Segmenting across places: The need for fair transfer learning with satellite imagery
IEEE Conference on Computer Vision and Pattern Recognition(2022)
摘要
The increasing availability of high-resolution satellite imagery has enabled the use of machine learning to support land-cover measurement and inform policy-making. However, labelling satellite images is expensive and is available for only some locations. This prompts the use of transfer learning to adapt models from data-rich locations to others. Given the potential for high-impact applications of satellite imagery across geographies, a systematic assessment of transfer learning implications is warranted. In this work, we consider the task of land-cover segmentation and study the fairness implications of transferring models across locations. We leverage a large satellite image segmentation benchmark with 5987 images from 18 districts (9 urban and 9 rural). Via fairness metrics we quantify disparities in model performance along two axes – across urban-rural locations and across land-cover classes. Findings show that state-of-the-art models have better overall accuracy in rural areas compared to urban areas, through unsupervised domain adaptation methods transfer learning better to urban versus rural areas and enlarge fairness gaps. In analysis of reasons for these findings, we show that raw satellite images are overall more dissimilar between source and target districts for rural than for urban locations. This work highlights the need to conduct fairness analysis for satellite imagery segmentation models and motivates the development of methods for fair transfer learning in order not to introduce disparities between places, particularly urban and rural locations.
更多查看译文
关键词
fair transfer,high-resolution satellite imagery,machine learning,land-cover measurement,policy-making,labelling satellite images,data-rich locations,high-impact applications,transfer learning implications,land-cover segmentation,fairness implications,satellite image segmentation benchmark,via fairness metrics,urban-rural locations,land-cover classes,rural areas,urban areas,unsupervised domain adaptation methods transfer learning,fairness gaps,raw satellite images,urban locations,fairness analysis,satellite imagery segmentation models
AI 理解论文
溯源树
样例
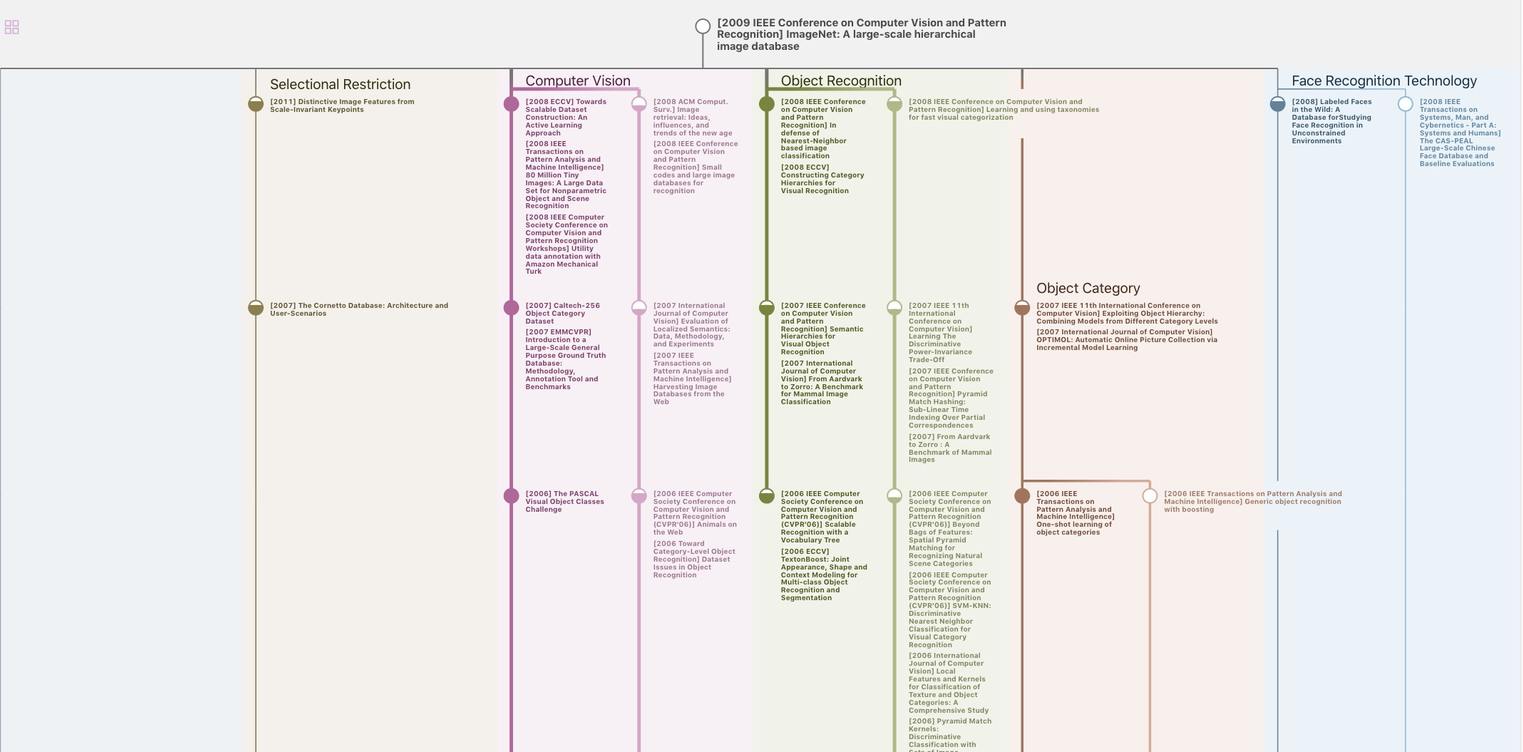
生成溯源树,研究论文发展脉络
Chat Paper
正在生成论文摘要