A Differential Privacy Strategy Based on Local Features of Non-Gaussian Noise in Federated Learning.
SENSORS(2022)
摘要
As an emerging artificial intelligence technology, federated learning plays a significant role in privacy preservation in machine learning, although its main objective is to prevent peers from peeping data. However, attackers from the outside can steal metadata in transit and through data reconstruction or other techniques to obtain the original data, which poses a great threat to the security of the federated learning system. In this paper, we propose a differential privacy strategy including encryption and decryption methods based on local features of non-Gaussian noise, which aggregates the noisy metadata through a sequential Kalman filter in federated learning scenarios to increase the reliability of the federated learning method. We name the local features of non-Gaussian noise as the non-Gaussian noise fragments. Compared with the traditional methods, the proposed method shows stronger security performance for two reasons. Firstly, non-Gaussian noise fragments contain more complex statistics, making them more difficult for attackers to identify. Secondly, in order to obtain accurate statistical features, attackers must aggregate all of the noise fragments, which is very difficult due to the increasing number of clients. We conduct experiments that demonstrate that the proposed method can greatly enhanced the system's security.
更多查看译文
关键词
federated learning (FL), differential privacy, Kalman filter, non-Gaussian noise
AI 理解论文
溯源树
样例
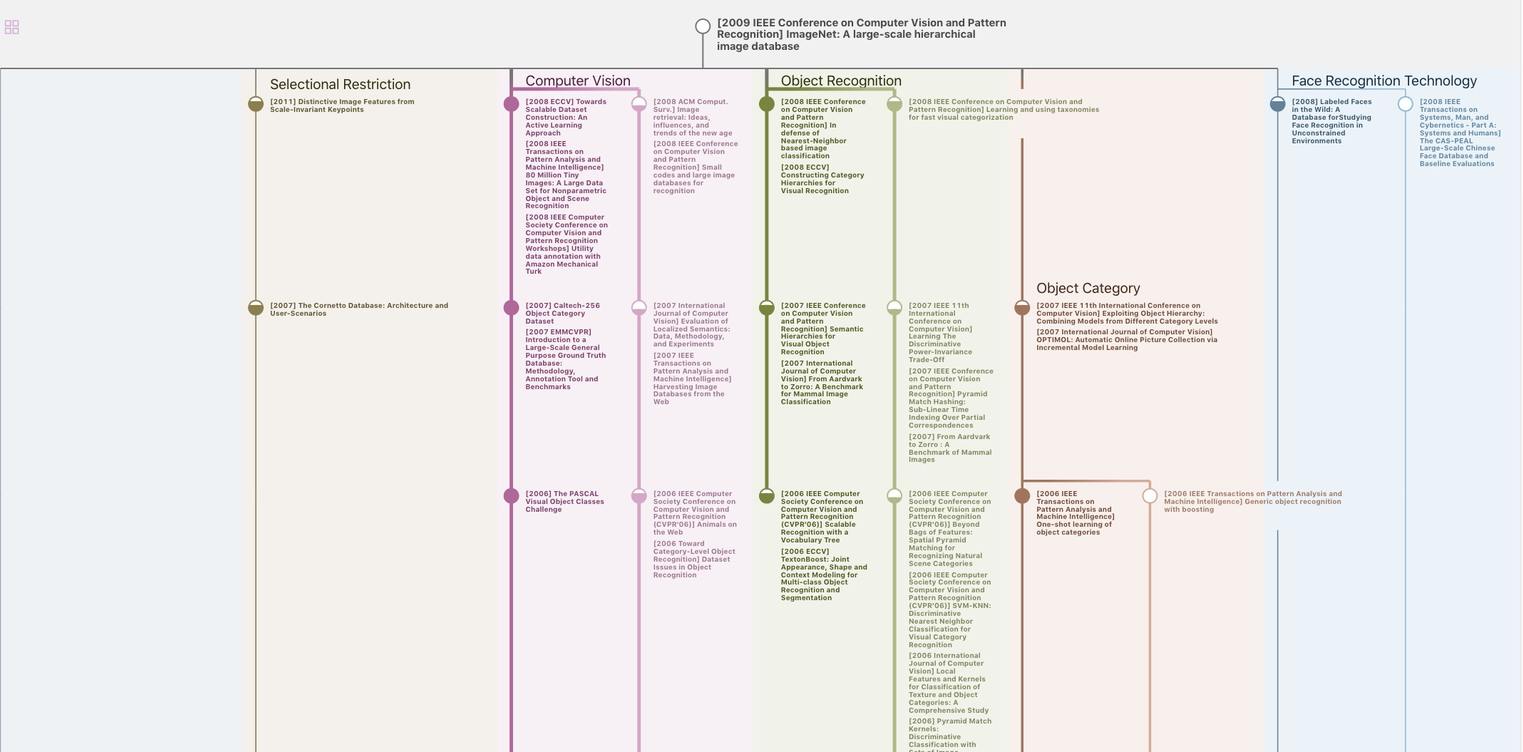
生成溯源树,研究论文发展脉络
Chat Paper
正在生成论文摘要