Understanding approximate and unrolled dictionary learning for pattern recovery
International Conference on Learning Representations (ICLR)(2022)
摘要
Dictionary learning consists of finding a sparse representation from noisy data and is a common way to encode data-driven prior knowledge on signals. Alternating minimization (AM) is standard for the underlying optimization, where gradient descent steps alternate with sparse coding procedures. The major drawback of this method is its prohibitive computational cost, making it unpractical on large real-world data sets. This work studies an approximate formulation of dictionary learning based on unrolling and compares it to alternating minimization to find the best trade-off between speed and precision. We analyze the asymptotic behavior and convergence rate of gradients estimates in both methods. We show that unrolling performs better on the support of the inner problem solution and during the first iterations. Finally, we apply unrolling on pattern learning in magnetoencephalography (MEG) with the help of a stochastic algorithm and compare the performance to a state-of-the-art method.
更多查看译文
关键词
Dictionary learning,bi-level optimization,unrolling,pattern learning
AI 理解论文
溯源树
样例
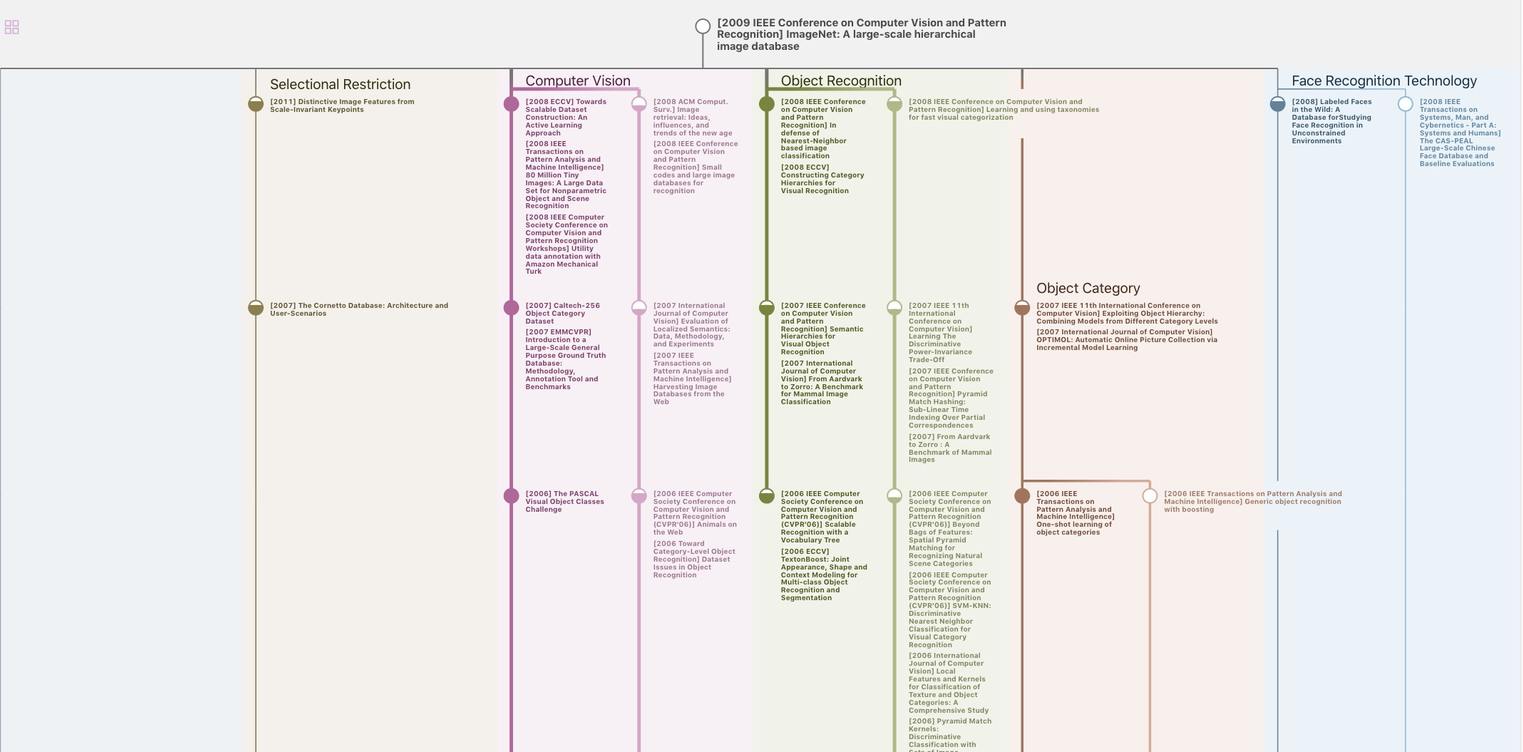
生成溯源树,研究论文发展脉络
Chat Paper
正在生成论文摘要