Negligible effect of brain MRI data preprocessing for tumor segmentation
arxiv(2023)
摘要
Magnetic resonance imaging (MRI) data is heterogeneous due to differences in device manufacturers, scanning protocols, and inter-subject variability. A conventional way to mitigate MR image heterogeneity is to apply preprocessing transformations such as anatomy alignment, voxel resampling, signal intensity equalization, image denoising, and localization of regions of interest. Although a preprocessing pipeline standardizes image appearance, its influence on the quality of image segmentation and on other downstream tasks in deep neural networks has never been rigorously studied. We conduct experiments on three publicly available datasets and evaluate the effect of different preprocessing steps in intra- and inter-dataset training scenarios. Our results demonstrate that most popular standardization steps add no value to the network performance; moreover, preprocessing can hamper model performance. We suggest that image intensity normalization approaches do not contribute to model accuracy because of the reduction of signal variance with image standardization. Finally, we show that the contribution of skull-stripping in data preprocessing is almost negligible if measured in terms of estimated tumor volume. We show that the only essential transformation for accurate deep learning analysis is the unification of voxel spacing across the dataset. In contrast, inter-subjects anatomy alignment in the form of non-rigid atlas registration is not necessary and intensity equalization steps (denoising, bias-field correction and histogram matching) do not improve models' performance. The study code is accessible online \footnote{https://github.com/MedImAIR/brain-mri-processing-pipeline}.
更多查看译文
关键词
brain mri data,tumor segmentation
AI 理解论文
溯源树
样例
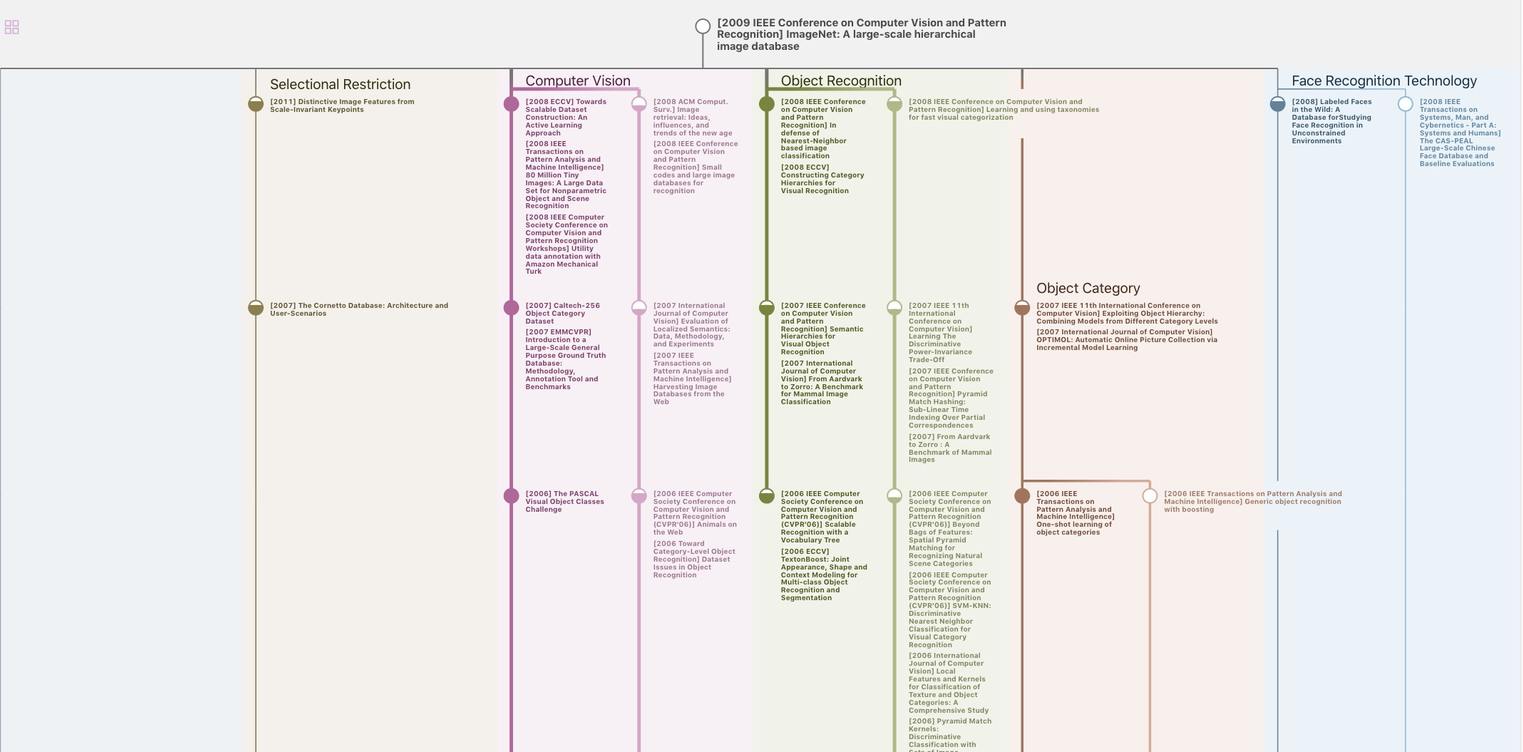
生成溯源树,研究论文发展脉络
Chat Paper
正在生成论文摘要