Deep Attentive Variational Inference
International Conference on Learning Representations (ICLR)(2022)
摘要
Stochastic Variational Inference is a powerful framework for learning large-scale probabilistic latent variable models. However, typical assumptions on the factorization or independence of the latent variables can substantially restrict its capacity for inference and generative modeling. A major line of active research aims at building more expressive variational models by designing deep hierarchies of interdependent latent variables. Although these models exhibit superior performance and enable richer latent representations, we show that they incur diminishing returns: adding more stochastic layers to an already very deep model yields small predictive improvement while substantially increasing the inference and training time. Moreover, the architecture for this class of models favors local interactions among the latent variables between neighboring layers when designing the conditioning factors of the involved distributions. This is the first work that proposes attention mechanisms to build more expressive variational distributions in deep probabilistic models by explicitly modeling both local and global interactions in the latent space. Specifically, we propose deep attentive variational autoencoder and test it on a variety of established datasets. We show it achieves state-of-the-art log-likelihoods while using fewer latent layers and requiring less training time than existing models. The proposed non-local inference reduces computational footprint by alleviating the need for deep hierarchies.
更多查看译文
关键词
variational inference,approximate inference,deep probabilistic models,deep probabilistic learning,variational autoencoder,probabilistic methods for deep learning,attention
AI 理解论文
溯源树
样例
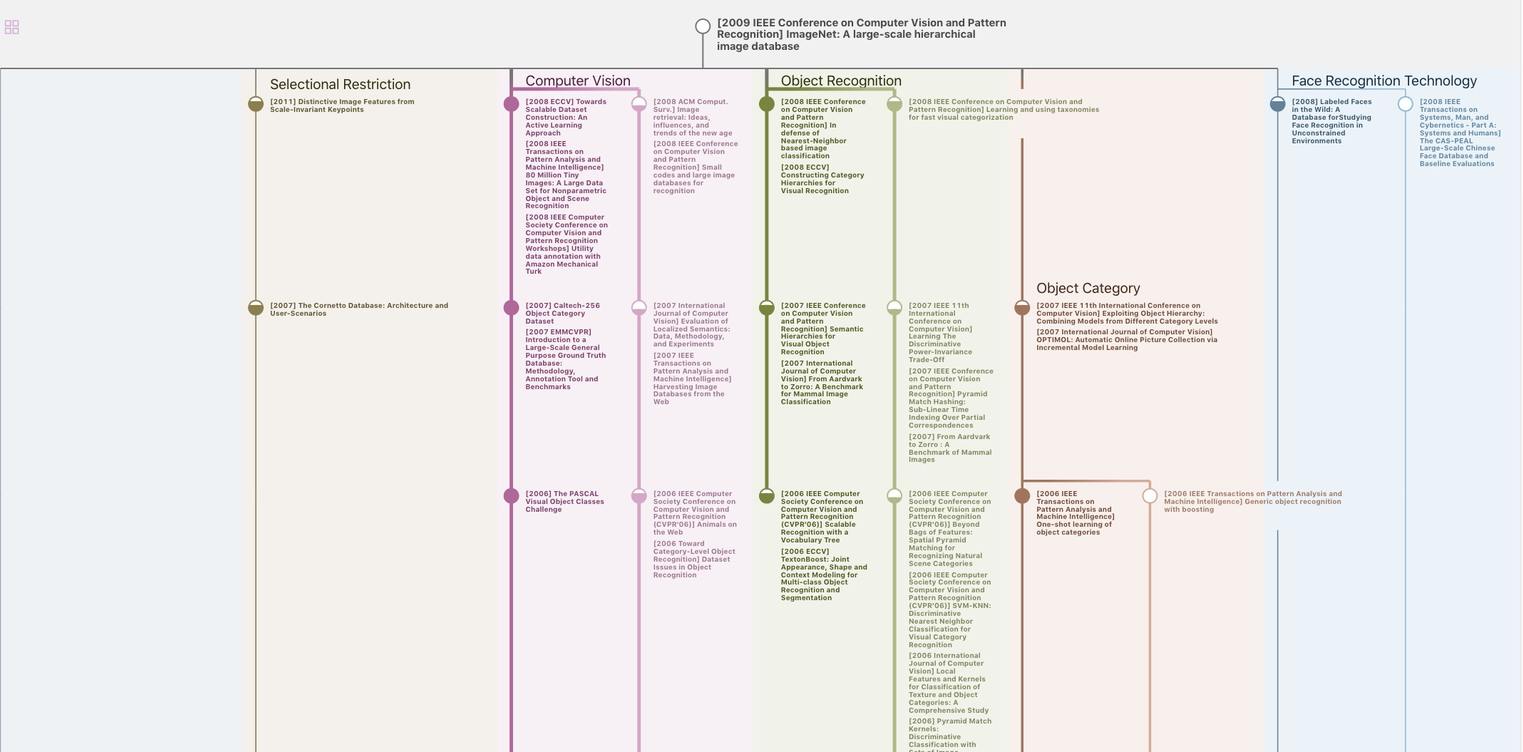
生成溯源树,研究论文发展脉络
Chat Paper
正在生成论文摘要