Sim-to-Real 6D Object Pose Estimation via Iterative Self-training for Robotic Bin-picking
arXiv (Cornell University)(2022)
摘要
In this paper, we propose an iterative self-training framework for sim-to-real 6D object pose estimation to facilitate cost-effective robotic grasping. Given a bin-picking scenario, we establish a photo-realistic simulator to synthesize abundant virtual data, and use this to train an initial pose estimation network. This network then takes the role of a teacher model, which generates pose predictions for unlabeled real data. With these predictions, we further design a comprehensive adaptive selection scheme to distinguish reliable results, and leverage them as pseudo labels to update a student model for pose estimation on real data. To continuously improve the quality of pseudo labels, we iterate the above steps by taking the trained student model as a new teacher and re-label real data using the refined teacher model. We evaluate our method on a public benchmark and our newly-released dataset, achieving an ADD(-S) improvement of 11.49% and 22.62% respectively. Our method is also able to improve robotic bin-picking success by 19.54%, demonstrating the potential of iterative sim-to-real solutions for robotic applications.
更多查看译文
关键词
object,sim-to-real,self-training
AI 理解论文
溯源树
样例
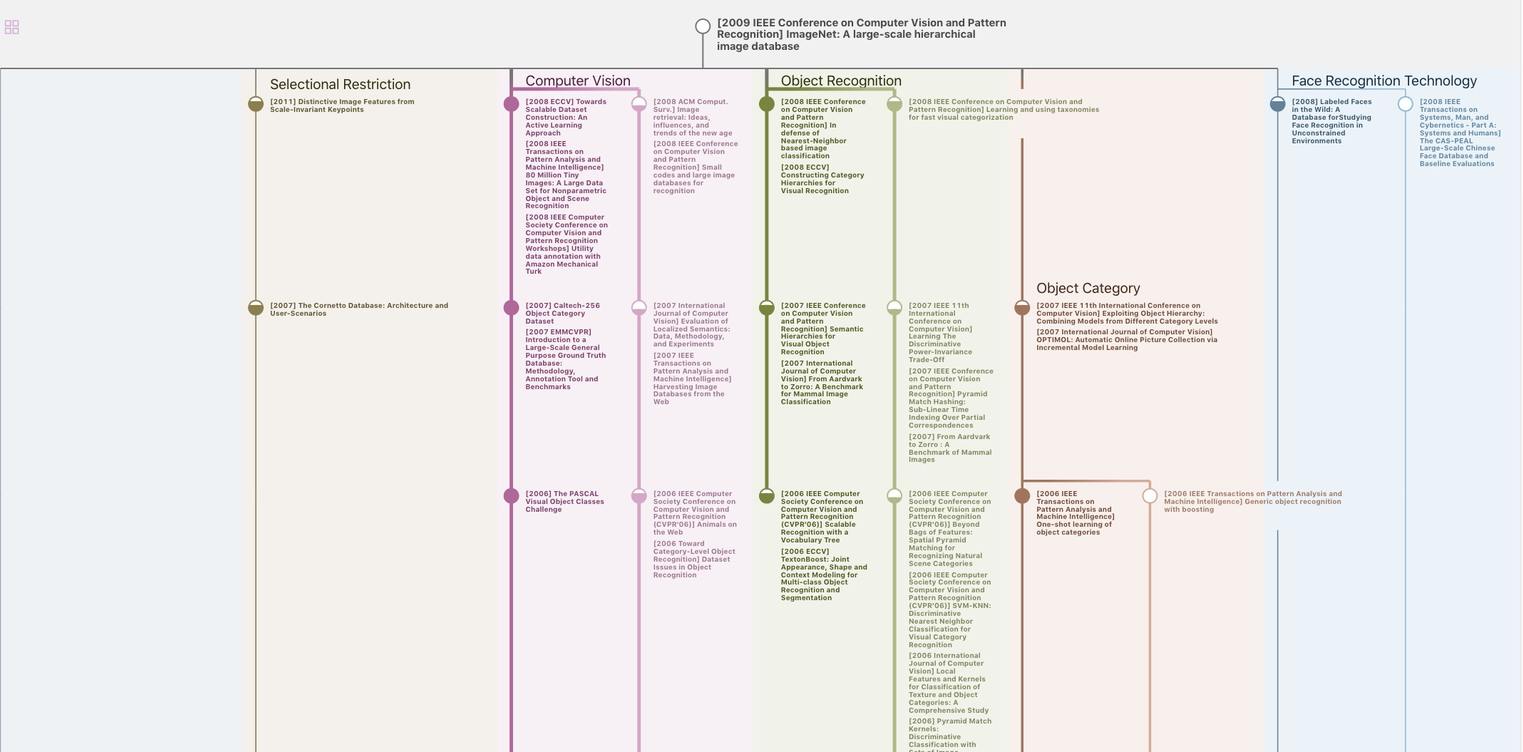
生成溯源树,研究论文发展脉络
Chat Paper
正在生成论文摘要