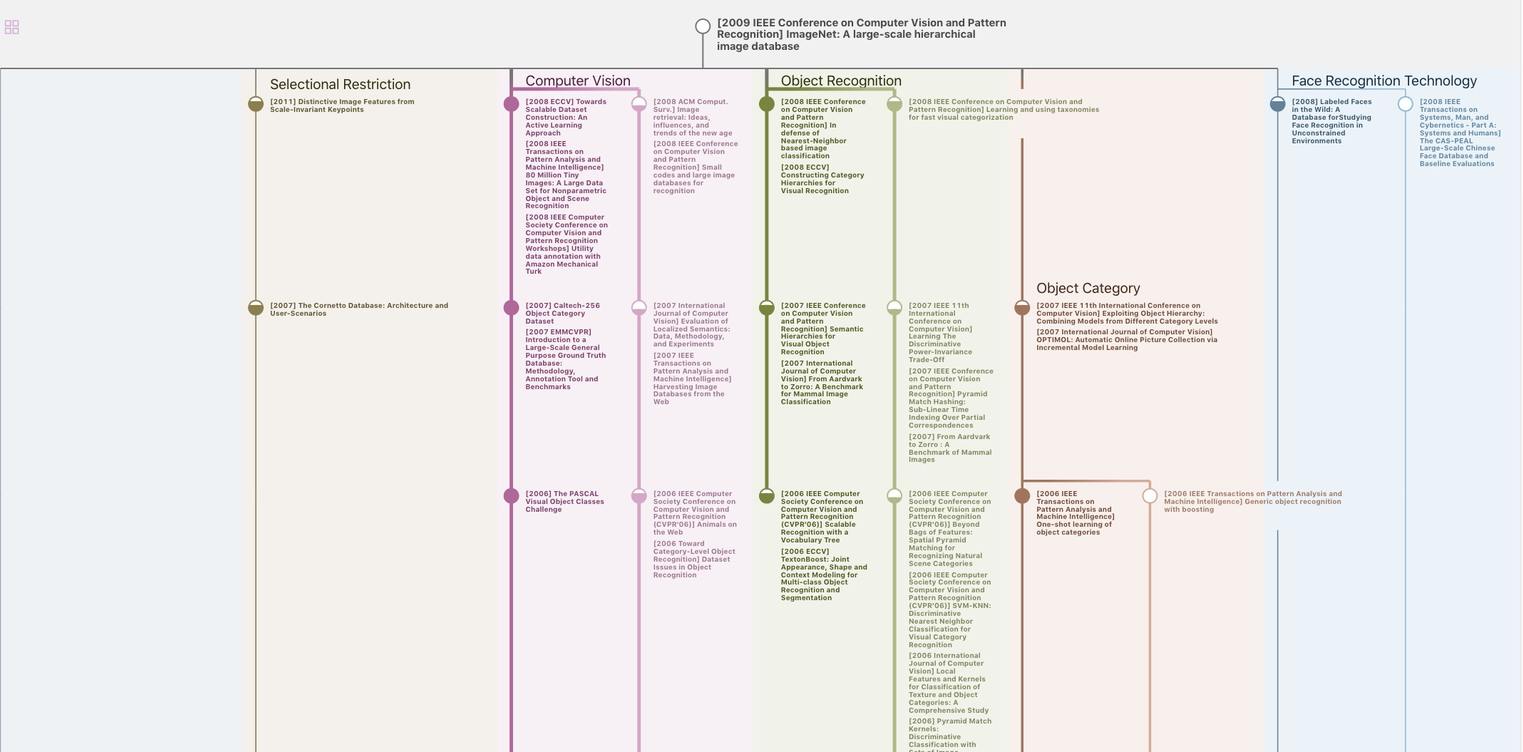
生成溯源树,研究论文发展脉络
Chat Paper
正在生成论文摘要