Bayesian optimization with experimental failure for high-throughput materials growth
NPJ COMPUTATIONAL MATERIALS(2022)
摘要
A crucial problem in achieving innovative high-throughput materials growth with machine learning, such as Bayesian optimization (BO), and automation techniques has been a lack of an appropriate way to handle missing data due to experimental failures. Here, we propose a BO algorithm that complements the missing data in optimizing materials growth parameters. The proposed method provides a flexible optimization algorithm that searches a wide multi-dimensional parameter space. We demonstrate the effectiveness of the method with simulated data as well as in its implementation for actual materials growth, namely machine-learning-assisted molecular beam epitaxy (ML-MBE) of SrRuO 3 , which is widely used as a metallic electrode in oxide electronics. Through the exploitation and exploration in a wide three-dimensional parameter space, while complementing the missing data, we attained tensile-strained SrRuO 3 film with a high residual resistivity ratio of 80.1, the highest among tensile-strained SrRuO 3 films ever reported, in only 35 MBE growth runs.
更多查看译文
关键词
Computational methods,Electronic properties and materials,Materials Science,general,Characterization and Evaluation of Materials,Mathematical and Computational Engineering,Theoretical,Mathematical and Computational Physics,Computational Intelligence,Mathematical Modeling and Industrial Mathematics
AI 理解论文
溯源树
样例
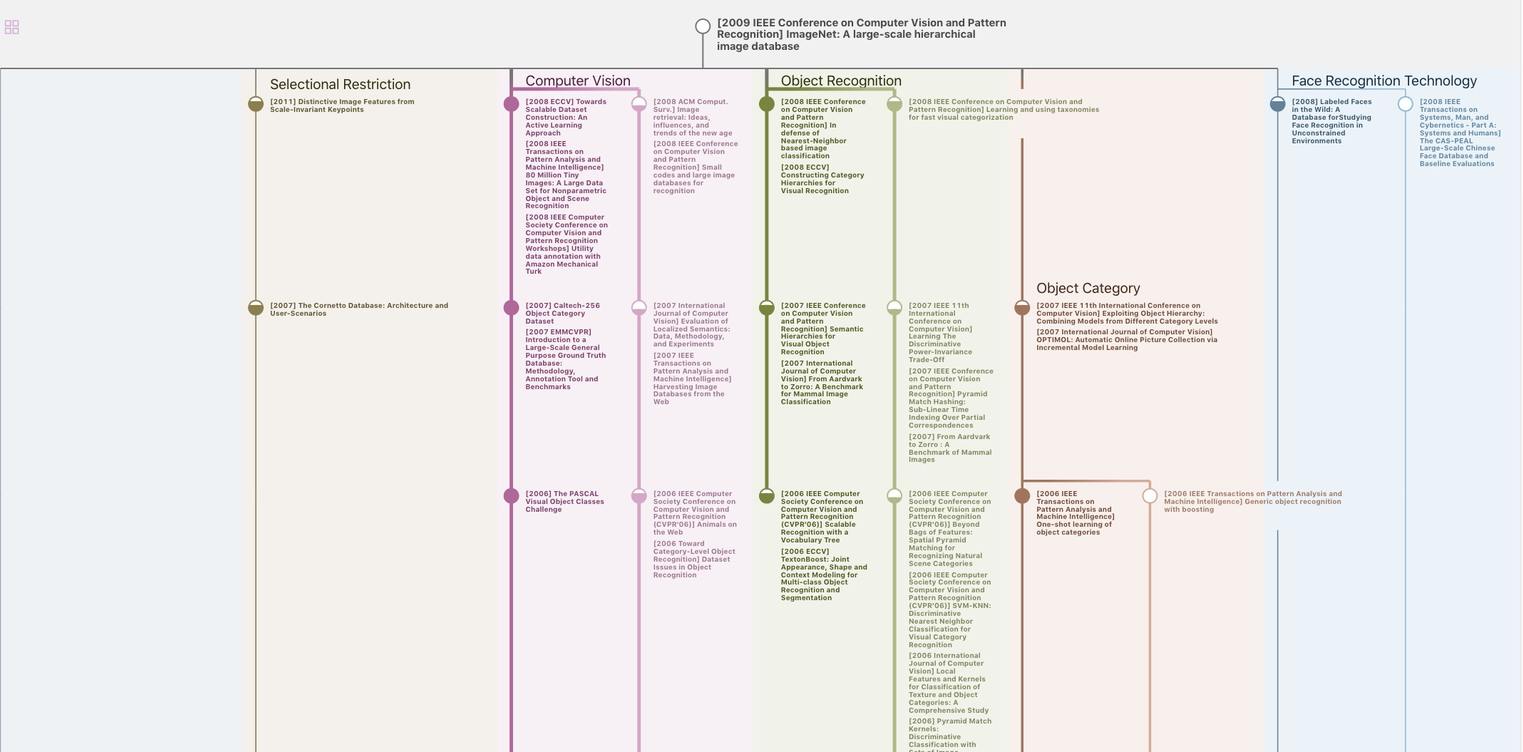
生成溯源树,研究论文发展脉络
Chat Paper
正在生成论文摘要