Modeling and contractivity of neural-synaptic networks with Hebbian learning
Automatica(2024)
摘要
This paper is concerned with the modeling and analysis of two of the most commonly used recurrent neural network models (i.e., Hopfield neural network and firing-rate neural network) with dynamic recurrent connections undergoing Hebbian learning rules. To capture the synaptic sparsity of neural circuits we propose a low dimensional formulation. We then characterize certain key dynamical properties. First, we give biologically-inspired forward invariance results. Then, we give sufficient conditions for the non-Euclidean contractivity of the models. Our contraction analysis leads to stability and robustness of time-varying trajectories — for networks with both excitatory and inhibitory synapses governed by both Hebbian and anti-Hebbian rules. For each model, we propose a contractivity test based upon biologically meaningful quantities, e.g., neural and synaptic decay rate, maximum in-degree, and the maximum synaptic strength. Then, we show that the models satisfy Dale’s Principle. Finally, we illustrate the effectiveness of our results via a numerical example.
更多查看译文
关键词
Nonlinear network systems,Hebbian/anti-Hebbian learning,Contraction theory
AI 理解论文
溯源树
样例
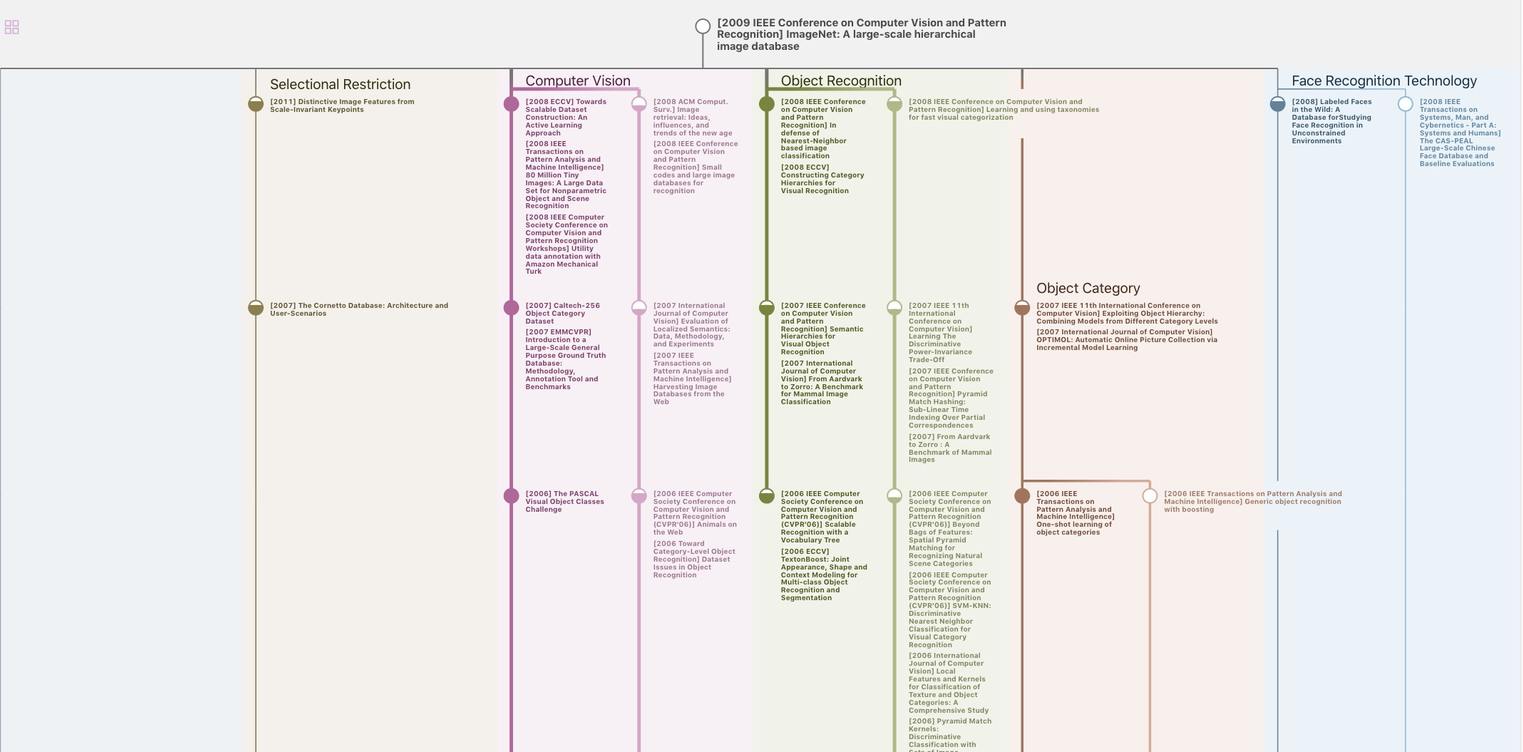
生成溯源树,研究论文发展脉络
Chat Paper
正在生成论文摘要