Stability Regularization for Discrete Representation Learning
International Conference on Learning Representations (ICLR)(2022)
摘要
We present a method for training neural network models with discrete stochastic variables.
The core of the method is \emph{stability regularization}, which is a regularization procedure based on the idea of noise stability developed in Gaussian isoperimetric theory in the analysis of Gaussian functions.
Stability regularization is method to make the output of continuous functions of Gaussian random variables close to discrete, that is binary or categorical, without the need for significant manual tuning.
The method allows control over the extent to which a Gaussian function's output is close to discrete, thus allowing for continued flow of gradient.
The method can be used standalone or in combination with existing continuous relaxation methods.
We validate the method in a broad range of experiments using discrete variables including neural relational inference, generative modeling, clustering and conditional computing.
更多查看译文
AI 理解论文
溯源树
样例
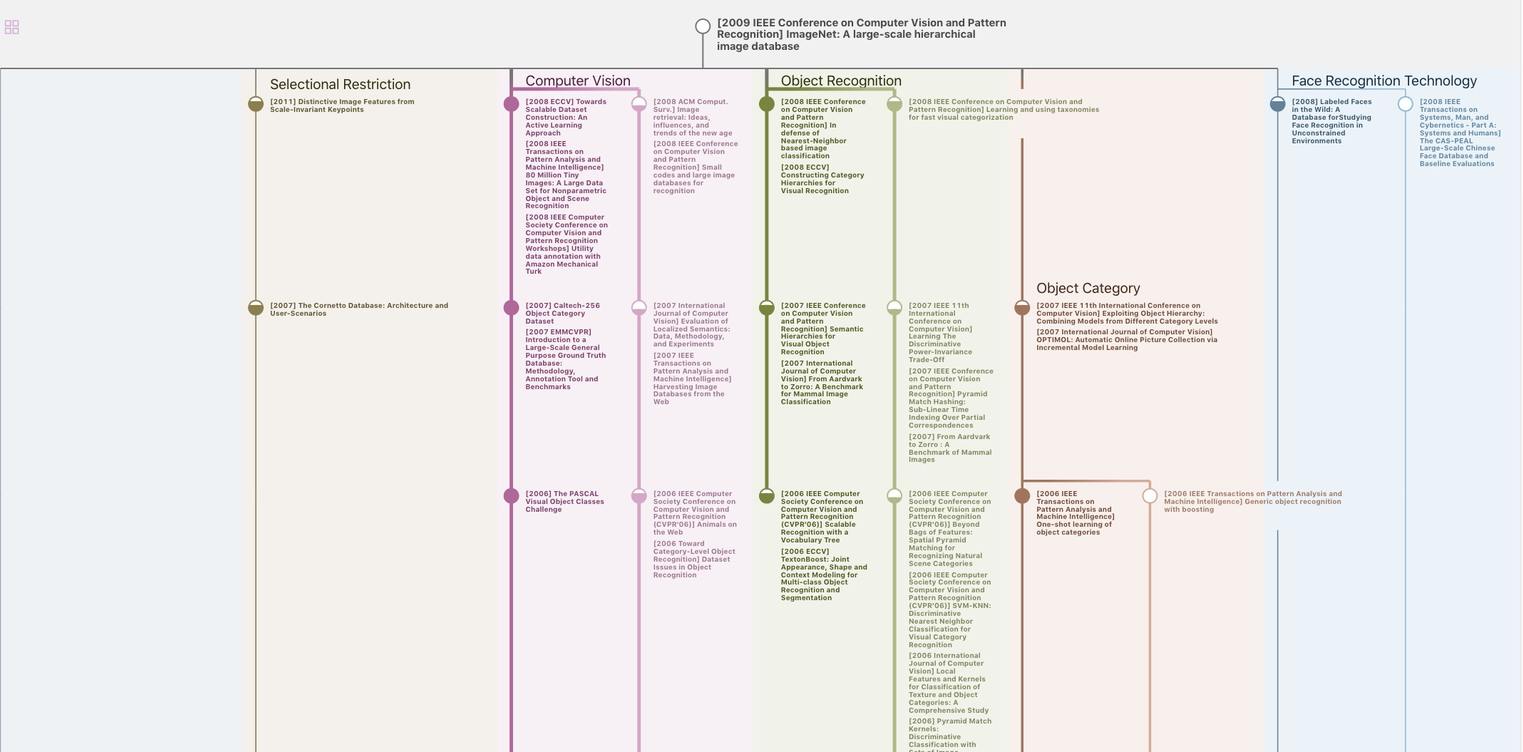
生成溯源树,研究论文发展脉络
Chat Paper
正在生成论文摘要