Deep Embeddings for Robust User-Based Amateur Vocal Percussion Classification
arxiv(2022)
摘要
Vocal Percussion Transcription (VPT) is concerned with the automatic detection and classification of vocal percussion sound events, allowing music creators and producers to sketch drum lines on the fly. Classifier algorithms in VPT systems learn best from small user-specific datasets, which usually restrict modelling to small input feature sets to avoid data overfitting. This study explores several deep supervised learning strategies to obtain informative feature sets for amateur vocal percussion classification. We evaluated the performance of these sets on regular vocal percussion classification tasks and compared them with several baseline approaches including feature selection methods and a speech recognition engine. These proposed learning models were supervised with several label sets containing information from four different levels of abstraction: instrument-level, syllable-level, phoneme-level, and boxeme-level. Results suggest that convolutional neural networks supervised with syllable-level annotations produced the most informative embeddings for classification, which can be used as input representations to fit classifiers with. Finally, we used back-propagation-based saliency maps to investigate the importance of different spectrogram regions for feature learning.
更多查看译文
关键词
classification,user-based
AI 理解论文
溯源树
样例
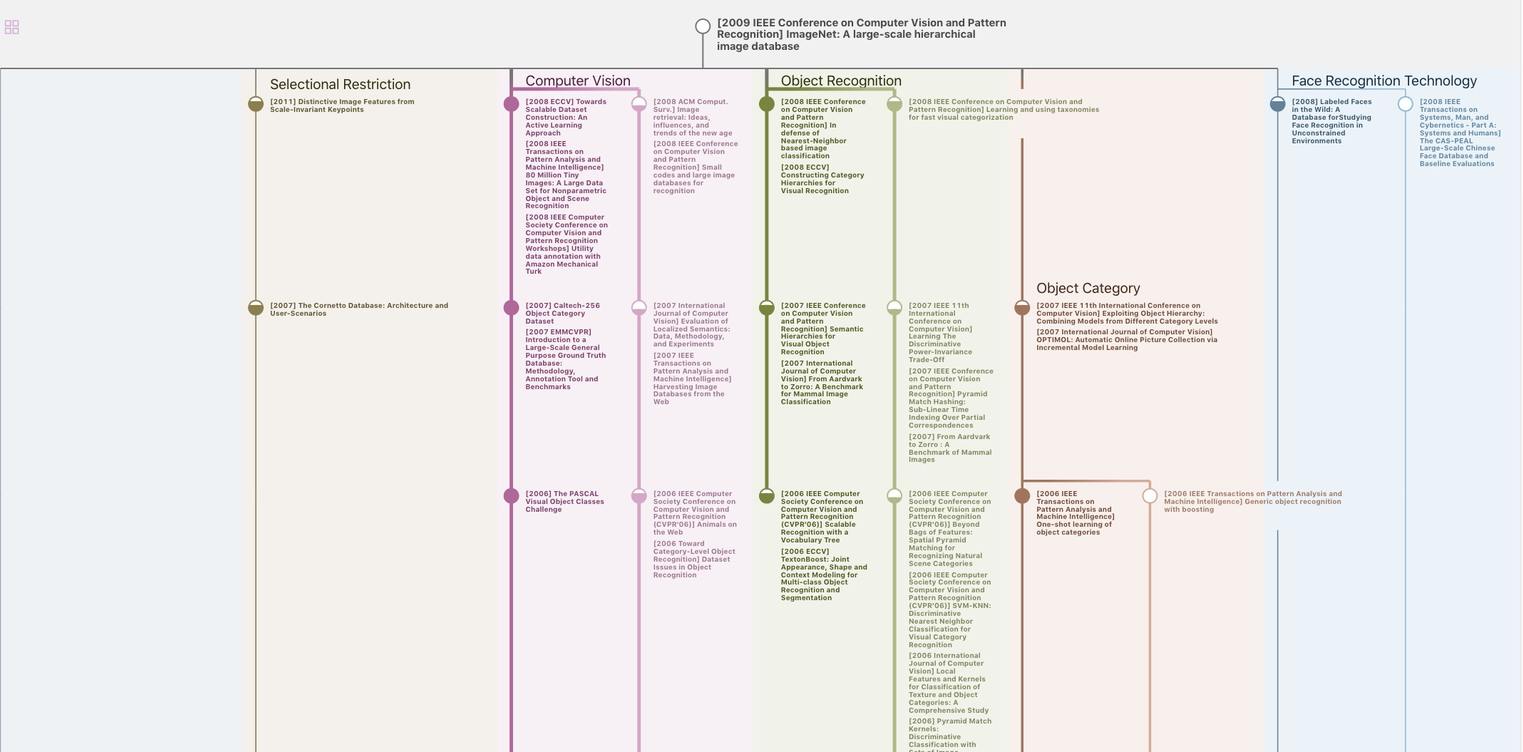
生成溯源树,研究论文发展脉络
Chat Paper
正在生成论文摘要