Characterization of Saturation and Pressure Distribution Based on Deep Learning for a Typical Carbonate Reservoir in the Middle East
Journal of petroleum science & engineering(2022)
摘要
The typical carbonate reservoir in the Middle East has huge potential, but due to low permeability and high gas-oil ratio, the characteristics of reservoir saturation and pressure distribution are not clear, which makes reservoir development very difficult. As an intelligent prediction and analysis technique, deep learning provides a novel solution to solve this problem. In this study, firstly, the actual static geological data and dynamic production data from the target reservoir were gathered to provide a data foundation for model training and analysis. And then, Random Forest algorithms, Reservoir Engineering methods, and Kriging interpolation algorithms were selected to predict and supplement the missing data of pressure and saturation field. After that, the Convolutional Long Short-term Memory (ConvLSTM) neural network algorithm was employed to build an optimal model to forecast the oil/water/gas saturation distribution and pressure distribution fields. The results showed that the optimal ConvLSTM model achieved good prediction performance. Not only the calculation efficiency, but also the prediction accuracy was higher, in which the average R2 was 0.8825 and the average accuracy was 0.865. Furthermore, we proposed five development optimizations for the remaining oil potential tapping strategies according to the prediction results. The workflow proposed in this study provides a new direction for the characterization of saturation and pressure distribution in a typical carbonate reservoir.
更多查看译文
关键词
Saturation and pressure distribution characterization,Deep learning,Neural network,Carbonate reservoir,Middle east
AI 理解论文
溯源树
样例
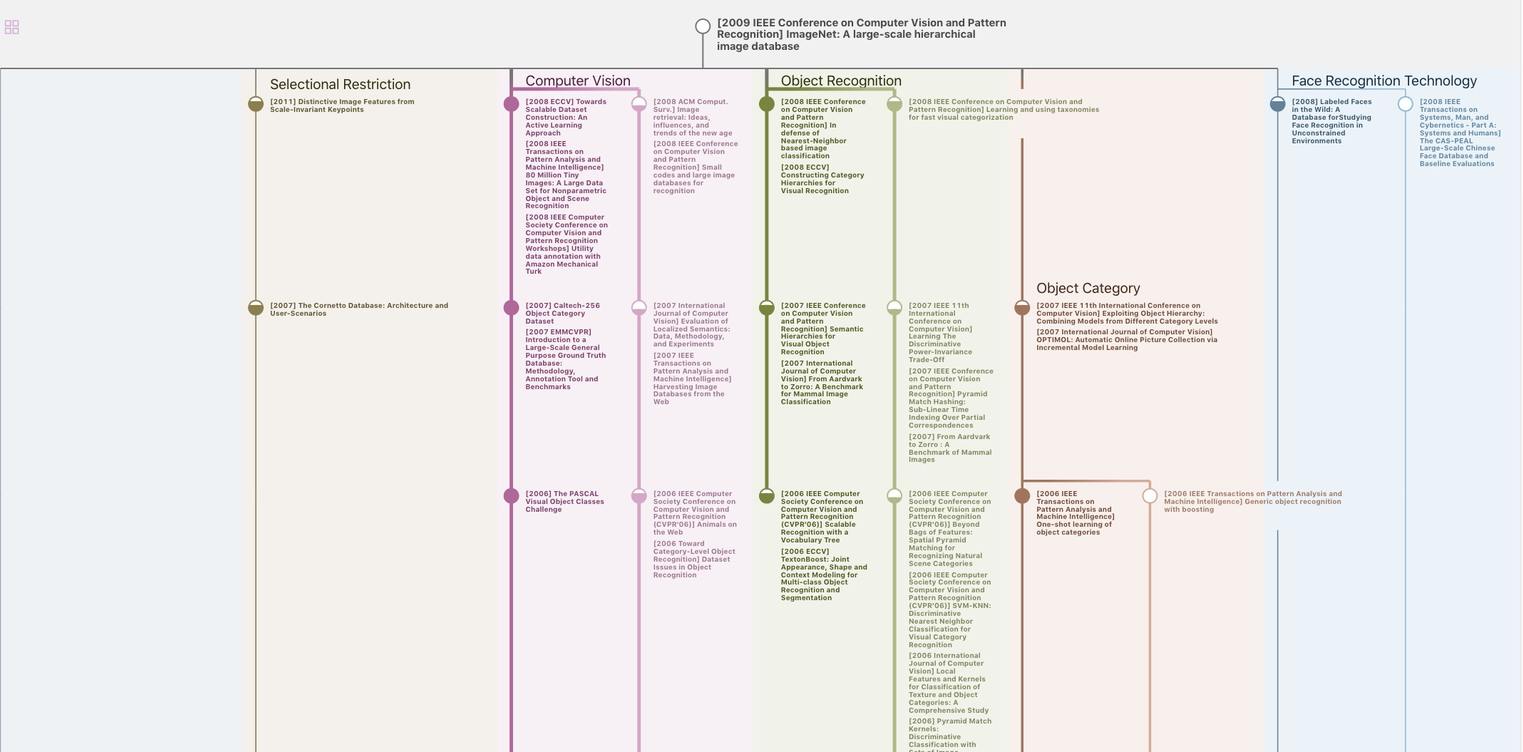
生成溯源树,研究论文发展脉络
Chat Paper
正在生成论文摘要