TANet: Thread-Aware Pretraining for Abstractive Conversational Summarization.
The Annual Conference of the North American Chapter of the Association for Computational Linguistics(2022)
摘要
Although pre-trained language models (PLMs) have achieved great success and become a milestone in NLP, abstractive conversational summarization remains a challenging but less studied task. The difficulty lies in two aspects. One is the lack of large-scale conversational summary data. Another is that applying the existing pre-trained models to this task is tricky because of the structural dependence within the conversation and its informal expression, etc. In this work, we first build a large-scale (11M) pretraining dataset called RCS, based on the multi-person discussions in the Reddit community. We then present TANet, a thread-aware Transformer-based network. Unlike the existing pre-trained models that treat a conversation as a sequence of sentences, we argue that the inherent contextual dependency among the utterances plays an essential role in understanding the entire conversation and thus propose two new techniques to incorporate the structural information into our model. The first is thread-aware attention which is computed by taking into account the contextual dependency within utterances. Second, we apply thread prediction loss to predict the relations between utterances. We evaluate our model on four datasets of real conversations, covering types of meeting transcripts, customer-service records, and forum threads. Experimental results demonstrate that TANET achieves a new state-of-the-art in terms of both automatic evaluation and human judgment.
更多查看译文
关键词
abstractive conversational
AI 理解论文
溯源树
样例
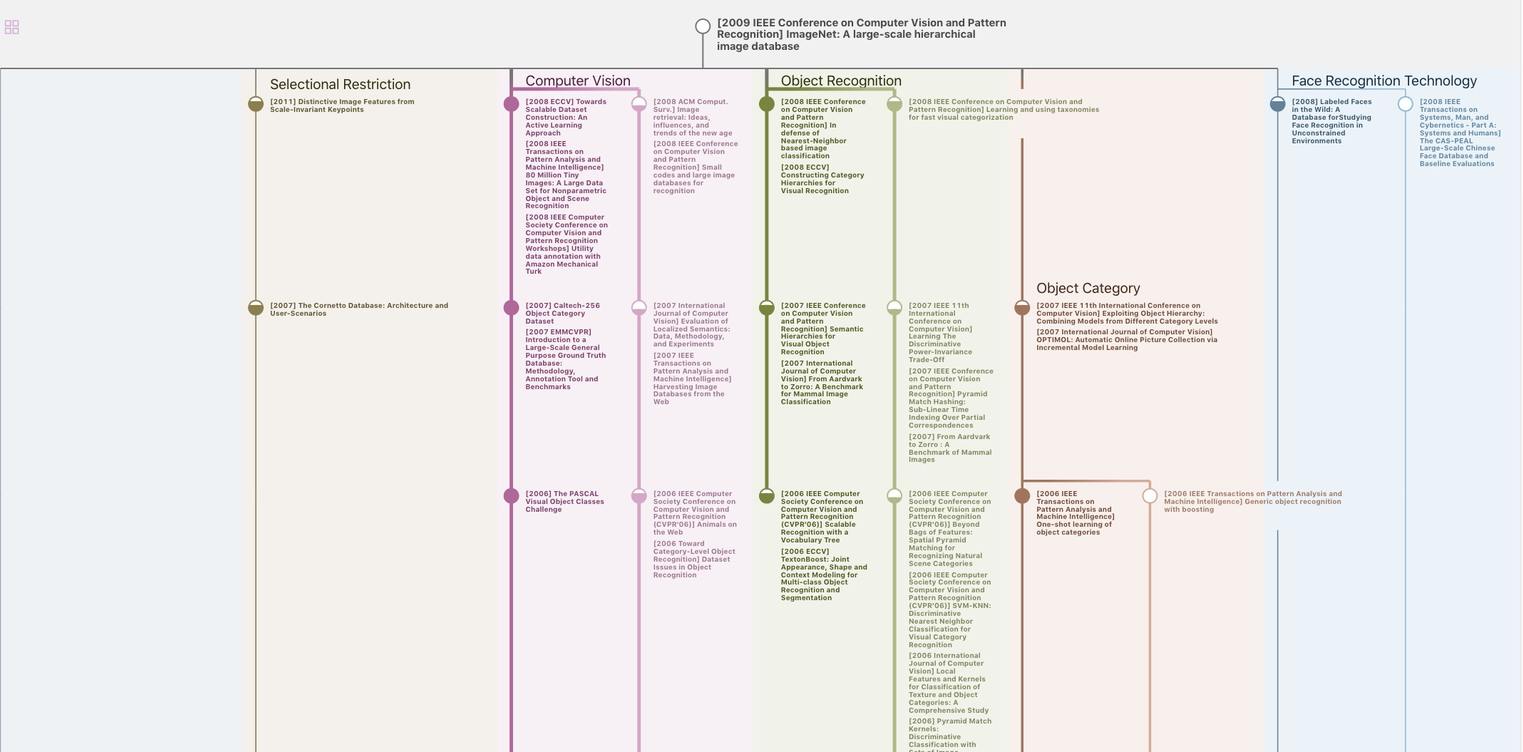
生成溯源树,研究论文发展脉络
Chat Paper
正在生成论文摘要