Efficient and accurate atomistic modeling of dopant migration using deep neural network
Materials Science in Semiconductor Processing(2022)
摘要
This paper proposes an efficient and accurate method to model atomistic dopant migration, by leveraging the emerging deep neural network (DNN). By performing nudged elastic band (NEB) simulations of three prototype systems (B-doped Si, Li-doped Si, and C-doped GaN), it is shown that the proposed DNN-based method runs about 104-105 times faster than the widely-used atomistic dopant migration modeling method based on density functional theory (DFT), meanwhile keeping DFT-level high accuracy. Active learning is used to reduce training set redundancy, and the DNN model is further optimized for more accurate NEB calculation. As a result, the dopant atomic position in saddle-point and the dopant migration energy barrier in the migration energy path (MEP) predicted by the proposed DNN-based NEB deviate merely about 10−2 Å and 10−2 eV, respectively, from those predicted by the established DFT-based NEB. Given its efficiency and accuracy, the proposed DNN-based method might be useful to develop future-generation atomic-scale technology computer-aided design (TCAD) tools.
更多查看译文
关键词
Deep neural network,Nudged elastic band,Ab-initio TCAD,Dopant,Density functional theory
AI 理解论文
溯源树
样例
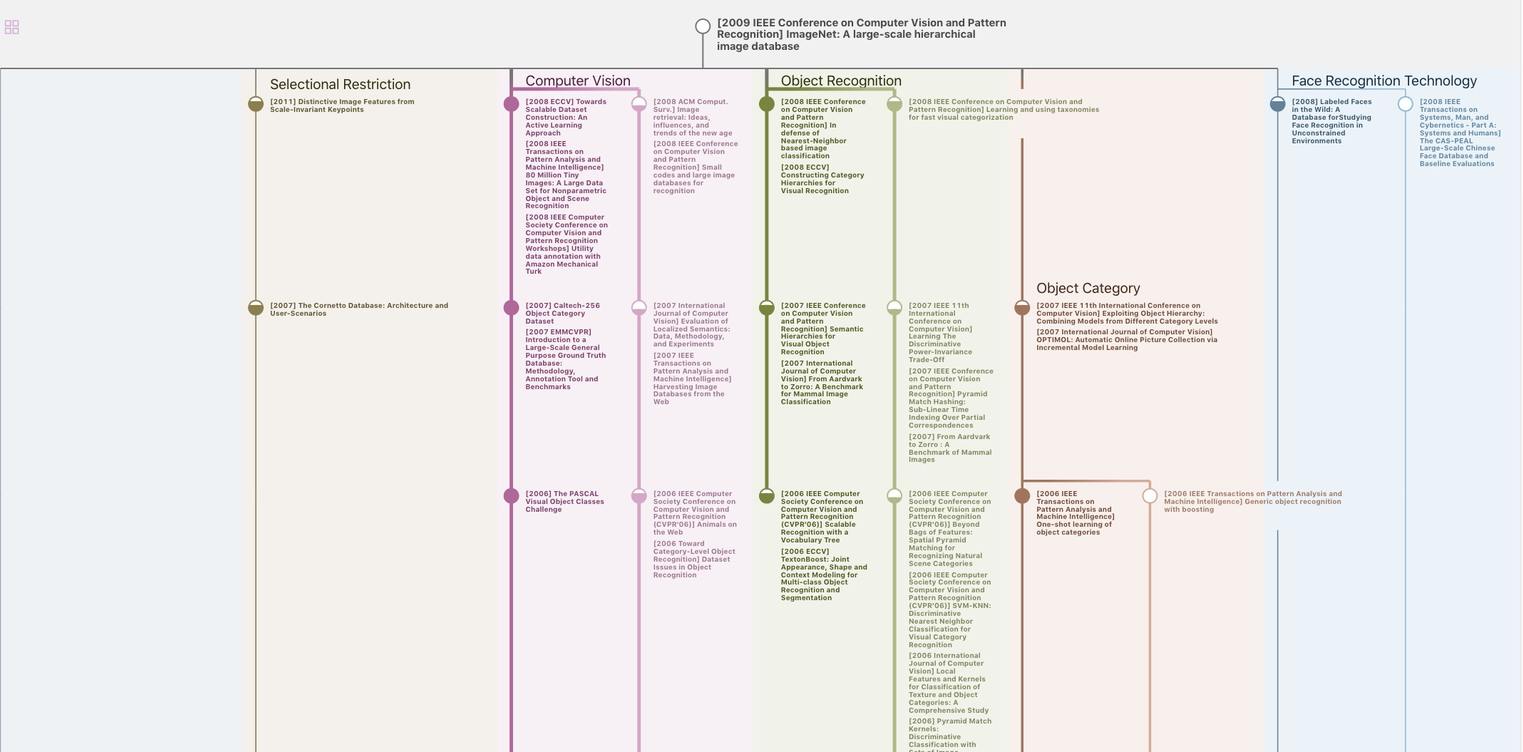
生成溯源树,研究论文发展脉络
Chat Paper
正在生成论文摘要