An Effective Semi-Supervised Approach for Liver CT Image Segmentation.
IEEE journal of biomedical and health informatics(2022)
摘要
Despite the substantial progress made by deep networks in the field of medical image segmentation, they generally require sufficient pixel-level annotated data for training. The scale of training data remains to be the main bottleneck to obtain a better deep segmentation model. Semi-supervised learning is an effective approach that alleviates the dependence on labeled data. However, most existing semi-supervised image segmentation methods usually do not generate high-quality pseudo labels to expand training dataset. In this paper, we propose a deep semi-supervised approach for liver CT image segmentation by expanding pseudo-labeling algorithm under the very low annotated-data paradigm. Specifically, the output features of labeled images from the pretrained network combine with corresponding pixel-level annotations to produce class representations according to the mean operation. Then pseudo labels of unlabeled images are generated by calculating the distances between unlabeled feature vectors and each class representation. To further improve the quality of pseudo labels, we adopt a series of operations to optimize pseudo labels. A more accurate segmentation network is obtained by expanding the training dataset and adjusting the contributions between supervised and unsupervised loss. Besides, the novel random patch based on prior locations is introduced for unlabeled images in the training procedure. Extensive experiments show our method has achieved more competitive results compared with other semi-supervised methods when fewer labeled slices of LiTS dataset are available.
更多查看译文
关键词
Image segmentation,Training,Liver,Solid modeling,Computed tomography,Medical diagnostic imaging,Bioinformatics,Semi-supervised learning,medical image segmentation,data-augmentation,liver segmentation
AI 理解论文
溯源树
样例
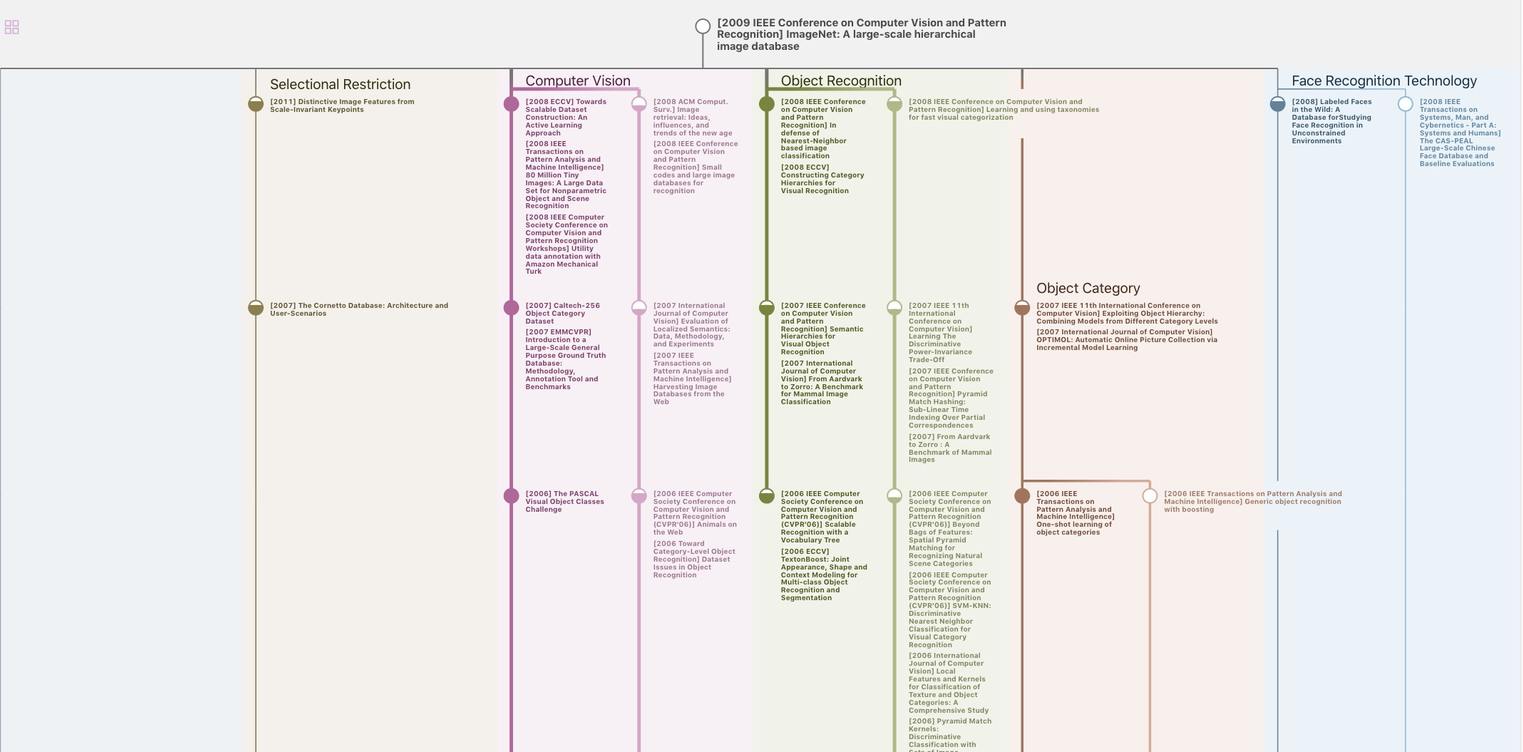
生成溯源树,研究论文发展脉络
Chat Paper
正在生成论文摘要