GAP: A Graph-aware Language Model Framework for Knowledge Graph-to-Text Generation.
International Conference on Computational Linguistics(2022)
摘要
Recent improvements in KG-to-text generation are due to additional auxiliary pre-training tasks designed to give the fine-tune task a boost in performance. These tasks require extensive computational resources while only suggesting marginal improvements. Here, we demonstrate that by fusing graph-aware elements into existing pre-trained language models, we are able to outperform state-of-the-art models and close the gap imposed by additional pre-training tasks. We do so by proposing a mask structure to capture neighborhood information and a novel type encoder that adds a bias to the graph-attention weights depending on the connection type. Experiments on two KG-to-text benchmark datasets show our models are competitive while involving fewer parameters and no additional pre-training tasks. By formulating the problem as a framework, we can interchange the various proposed components and begin interpreting KG-to-text generative models based on the topological and type information found in a graph.
更多查看译文
关键词
generation,language,graph-aware,graph-to-text
AI 理解论文
溯源树
样例
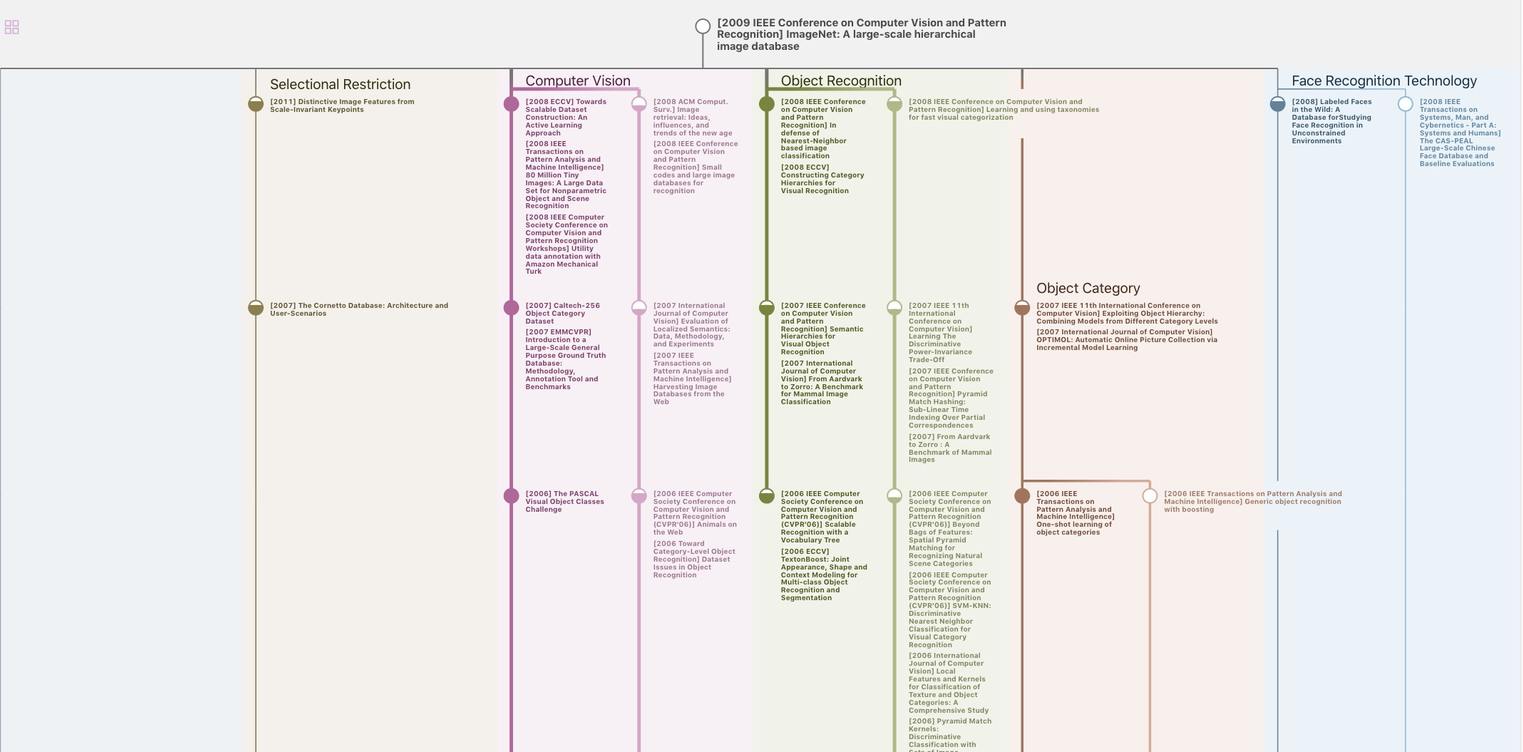
生成溯源树,研究论文发展脉络
Chat Paper
正在生成论文摘要