Machine Learning for Plant Stress Modeling: A Perspective towards Hormesis Management
PLANTS-BASEL(2022)
摘要
Plant stress is one of the most significant factors affecting plant fitness and, consequently, food production. However, plant stress may also be profitable since it behaves hormetically; at low doses, it stimulates positive traits in crops, such as the synthesis of specialized metabolites and additional stress tolerance. The controlled exposure of crops to low doses of stressors is therefore called hormesis management, and it is a promising method to increase crop productivity and quality. Nevertheless, hormesis management has severe limitations derived from the complexity of plant physiological responses to stress. Many technological advances assist plant stress science in overcoming such limitations, which results in extensive datasets originating from the multiple layers of the plant defensive response. For that reason, artificial intelligence tools, particularly Machine Learning (ML) and Deep Learning (DL), have become crucial for processing and interpreting data to accurately model plant stress responses such as genomic variation, gene and protein expression, and metabolite biosynthesis. In this review, we discuss the most recent ML and DL applications in plant stress science, focusing on their potential for improving the development of hormesis management protocols.
更多查看译文
关键词
eustress, crop improvement, intelligent algorithms, agricultural engineering
AI 理解论文
溯源树
样例
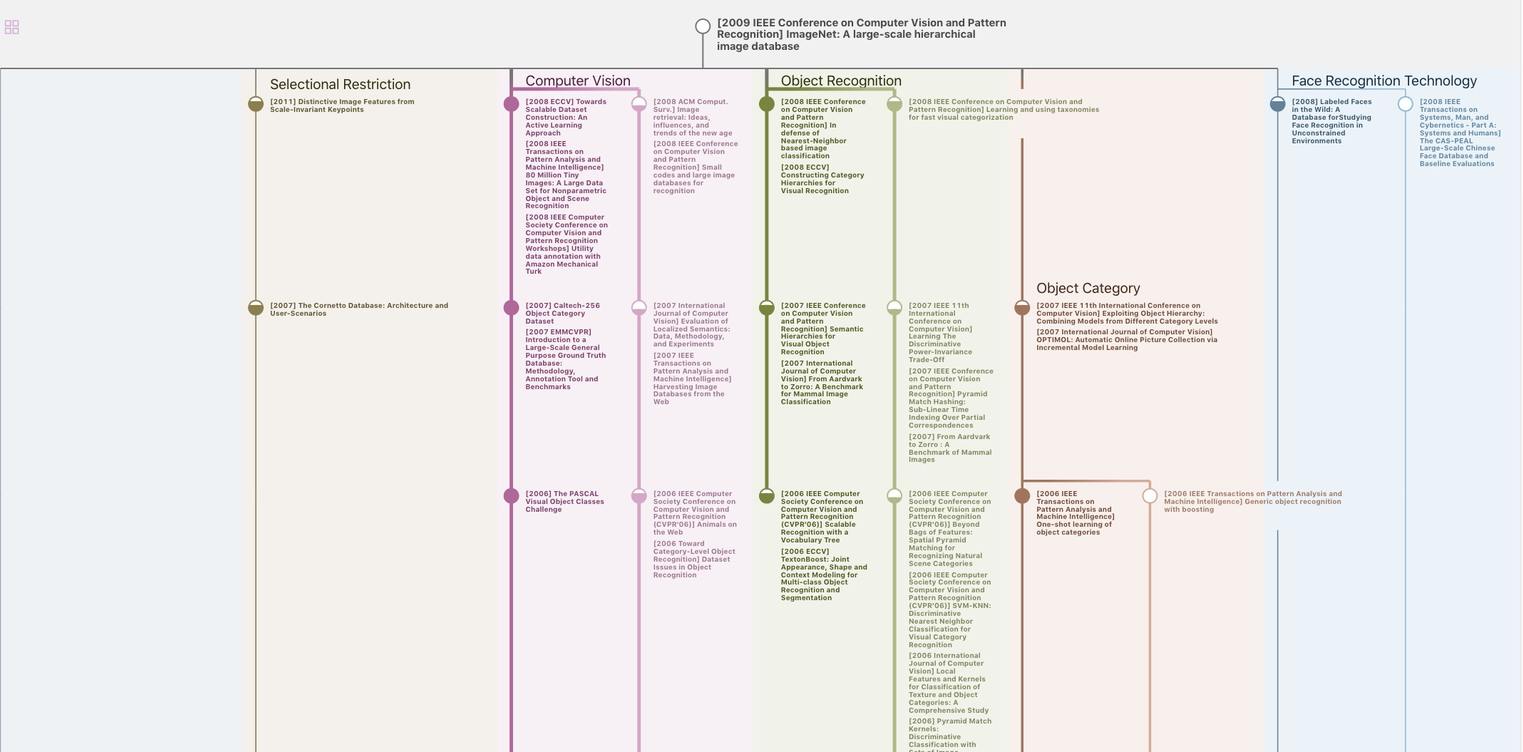
生成溯源树,研究论文发展脉络
Chat Paper
正在生成论文摘要