Designing experiments toward shrinkage estimation
ELECTRONIC JOURNAL OF STATISTICS(2023)
摘要
How can increasingly available observational data be used to improve the design of randomized controlled trials (RCTs)? We seek to de-sign a prospective RCT, with the intent of using an Empirical Bayes estimator to shrink the causal estimates from our trial toward causal estimates obtained from an observational study. We ask: how might we design the experiment to better complement the observational study in this setting?We show that the risk of such shrinkage estimators can be computed efficiently via numerical integration. We then propose three algorithms for determining the best allocation of units to strata given the estimator's plannned use: Neyman allocation; a "naive" design assuming no unmeasured confounding in the observational study; and a robust design accounting for the imperfect parameter estimates we would obtain from the observational study with unmeasured confounding. We propose guardrails on the designs, so that our experiment could be reasonably analyzed without shrinkage if desired.We demonstrate the viability of these experimental designs through a simulation study involving a rare, binary outcome. Lastly, we deploy our methods on real data from the Women's Health Initiative, a 1991 study estimating the health effects of hormone therapy on postmenopausal women. In particular, we determine how many units should be allocated to each treatment arm in each stratum of interest in order to maximally reduce estimation risk given the planned use of the shrinkage estimator. We find improved design provides further benefits over and above the benefit of the shrinkage estimator itself.
更多查看译文
关键词
Causal inference,experimental design,sensitivity analysis,empirical Bayes
AI 理解论文
溯源树
样例
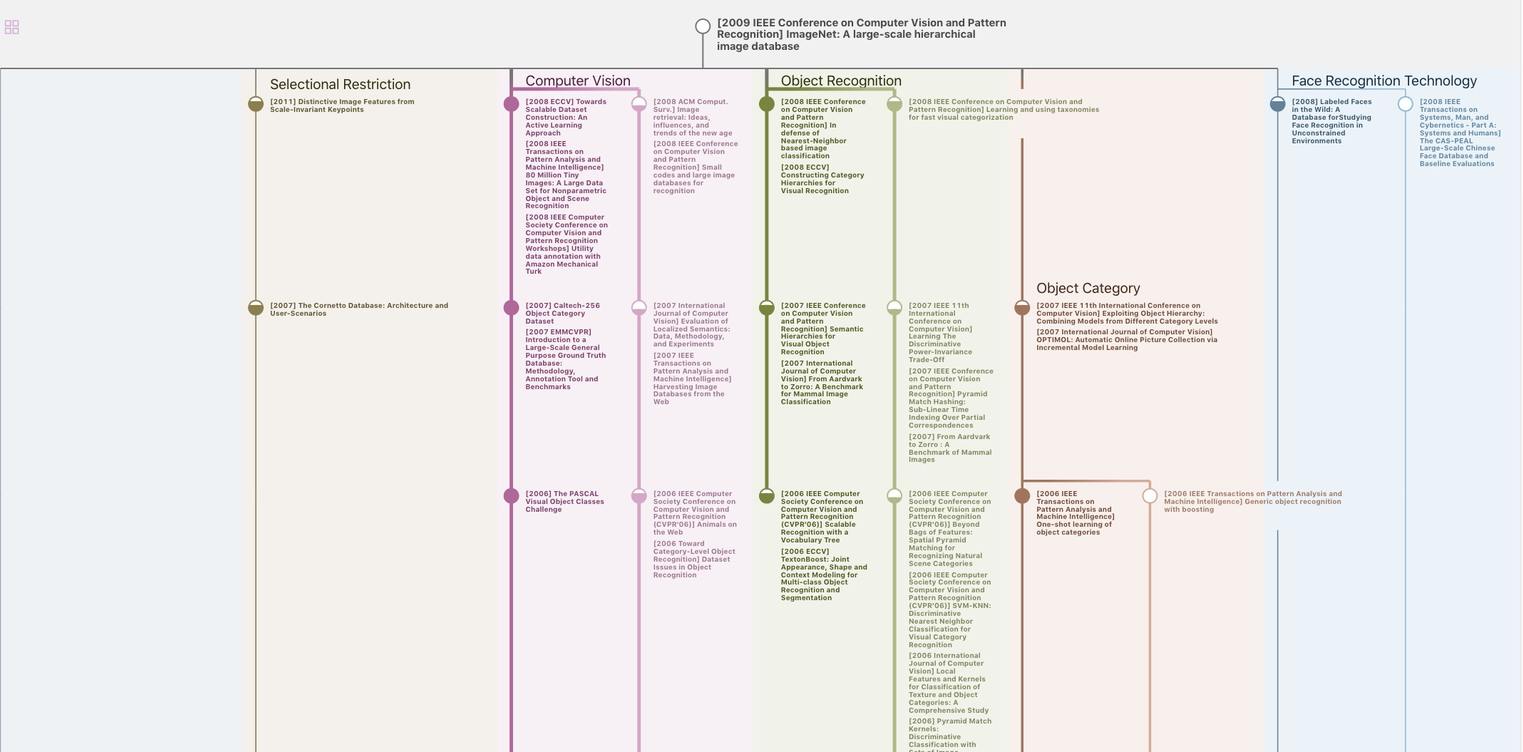
生成溯源树,研究论文发展脉络
Chat Paper
正在生成论文摘要