Attention Guided Global Enhancement and Local Refinement Network for Semantic Segmentation
IEEE TRANSACTIONS ON IMAGE PROCESSING(2022)
摘要
The encoder-decoder architecture is widely used as a lightweight semantic segmentation network. However, it struggles with a limited performance compared to a well-designed Dilated-FCN model for two major problems. First, commonly used upsampling methods in the decoder such as interpolation and deconvolution suffer from a local receptive field, unable to encode global contexts. Second, low-level features may bring noises to the network decoder through skip connections for the inadequacy of semantic concepts in early encoder layers. To tackle these challenges, a Global Enhancement Method is proposed to aggregate global information from high-level feature maps and adaptively distribute them to different decoder layers, alleviating the shortage of global contexts in the upsampling process. Besides, aLocal Refinement Module is developed by utilizing the decoder features as the semantic guidance to refine the noisy encoder features before the fusion of these two (the decoder features and the encoder features). Then, the two methods are integrated into a Context Fusion Block, and based on that, a novel Attention guided Global enhancement and Local refinement Network (AGLN) is elaborately designed. Extensive experiments on PASCAL Context, ADE20K, and PASCAL VOC 2012 datasets have demonstrated the effectiveness of the proposed approach. In particular, with a vanilla ResNet-101 backbone, AGLN achieves the state-of-the-art result (56.23% mean IOU) on the PASCAL Context dataset. The code is available at https://github.com/zhasen1996/AGLN.
更多查看译文
关键词
Semantics, Decoding, Image segmentation, Interpolation, Convolution, Aggregates, Context modeling, Semantic segmentation, encoder-decoder, global enhancement, local refinement, context fusion
AI 理解论文
溯源树
样例
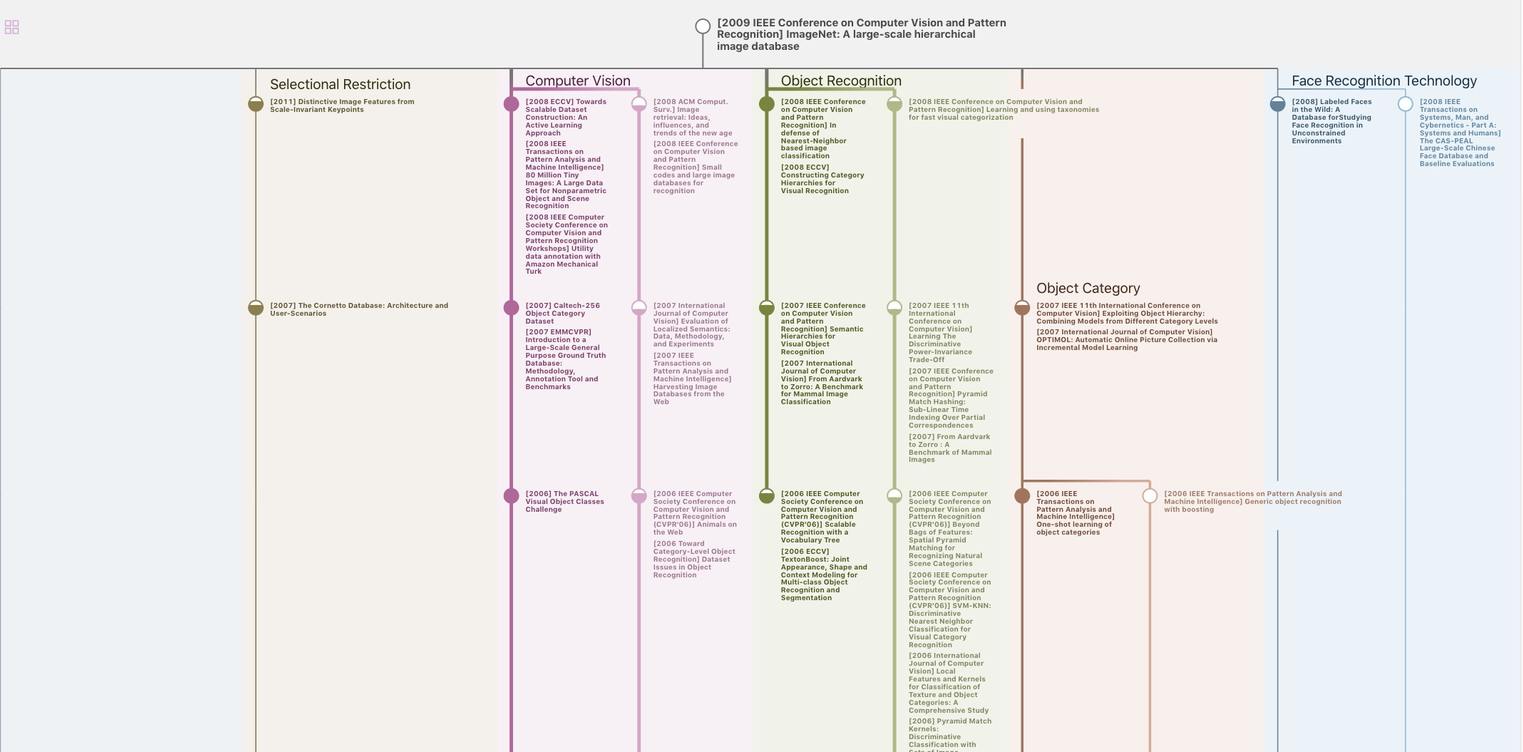
生成溯源树,研究论文发展脉络
Chat Paper
正在生成论文摘要