Defensive Patches for Robust Recognition in the Physical World
IEEE Conference on Computer Vision and Pattern Recognition(2022)
摘要
To operate in real-world high-stakes environments, deep learning systems have to endure noises that have been con-tinuously thwarting their robustness. Data-end defense, which improves robustness by operations on input data in-stead of modifying models, has attracted intensive attention due to its feasibility in practice. However, previous data-end defenses show low generalization against diverse noises and weak transferability across multiple models. Motivated by the fact that robust recognition depends on both local and global features, we propose a defensive patch generation framework to address these problems by helping mod-els better exploit these features. For the generalization against diverse noises, we inject class-specific identifiable patterns into a confined local patch prior, so that defensive patches could preserve more recognizable features towards specific classes, leading models for better recognition under noises. For the transferability across multiple models, we guide the defensive patches to capture more global fea-ture correlations within a class, so that they could activate model-shared global perceptions and transfer better among models. Our defensive patches show great potentials to im-prove application robustness in practice by simply sticking them around target objects. Extensive experiments show that we outperform others by large margins (improve 20+ % accuracy for both adversarial and corruption robustness on average in the digital and physical world). 1 1 Our codes are available at https://github.com/nlsde-safety-team/DefensivePatch.
更多查看译文
关键词
Vision applications and systems, Adversarial attack and defense, Computer vision for social good
AI 理解论文
溯源树
样例
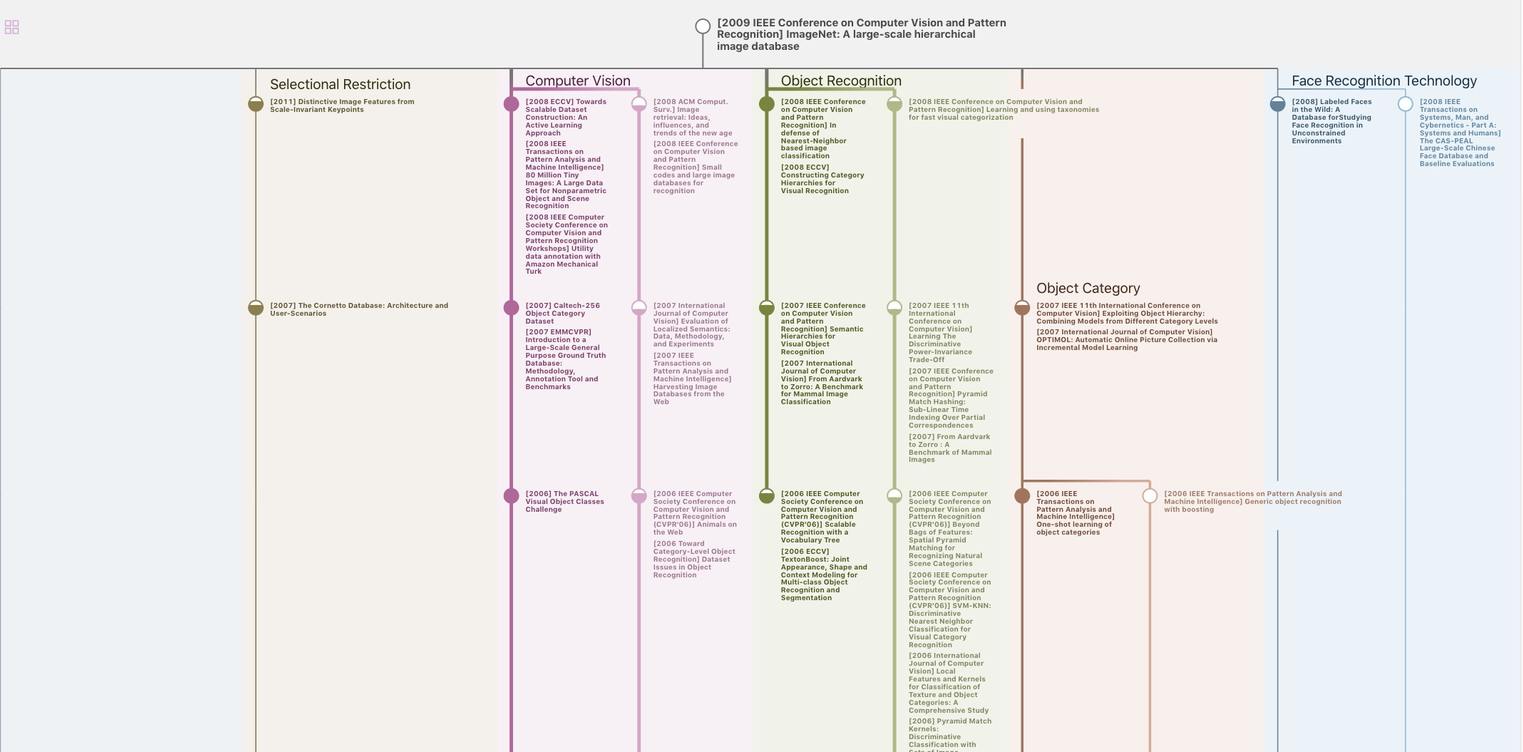
生成溯源树,研究论文发展脉络
Chat Paper
正在生成论文摘要